MS2L: Multi-Task Self-Supervised Learning for Skeleton Based Action Recognition
MM '20: The 28th ACM International Conference on Multimedia Seattle WA USA October, 2020(2020)
Abstract
In this paper, we address self-supervised representation learning from human skeletons for action recognition. Previous methods, which usually learn feature presentations from a single reconstruction task, may come across the overfitting problem, and the features are not generalizable for action recognition. Instead, we propose to integrate multiple tasks to learn more general representations in a self-supervised manner. To realize this goal, we integrate motion prediction, jigsaw puzzle recognition, and contrastive learning to learn skeleton features from different aspects. Skeleton dynamics can be modeled through motion prediction by predicting the future sequence. And temporal patterns, which are critical for action recognition, are learned through solving jigsaw puzzles. We further regularize the feature space by contrastive learning. Besides, we explore different training strategies to utilize the knowledge from self-supervised tasks for action recognition. We evaluate our multi-task self-supervised learning approach with action classifiers trained under different configurations, including unsupervised, semi-supervised and fully-supervised settings. Our experiments on the NW-UCLA, NTU RGB+D, and PKUMMD datasets show remarkable performance for action recognition, demonstrating the superiority of our method in learning more discriminative and general features. Our project website is available at https: //langlandslin.github.io/projects/MSL/.
MoreTranslated text
Key words
Action recognition,multi-task,self-supervised learning
AI Read Science
Must-Reading Tree
Example
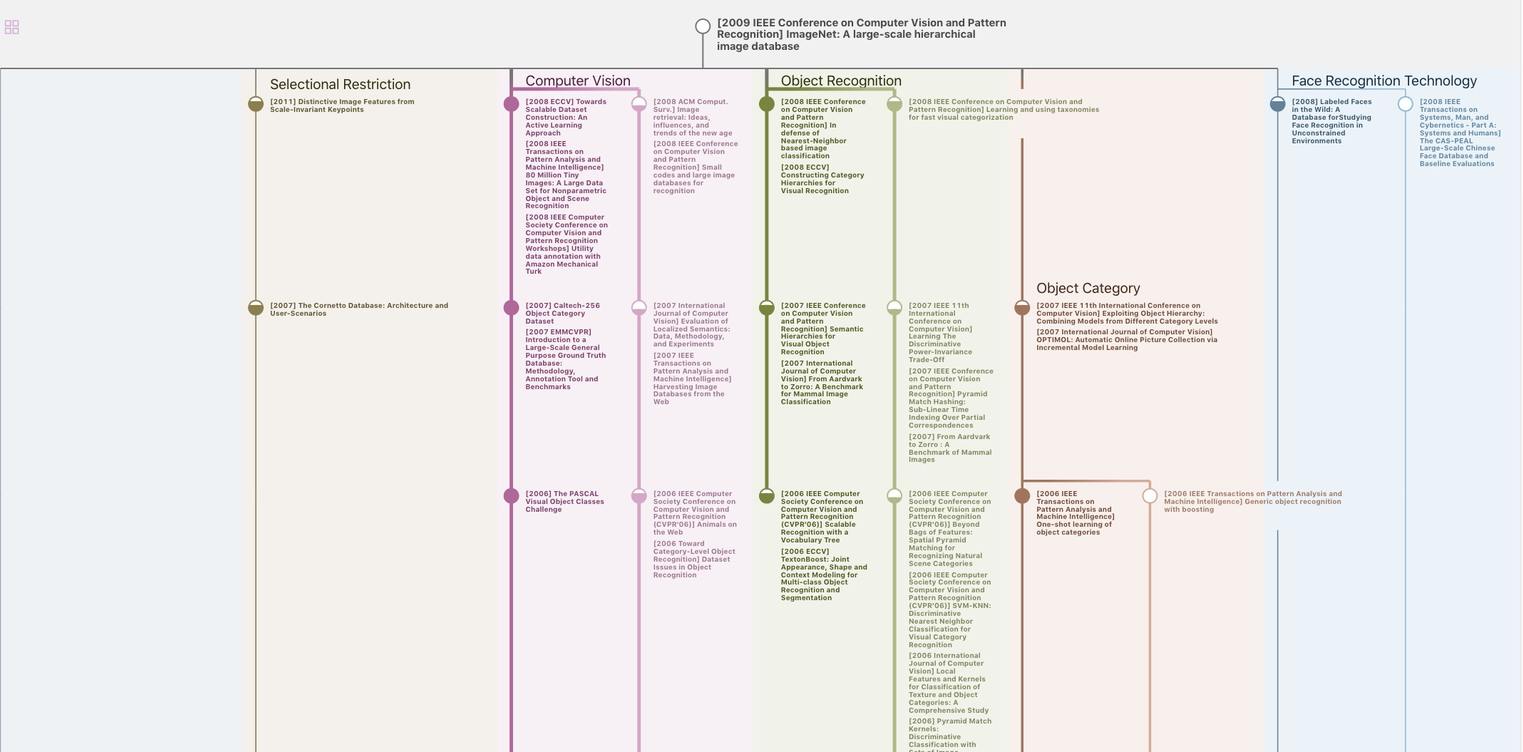
Generate MRT to find the research sequence of this paper
Chat Paper
Summary is being generated by the instructions you defined