FaiR-N: Fair and Robust Neural Networks for Structured Data
AIES '21: PROCEEDINGS OF THE 2021 AAAI/ACM CONFERENCE ON AI, ETHICS, AND SOCIETY(2021)
摘要
Fairness and robustness in machine learning are crucial when individuals are subject to automated decisions made by models in high-stake domains. To promote ethical artificial intelligence, fairness metrics that rely on comparing model error rates across sub-populations have been widely investigated for the detection and mitigation of bias. However, fairness measures that rely on comparing the ability to achieve recourse have been relatively unexplored. In this paper, we present a novel formulation for training neural networks that considers the distance of data observations to the decision boundary such that the new objective: (1) reduces the disparity in the average ability of recourse between individuals in each protected group, and (2) increases the average distance of data points to the boundary to promote adversarial robustness. We demonstrate that models trained with this new objective are more fair and adversarially robust neural networks, with similar accuracies, when compared to models without it. We also investigate a trade-off between the recourse-based fairness and robustness objectives. Moreover, we qualitatively motivate and empirically show that reducing recourse disparity across protected groups also improves fairness measures that rely on error rates. To the best of our knowledge, this is the first time that recourse disparity across groups are considered to train fairer neural networks.
更多查看译文
关键词
Fairness, Robustness, Neural Networks, Ethical Artificial Intelligence
AI 理解论文
溯源树
样例
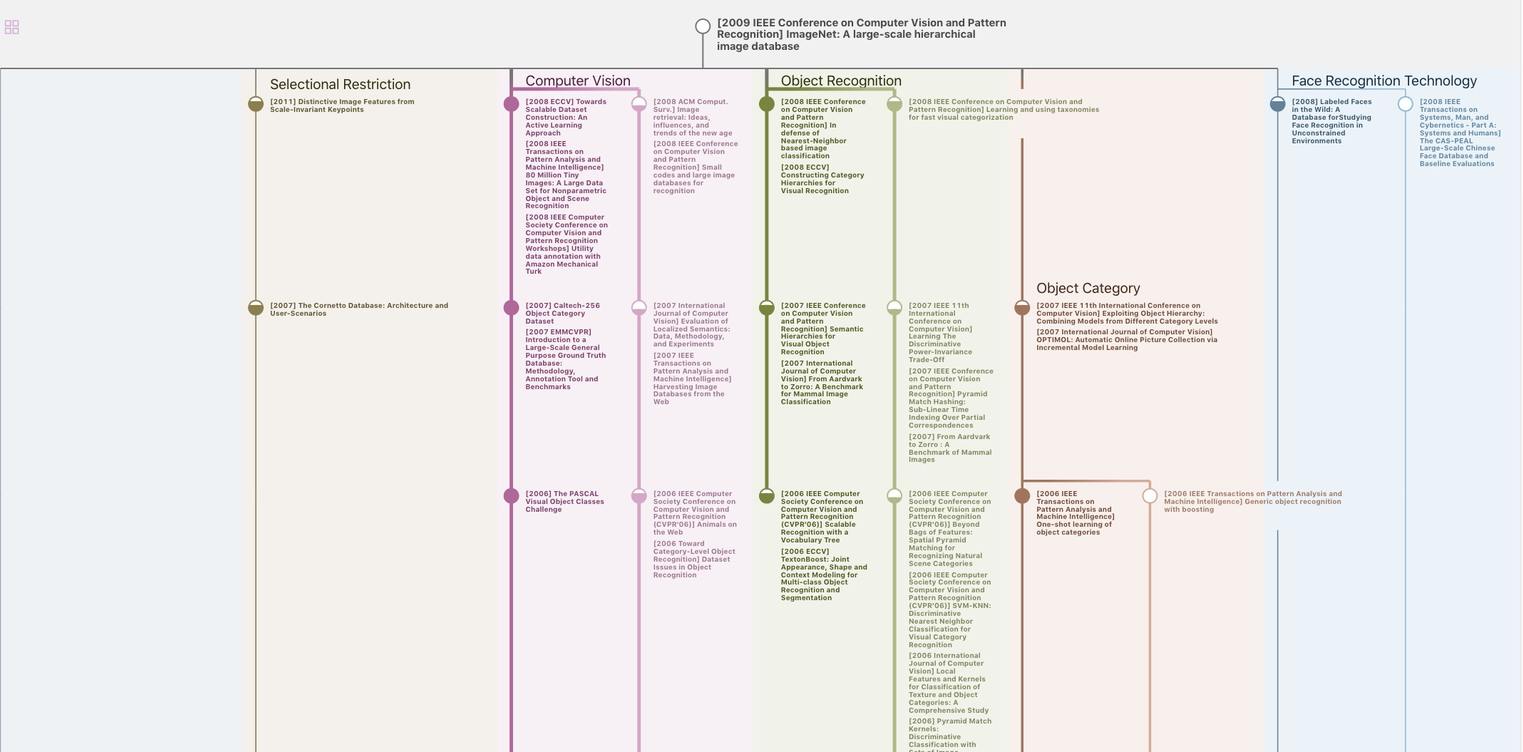
生成溯源树,研究论文发展脉络
Chat Paper
正在生成论文摘要