Efficient Imitation Learning with Local Trajectory Optimization
ICML 2020 Workshop on Inductive Biases, Invariances and Generalization in RL(2020)
摘要
Imitation learning is a powerful approach to optimize sequential decision making policies from demonstrations. Most strategies in imitation learning rely on per-step supervision from precollected demonstrations as in behavioral cloning (Pomerleau, 1989) or from interactive expert policy queries such as DAgger (Ross et al., 2011). In this work, we present a unified view of behavioral cloning and DAgger through the lens of local trajectory optimization, which offers a means of interpolating between them. We provide theoretical justification for the proposed local trajectory optimization algorithm and show empirically that our method, POLISH (Policy Optimization by Local Improvement through Search), is much faster than methods that plan globally, speeding up training by a factor of up to 14 in wall clock time. Furthermore, the resulting policy outperforms strong baselines in both reinforcement learning and imitation learning.
更多查看译文
AI 理解论文
溯源树
样例
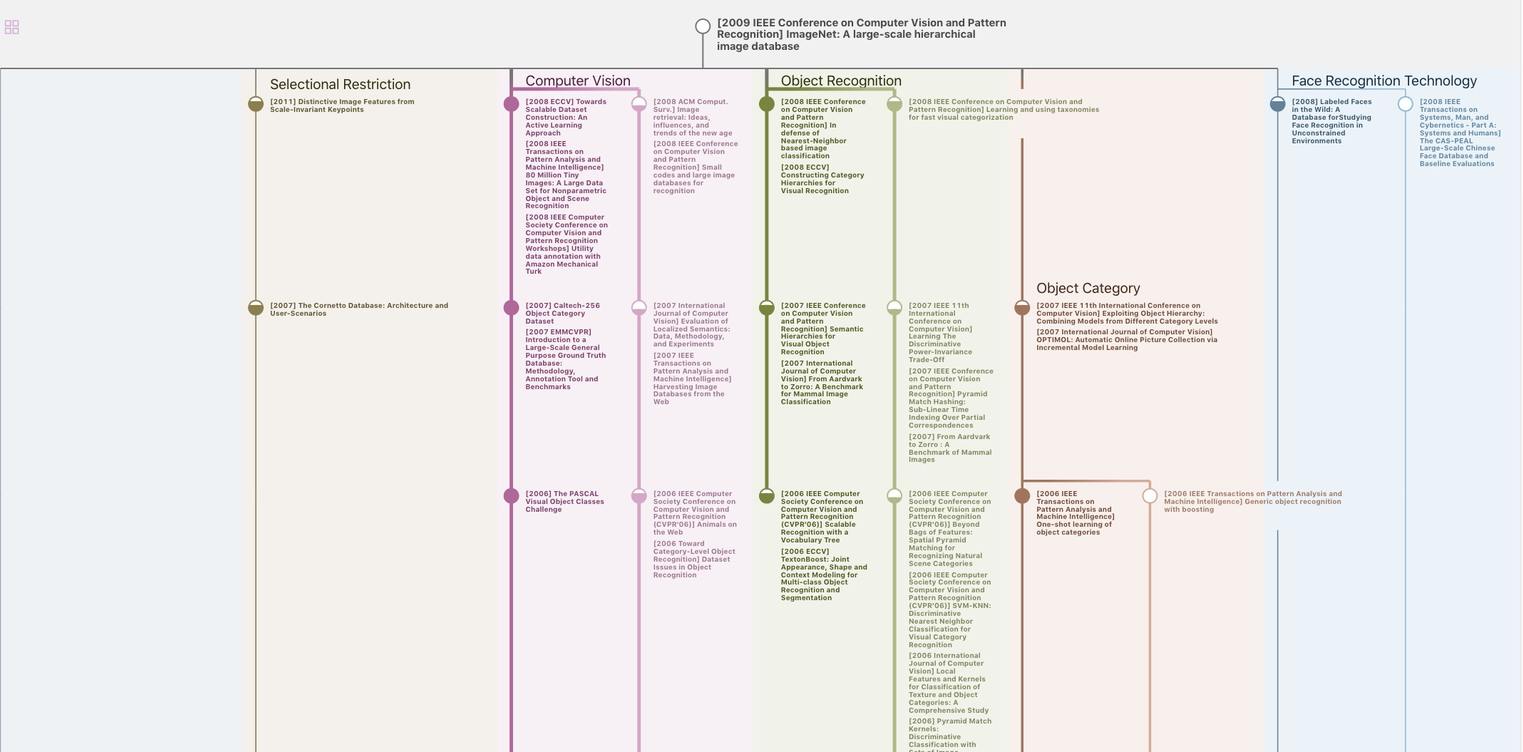
生成溯源树,研究论文发展脉络
Chat Paper
正在生成论文摘要