Spherical Knowledge Distillation
arxiv(2020)
摘要
Knowledge distillation aims at obtaining a small but effective deep model by transferring knowledge from a much larger one. The previous approaches try to reach this goal by simply "logit-supervised" information transferring between the teacher and student, which somehow can be subsequently decomposed as the transfer of normalized logits and $l^2$ norm. We argue that the norm of logits is actually interference, which damages the efficiency in the transfer process. To address this problem, we propose Spherical Knowledge Distillation (SKD). Specifically, we project the teacher and the student's logits into a unit sphere, and then we can efficiently perform knowledge distillation on the sphere. We verify our argument via theoretical analysis and ablation study. Extensive experiments have demonstrated the superiority and scalability of our method over the SOTAs.
更多查看译文
关键词
spherical knowledge distillation
AI 理解论文
溯源树
样例
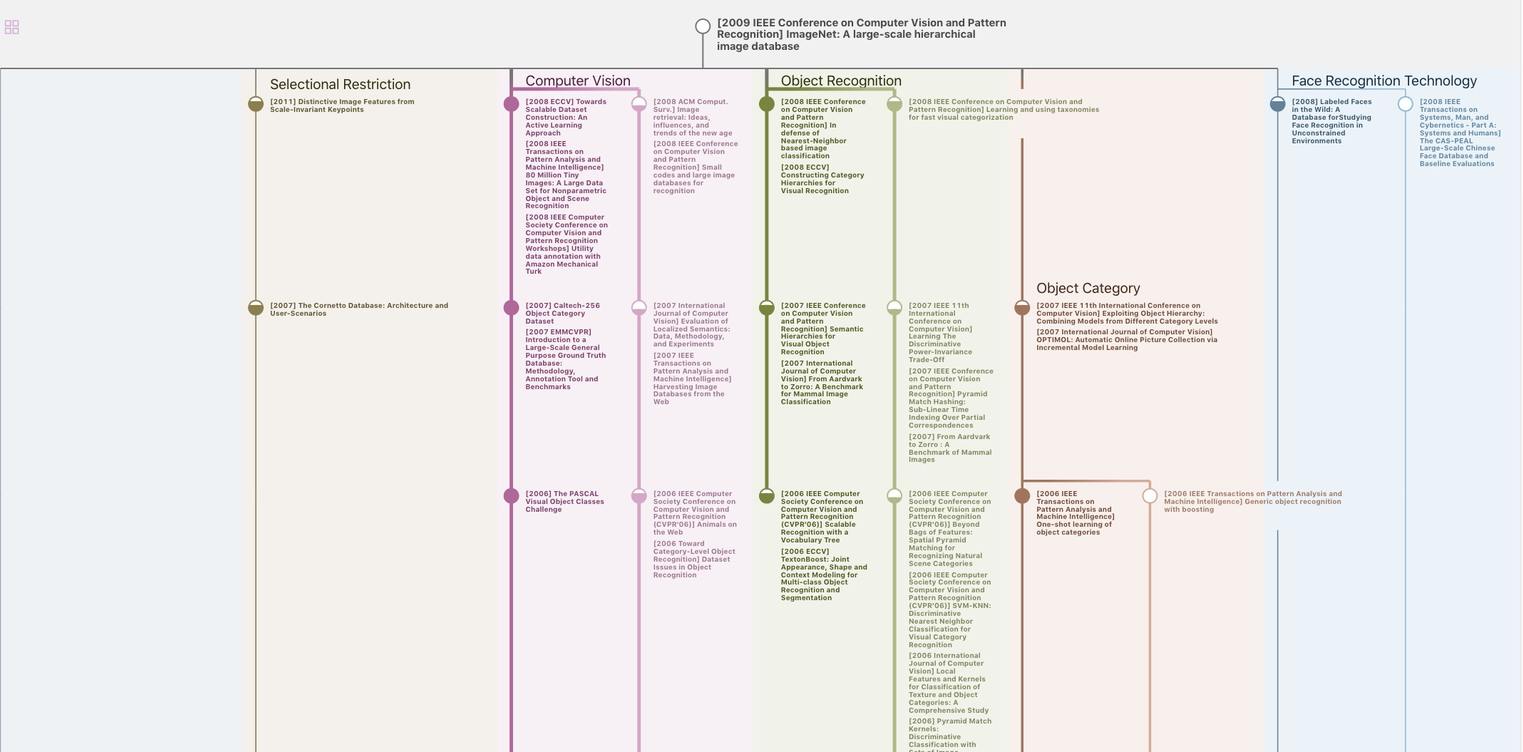
生成溯源树,研究论文发展脉络
Chat Paper
正在生成论文摘要