Towards Understanding Pixel Vulnerability under Adversarial Attacks for Images
arxiv(2020)
摘要
Deep neural network image classifiers are reported to be susceptible to adversarial evasion attacks, which use carefully crafted images created to mislead a classifier. Recently, various kinds of adversarial attack methods have been proposed, most of which focus on adding small perturbations to all of the pixels of a real image. We find that a considerable amount of the perturbations on an image generated by some widely-used attacks may contribute little in attacking a classifier. However, they usually result in a more easily detectable adversarial image by both humans and adversarial attack detection algorithms. Therefore, it is important to impose the perturbations on the most vulnerable pixels of an image that can change the predictions of classifiers more readily. With the pixel vulnerability, given an existing attack, we can make its adversarial images more realistic and less detectable with fewer perturbations but keep its attack performance the same. Moreover, the discovered vulnerability assists to get a better understanding of the weakness of deep classifiers. Derived from the information-theoretic perspective, we propose a probabilistic approach for automatically finding the pixel vulnerability of an image, which is compatible with and improves over many existing adversarial attacks.
更多查看译文
关键词
understanding pixel vulnerability,adversarial attacks,images
AI 理解论文
溯源树
样例
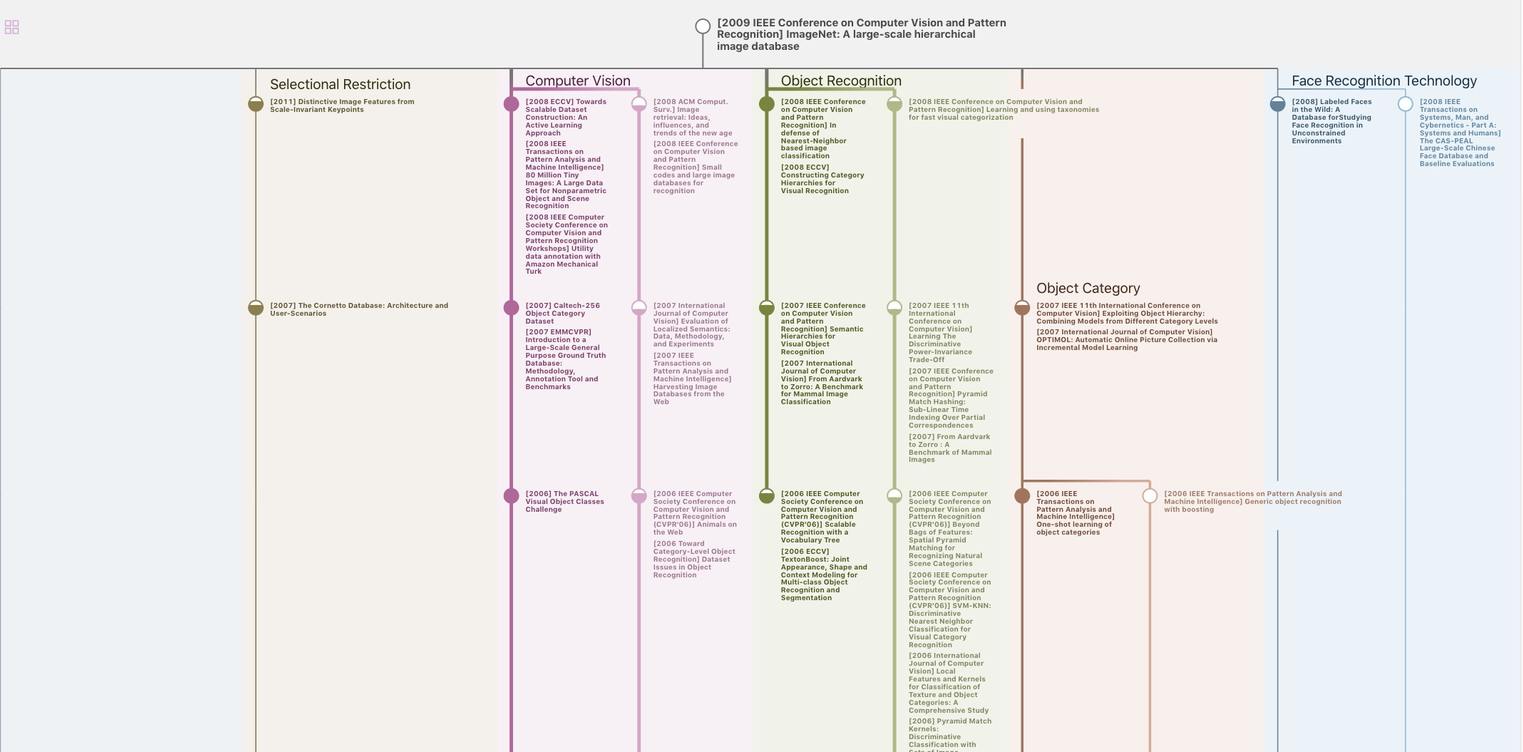
生成溯源树,研究论文发展脉络
Chat Paper
正在生成论文摘要