Connected Vehicle Based Distributed Meta-Learning for Online Adaptive Engine/Powertrain Fuel Consumption Modeling
IEEE Transactions on Vehicular Technology(2020)
摘要
A microscopic fuel consumption model with respect to comprehensive fuel consumption key impact factors that can reflect dynamic engine operations and various real-world driving conditions is essential for fuel efficient model-based vehicle and powertrain control (MB-VPC). Existing microscopic fuel consumption models are mostly steady state models with limited vehicle parameters as dynamic variables. Data-driven solutions can make the model comprehensive by introducing broad impact factors. However, without the required accessible online computational capability and fast model adaptation mechanisms, those data-driven solutions cannot quickly adapt to unseen driving conditions and engine condition changes based on real-world vehicle data to support MB-VPC. In this paper, a connected vehicle-based data mining (CV-DM) framework is proposed to achieve online adaptive dynamic fuel consumption modeling through knowledge sharing over CVs and the CV remote data center. Based on the CV-DM framework, CV-supported Distributed Meta-regression (CV-DMR) algorithms are developed to realize a fast few-shot adaptation with limited training data. Extensive proof-of-concept experiments are conducted with steady-state and transient vehicle engine data. Compared to the baseline physical model and the existing non-adaptive grey-box model, prediction accuracy is improved by 47%–85% and 38%–80% respectively with only limited training data needed for the subject vehicle. Accordingly, a fuel savings of up to 9.4% can be achieved owing to the improvement of prediction accuracy.
更多查看译文
关键词
Adaptation models,Fuels,Data models,Computational modeling,Vehicle dynamics,Engines,Connected vehicles
AI 理解论文
溯源树
样例
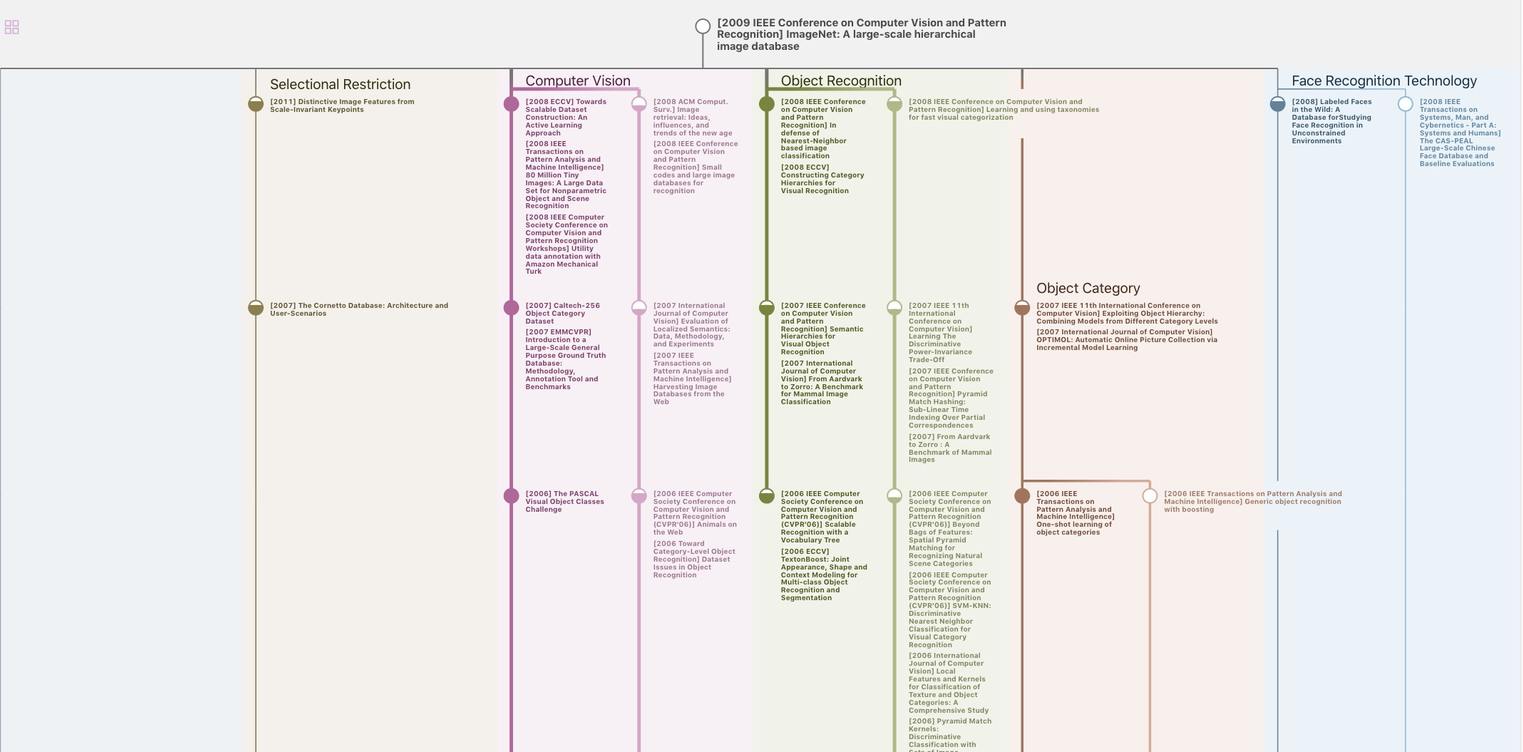
生成溯源树,研究论文发展脉络
Chat Paper
正在生成论文摘要