MulDE: Multi-teacher Knowledge Distillation for Low-dimensional Knowledge Graph Embeddings
International World Wide Web Conference(2021)
摘要
ABSTRACT Link prediction based on knowledge graph embeddings (KGE) aims to predict new triples to automatically construct knowledge graphs (KGs). However, recent KGE models achieve performance improvements by excessively increasing the embedding dimensions, which may cause enormous training costs and require more storage space. In this paper, instead of training high-dimensional models, we propose MulDE, a novel knowledge distillation framework, which includes multiple low-dimensional hyperbolic KGE models as teachers and two student components, namely Junior and Senior. Under a novel iterative distillation strategy, the Junior component, a low-dimensional KGE model, asks teachers actively based on its preliminary prediction results, and the Senior component integrates teachers’ knowledge adaptively to train the Junior component based on two mechanisms: relation-specific scaling and contrast attention. The experimental results show that MulDE can effectively improve the performance and training speed of low-dimensional KGE models. The distilled 32-dimensional model is competitive compared to the state-of-the-art high-dimensional methods on several widely-used datasets.
更多查看译文
关键词
Knowledge graph embeddings, link prediction, knowledge distillation, knowledge graph
AI 理解论文
溯源树
样例
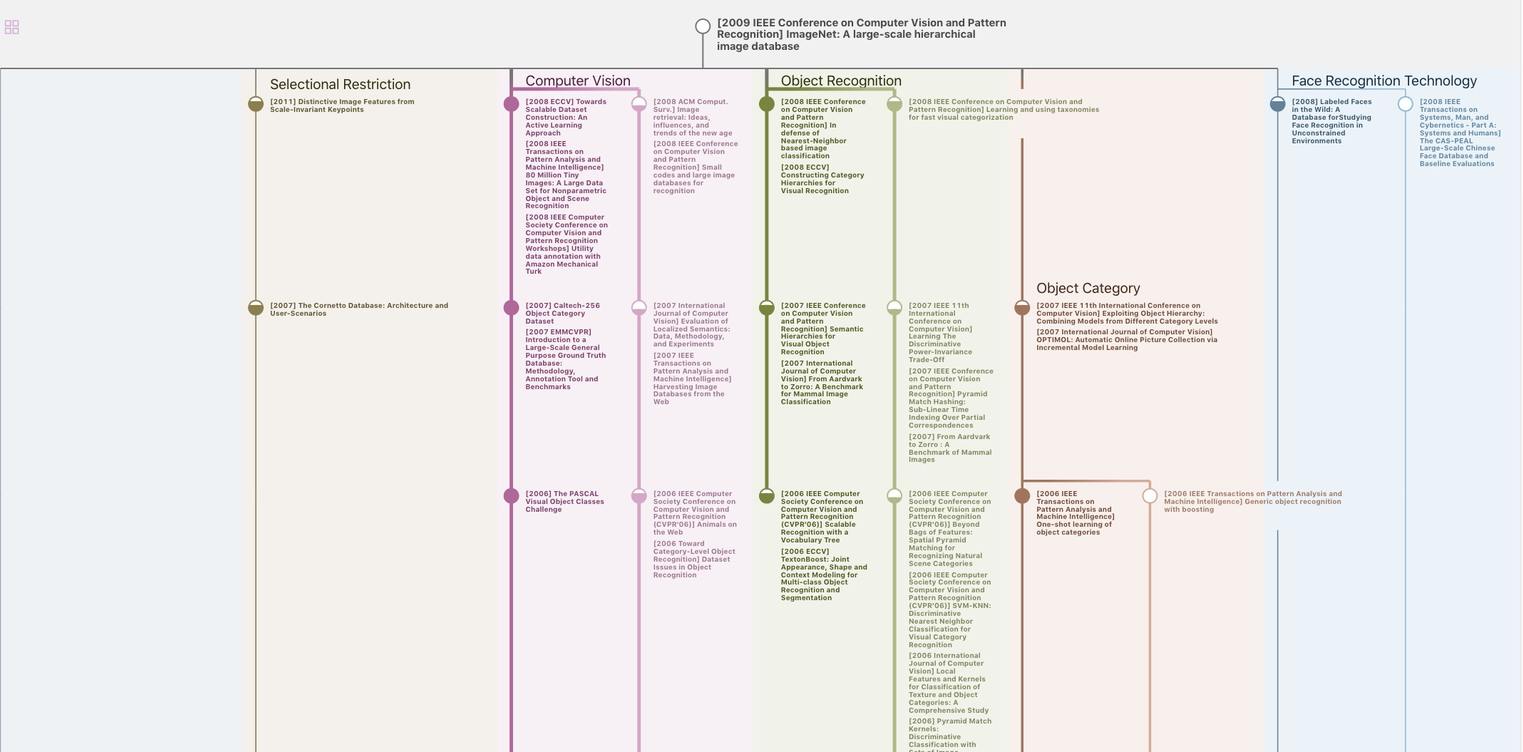
生成溯源树,研究论文发展脉络
Chat Paper
正在生成论文摘要