A data partition strategy for dimension reduction
AIMS MATHEMATICS(2020)
摘要
Based on the idea that different data contributes differently to dimension reduction, we propose a weighted affinity propagation strategy to partition the data into representative data and common data. The representative data have dominant features while the common data have less importance. In the dimension reduction, the sparse relationship and geodesic distances between pairs of representative data are preserved, and the common data are recovered through a linear combination of the adjacent representative data in the projection space. Experiments on benchmark datasets demonstrate the competitive performance of the proposed method with other methods.
更多查看译文
关键词
dimension reduction,affinity propagation,geodesic distance,linear combination
AI 理解论文
溯源树
样例
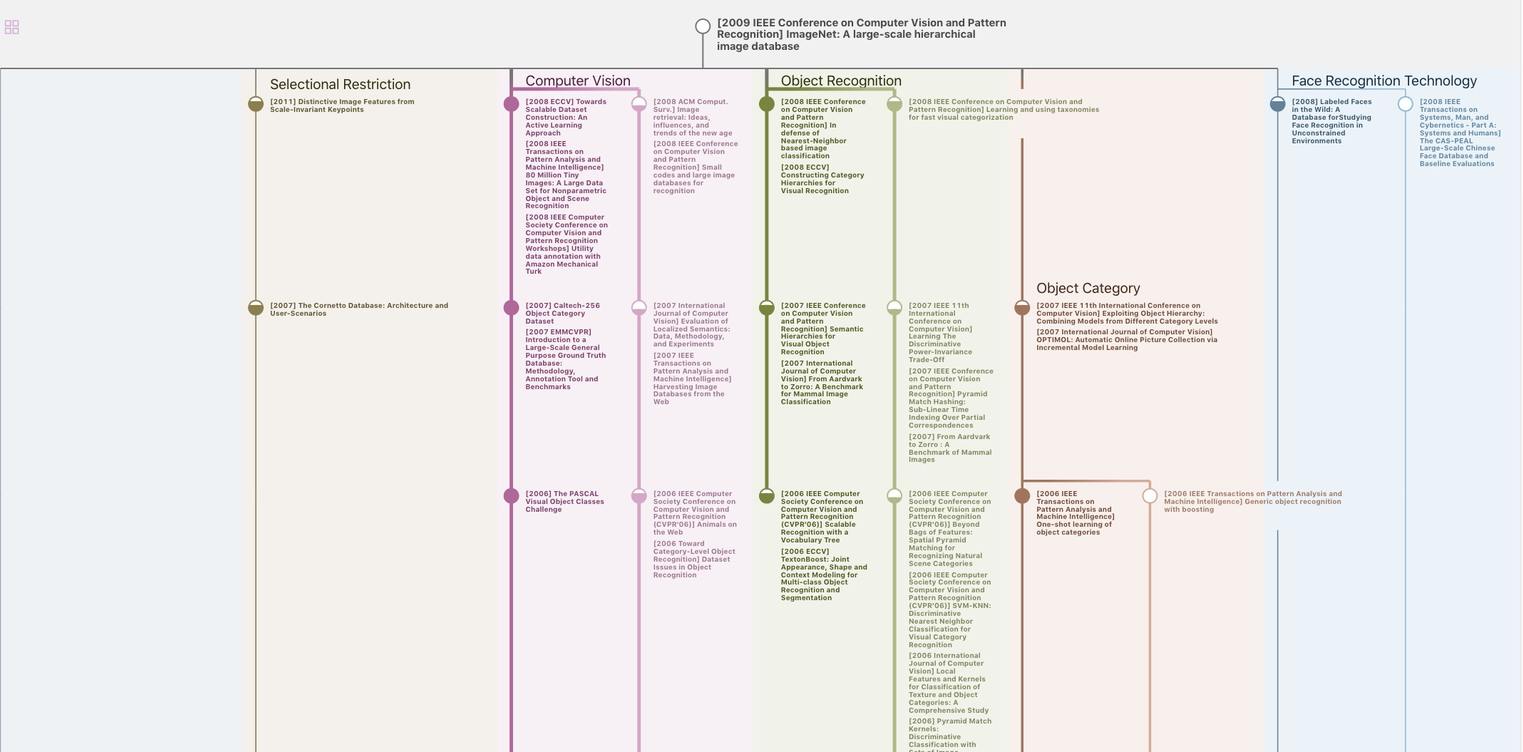
生成溯源树,研究论文发展脉络
Chat Paper
正在生成论文摘要