Using Quasispecies Patterns Of Hepatitis B Virus To Predict Hepatocellular Carcinoma With Deep Sequencing And Machine Learning
JOURNAL OF INFECTIOUS DISEASES(2021)
摘要
Background. Hepatitis B virus (HBV) infection is one of the main leading causes of hepatocellular carcinoma (HCC) worldwide. However, it remains uncertain how the reverse-transcriptase (rt) gene contributes to HCC progression.Methods. We enrolled a total of 307 patients with chronic hepatitis B (CHB) and 237 with HBV-related HCC from 13 medical centers. Sequence features comprised multidimensional attributes of rt nucleic acid and rt/s amino acid sequences. Machine-learning models were used to establish HCC predictive algorithms. Model performances were tested in the training and independent validation cohorts using receiver operating characteristic curves and calibration plots.Results. A random forest (RF) model based on combined metrics (10 features) demonstrated the best predictive performances in both cross and independent validation (AUC, 0.96; accuracy, 0.90), irrespective of HBV genotypes and sequencing depth. Moreover, HCC risk scores for individuals obtained from the RF model (AUC, 0.966; 95% confidence interval, .922-.989) outperformed alpha-fetoprotein (0.713; .632-.784) in distinguishing between patients with HCC and those with CHB.Conclusions. Our study provides evidence for the first time that HBV rt sequences contain vital HBV quasispecies features in predicting HCC. Integrating deep sequencing with feature extraction and machine-learning models benefits the longitudinal surveillance of CHB and HCC risk assessment.
更多查看译文
关键词
hepatocellular carcinoma (HCC), hepatitis B virus (HBV), next-generation sequencing (NGS), machine learning (ML), algorithm
AI 理解论文
溯源树
样例
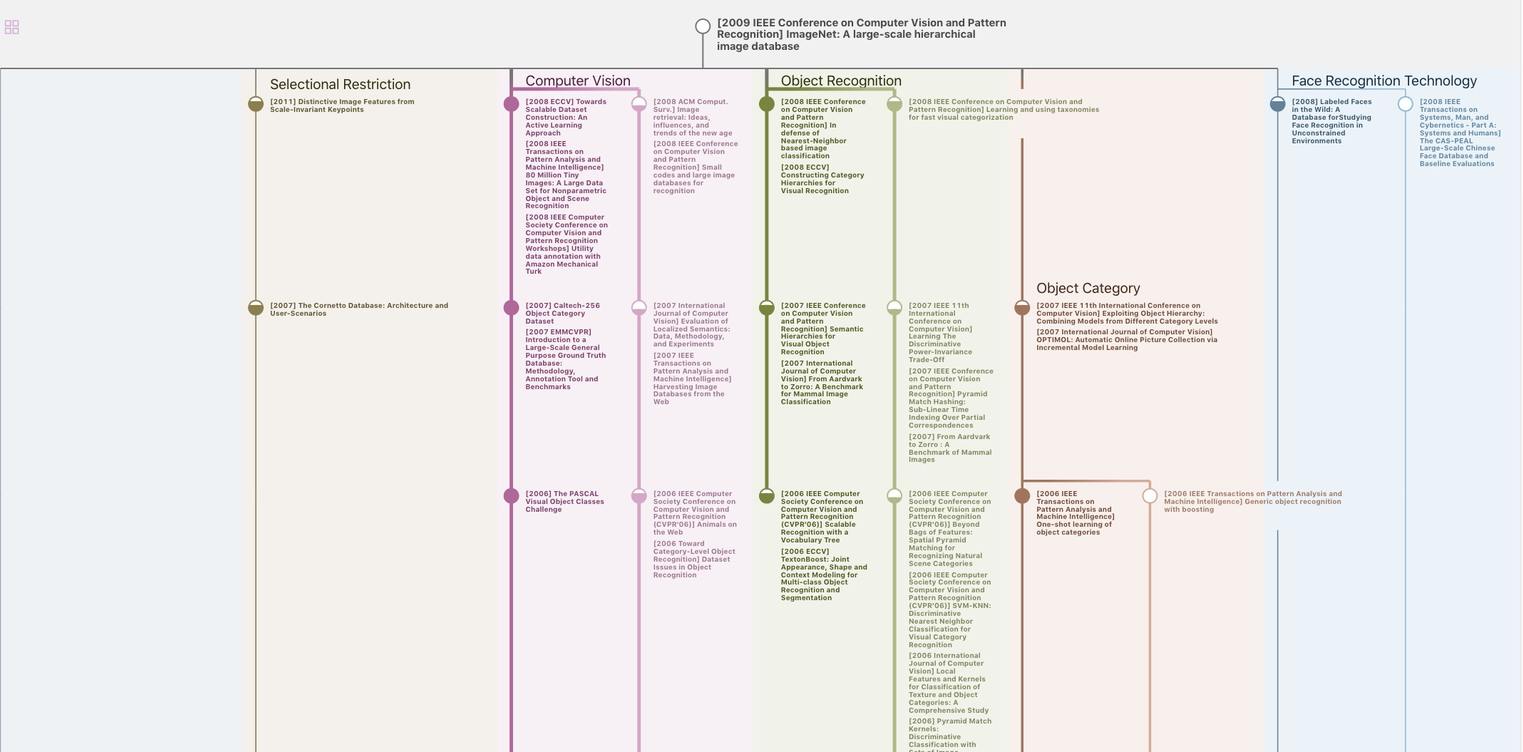
生成溯源树,研究论文发展脉络
Chat Paper
正在生成论文摘要