Pose-native Network Architecture Search for Multi-person Human Pose Estimation
MM '20: The 28th ACM International Conference on Multimedia Seattle WA USA October, 2020(2020)
摘要
Multi-person pose estimation has achieved great progress in recent years, even though, the precise prediction for occluded and invisible hard keypoints remains challenging. Most of the human pose estimation networks are equipped with an image classification-based pose encoder for feature extraction and a handcrafted pose decoder for high-resolution representations. However, the pose encoder might be sub-optimal because of the gap between image classification and pose estimation. The widely used multi-scale feature fusion in pose decoder is still coarse and cannot provide sufficient high-resolution details for hard keypoints. Neural Architecture Search (NAS) has shown great potential in many visual tasks to automatically search efficient networks. In this work, we present the Pose-native Network Architecture Search (PoseNAS) to simultaneously design a better pose encoder and pose decoder for pose estimation. Specifically, we directly search a data-oriented pose encoder with stacked searchable cells, which can provide an optimum feature extractor for the pose specific task. In the pose decoder, we exploit scale-adaptive fusion cells to promote rich information exchange across the multi-scale feature maps. Meanwhile, the pose decoder adopts a Fusion-and-Enhancement manner to progressively boost the high-resolution representations that are non-trivial for the precious prediction of hard keypoints. With the exquisitely designed search space and search strategy, PoseNAS can simultaneously search all modules in an end-to-end manner. PoseNAS achieves state-of-the-art performance on three public datasets, MPII, COCO, and PoseTrack, with small-scale parameters compared with the existing methods. Our best model obtains 76.7% mAP and 75.9% mAP on the COCO validation set and test set with only 33.6M parameters. Code and implementation are available at https://github.com/for-code0216/PoseNAS.
更多查看译文
AI 理解论文
溯源树
样例
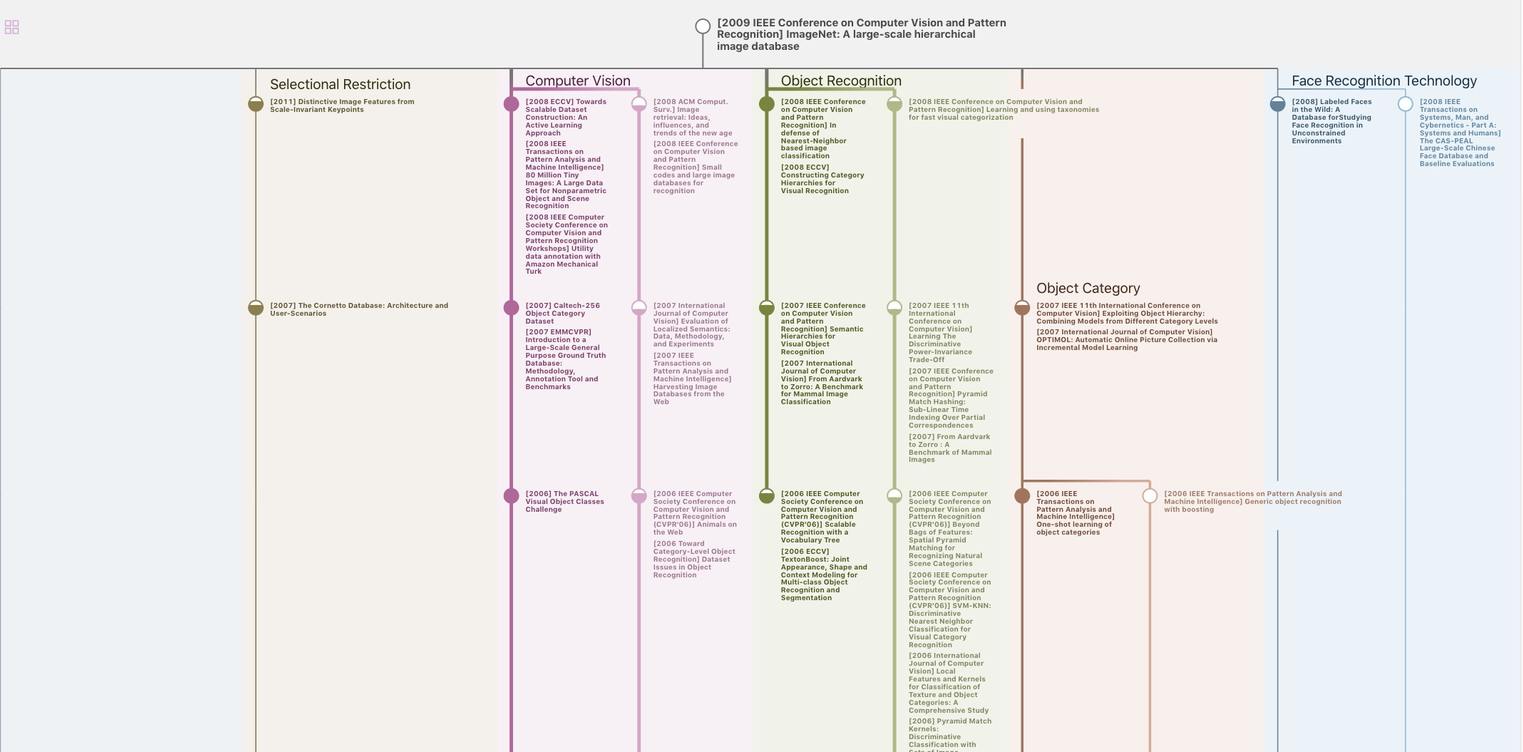
生成溯源树,研究论文发展脉络
Chat Paper
正在生成论文摘要