A Decomposition-Based Evolutionary Algorithm With Correlative Selection Mechanism For Many-Objective Optimization
EVOLUTIONARY COMPUTATION(2021)
摘要
Decomposition-based evolutionary algorithms have been quite successful in dealing with multiobjective optimization problems. Recently, more and more researchers attempt to apply the decomposition approach to solve many-objective optimization problems. A many-objective evolutionary algorithm based on decomposition with correlative selection mechanism (MOEA/D-CSM) is also proposed to solve many-objective optimization problems in this article. Since MOEA/D-SCM is based on a decomposition approach which adopts penalty boundary intersection (PBI), a set of reference points must be generated in advance. Thus, a new concept related to the set of reference points is introduced first, namely, the correlation between an individual and a reference point. Thereafter, a new selection mechanism based on the correlation is designed and called correlative selection mechanism. The correlative selection mechanism finds its correlative individuals for each reference point as soon as possible so that the diversity among population members is maintained. However, when a reference point has two or more correlative individuals, the worse correlative individuals may be removed from a population so that the solutions can be ensured to move toward the Pareto-optimal front. In a comprehensive experimental study, we apply-MOEA/D-CSM to a number of many-objective test problems with 3 to 15 objectives and make a comparison with three state-of-the-art many-objective evolutionary algorithms, namely, NSGA-III, MOEA/D, and RVEA. Experimental results show that the proposed MOEA/D-CSM can produce competitive results on most of the problems considered in this study.
更多查看译文
关键词
Many-objective optimization, evolutionary computation, decomposition, correlative selection mechanism
AI 理解论文
溯源树
样例
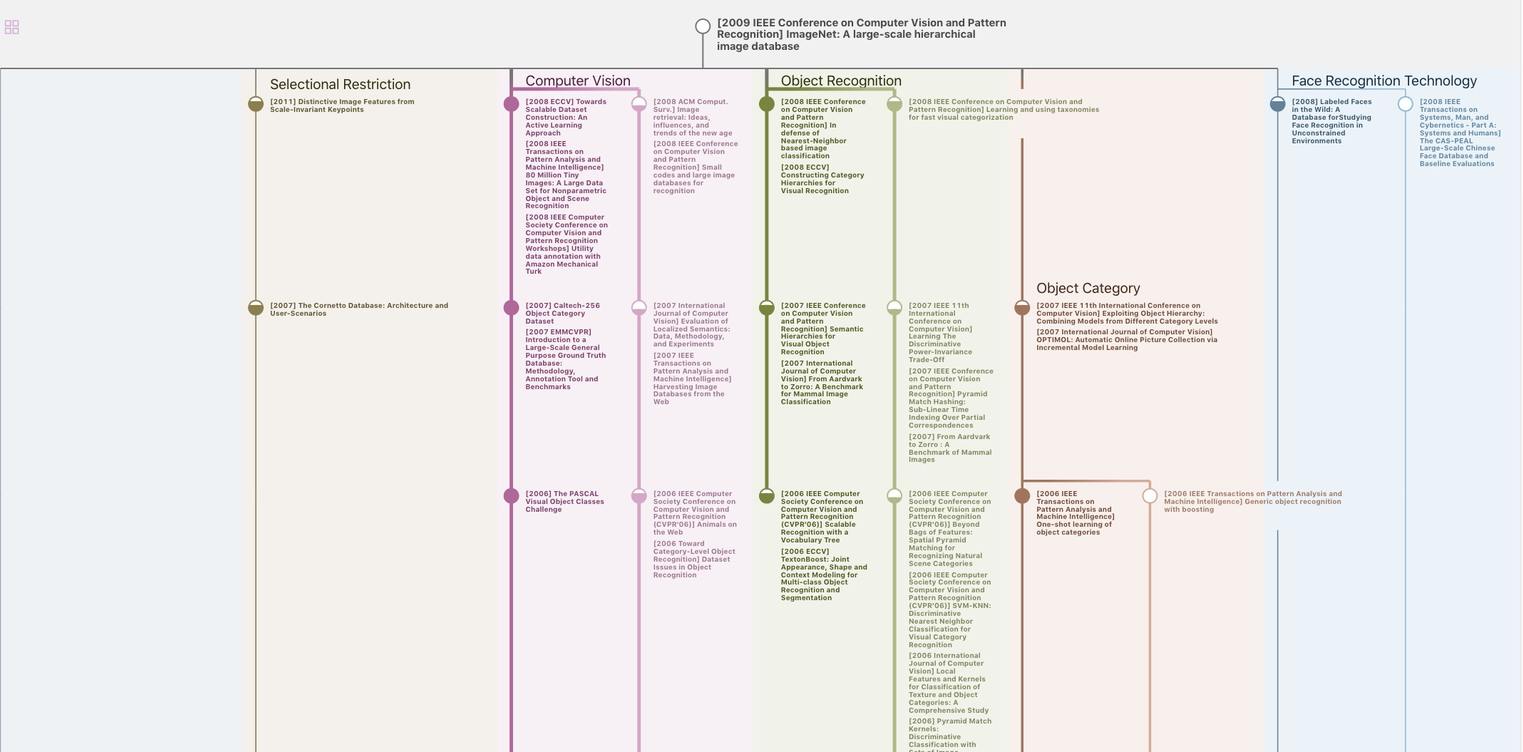
生成溯源树,研究论文发展脉络
Chat Paper
正在生成论文摘要