Statistical Inference For Online Decision Making Via Stochastic Gradient Descent
JOURNAL OF THE AMERICAN STATISTICAL ASSOCIATION(2021)
摘要
Online decision making aims to learn the optimal decision rule by making personalized decisions and updating the decision rule recursively. It has become easier than before with the help of big data, but new challenges also come along. Since the decision rule should be updated once per step, an offline update which uses all the historical data is inefficient in computation and storage. To this end, we propose a completely online algorithm that can make decisions and update the decision rule online via stochastic gradient descent. It is not only efficient but also supports all kinds of parametric reward models. Focusing on the statistical inference of online decision making, we establish the asymptotic normality of the parameter estimator produced by our algorithm and the online inverse probability weighted value estimator we used to estimate the optimal value. Online plugin estimators for the variance of the parameter and value estimators are also provided and shown to be consistent, so that interval estimation and hypothesis test are possible using our method. The proposed algorithm and theoretical results are tested by simulations and a real data application to news article recommendation.
更多查看译文
关键词
Big data, Epsilon-greedy, Inverse probability weighted estimation, Online decision making, Optimal decision rule, Value function
AI 理解论文
溯源树
样例
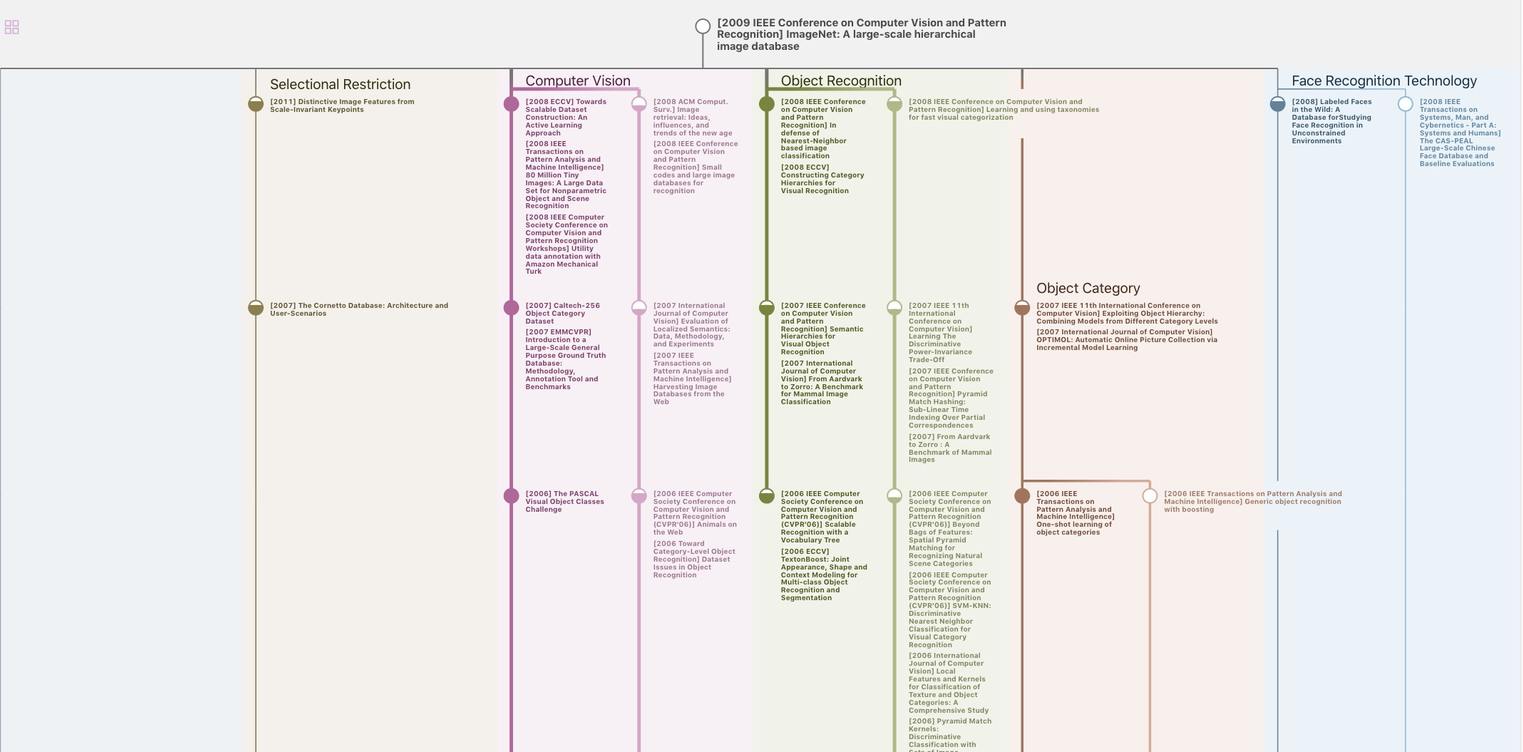
生成溯源树,研究论文发展脉络
Chat Paper
正在生成论文摘要