Multi-graph Convolutional Network for Unsupervised 3D Shape Retrieval
MM '20: The 28th ACM International Conference on Multimedia Seattle WA USA October, 2020(2020)
摘要
3D shape retrieval has attracted much research attention due to its wide applications in the fields of computer vision and multimedia. Various approaches have been proposed in recent years for learning 3D shape descriptor from different modalities. The existing works contain the following disadvantages: 1) the vast majority methods rely on the large scale of training data with clear category information; 2) many approaches focus on the fusion of multi-modal information but ignore the guidance of correlations among different modalities for shape representation learning; 3) many methods pay attention to the structural feature learning of 3D shape but ignore the guidance of structural similarity between every two shapes. To solve these problems, we propose a novel multi-graph network (MGN) for unsupervised 3D shape retrieval, which utilizes the correlations among modalities and structural similarity between two models to guide the shape representation learning process without category information. More specifically, we propose two novel loss functions: auto-correlation loss and cross-correlation loss. The auto-correlation loss utilizes information from different modalities to increase the discrimination of shape descriptor. The cross-correlation loss utilizes the structural similarity between two models to strengthen the intra-class similarity and increase the inter-class distinction. Finally, an effective similarity measurement is designed for the shape retrieval task. To validate the effectiveness of our proposed method, we conduct experiments on the ModelNet dataset. Experimental results demonstrate the effectiveness of our proposed method, and significant improvements have been achieved compared with state-of-the-art methods.
更多查看译文
关键词
3D Shape Retrieval, Multi-graph Method, Information Fusion
AI 理解论文
溯源树
样例
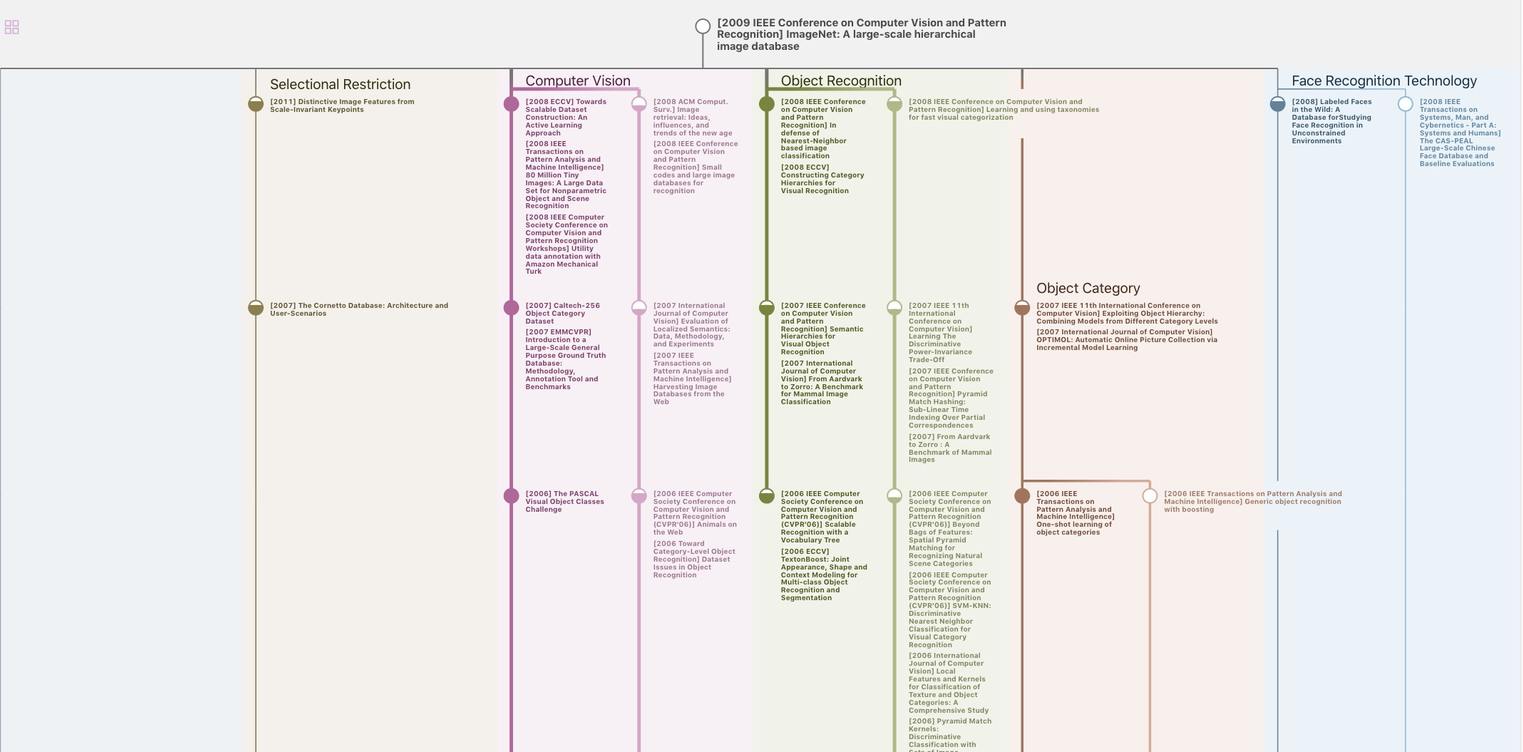
生成溯源树,研究论文发展脉络
Chat Paper
正在生成论文摘要