Efficient Wasserstein Natural Gradients for Reinforcement Learning
ICLR(2021)
摘要
A novel optimization approach is proposed for application to policy gradient methods and evolution strategies for reinforcement learning (RL). The procedure uses a computationally efficient Wasserstein natural gradient (WNG) descent that takes advantage of the geometry induced by a Wasserstein penalty to speed optimization. This method follows the recent theme in RL of including a divergence penalty in the objective to establish a trust region. Experiments on challenging tasks demonstrate improvements in both computational cost and performance over advanced baselines.
更多查看译文
关键词
efficient wasserstein natural gradients,reinforcement learning
AI 理解论文
溯源树
样例
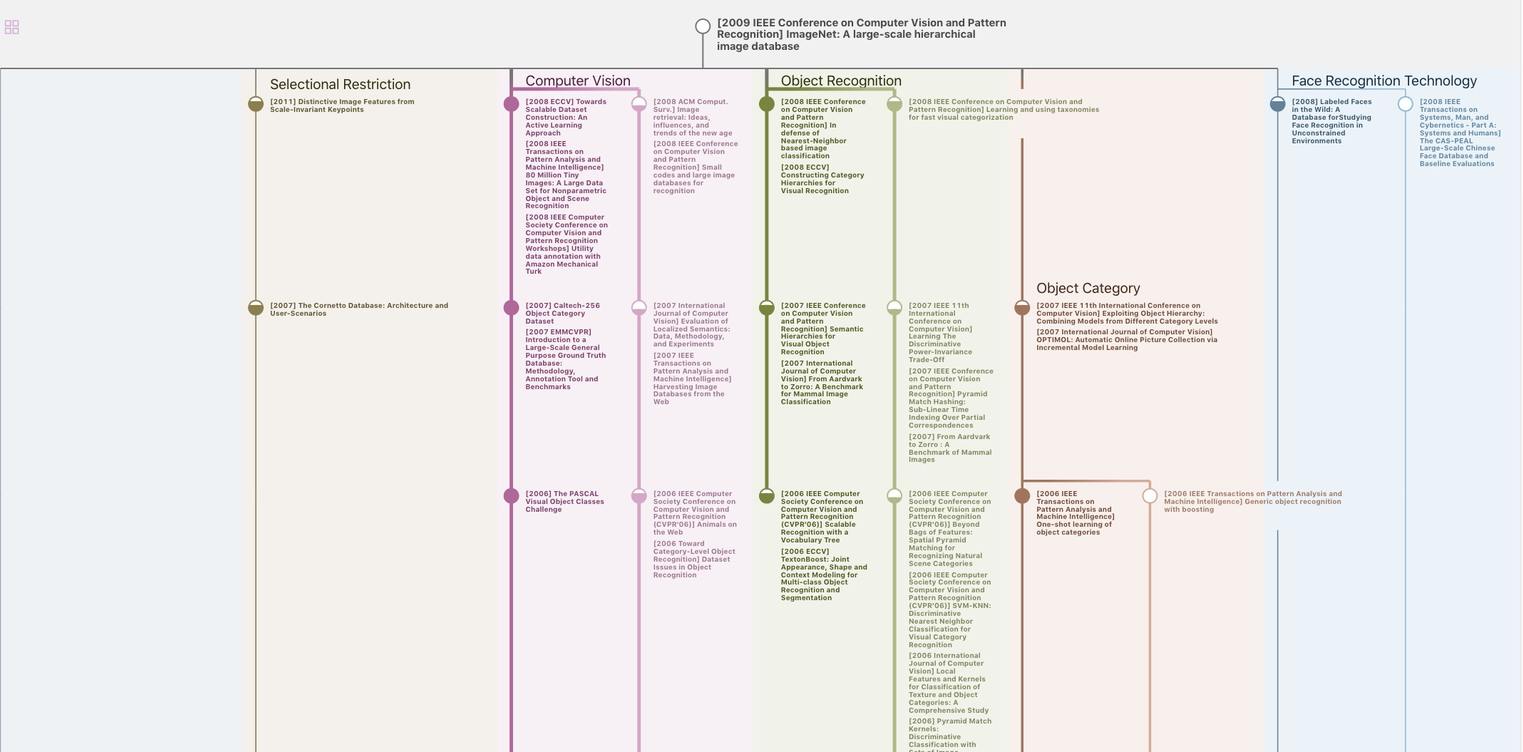
生成溯源树,研究论文发展脉络
Chat Paper
正在生成论文摘要