IExpressNet: Facial Expression Recognition with Incremental Classes
MM '20: The 28th ACM International Conference on Multimedia Seattle WA USA October, 2020(2020)
摘要
Existing methods on facial expression recognition (FER) are mainly trained in the setting when all expression classes are fixed in advance. However, in real applications, expression classes are becoming increasingly fine-grained and incremental. To deal with sequential expression classes, we can fine-tune or re-train these models, but this often results in poor performance or large computing resources consumption. To address these problems, we develop an Incremental Facial Expression Recognition Network (IExpressNet), which can learn a competitive multi-class classifier at any time with a lower requirement of computing resources. Specifically, IExpressNet consists of two novel components. First, we construct an exemplar set by dynamically selecting representative samples from old expression classes. Then, the exemplar set and new expression classes samples constitute the training set. Second, we design a novel center-expression-distilled loss. As for facial expression in the wild, center-expression-distilled loss enhances the discriminative power of the deeply learned features and prevents catastrophic forgetting. Extensive experiments are conducted on two large-scale FER datasets in the wild, RAF-DB and AffectNet. The results demonstrate the superiority of the proposed method as compared to state-of-the-art incremental learning approaches.
更多查看译文
关键词
Facial expression recognition, class incremental
AI 理解论文
溯源树
样例
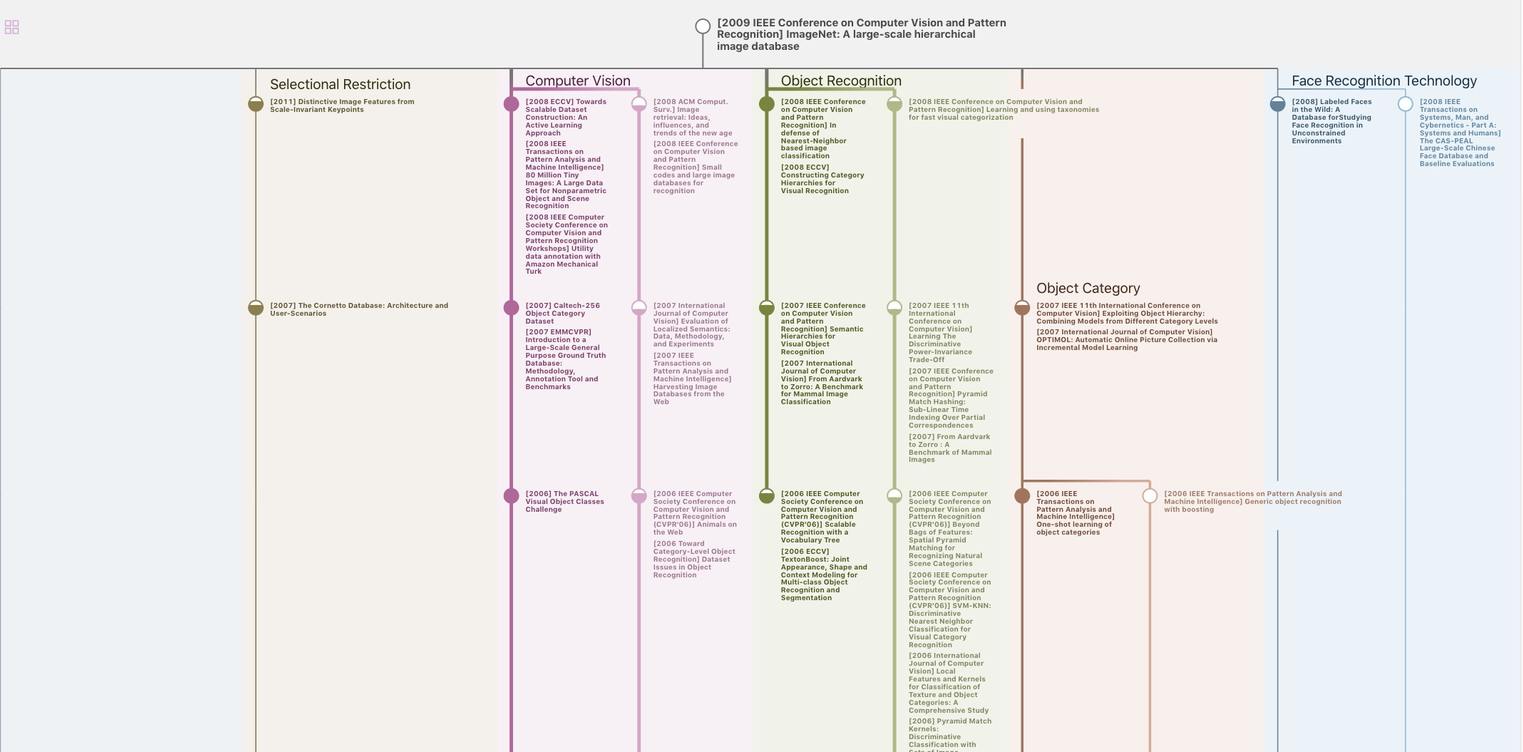
生成溯源树,研究论文发展脉络
Chat Paper
正在生成论文摘要