On Ensemble Learning-Based Secure Fusion Strategy for Robust Cooperative Sensing in Full-Duplex Cognitive Radio Networks
IEEE Transactions on Communications(2020)
摘要
We propose an ensemble machine learning (EML) based robust cooperative spectrum sensing framework in full-duplex cognitive radio networks (FD-CRNs), which is robust with accuracy against malicious attacks and interference. FD communication improves the spectrum awareness capability of secondary users (SUs) by allowing them to sense and transmit simultaneously over the same frequency band. However, it also complicates the sensing environment by introducing self-interference and co-channel interference. Meanwhile, the presence of malicious attacks such as Primary User Emulation and Spectrum Sensing Data Falsification (SSDF) attacks also degrades the sensing performance. To alleviate the influence of interference and attacks, we design an EML framework that provides robust and accurate fusion performance. In such a context, we analyse the spectrum waste probability, collision probability and secondary throughput in both FD Listen-Before-Talk and Listen-And-Talk protocols. Simulation results show that our proposed EML framework can provide lower and more robust false-alarm probability than single-model based fusion methods with the same detection probability constraint for any size of training sets. It also outperforms the conventional majority vote based fusion strategy in terms of spectrum waste probability, collision probability and secondary throughput for any number of SSDF SUs, only at the cost of slightly higher inference time.
更多查看译文
关键词
Sensors,Protocols,Machine learning,Support vector machines,Throughput,Feature extraction,Numerical models
AI 理解论文
溯源树
样例
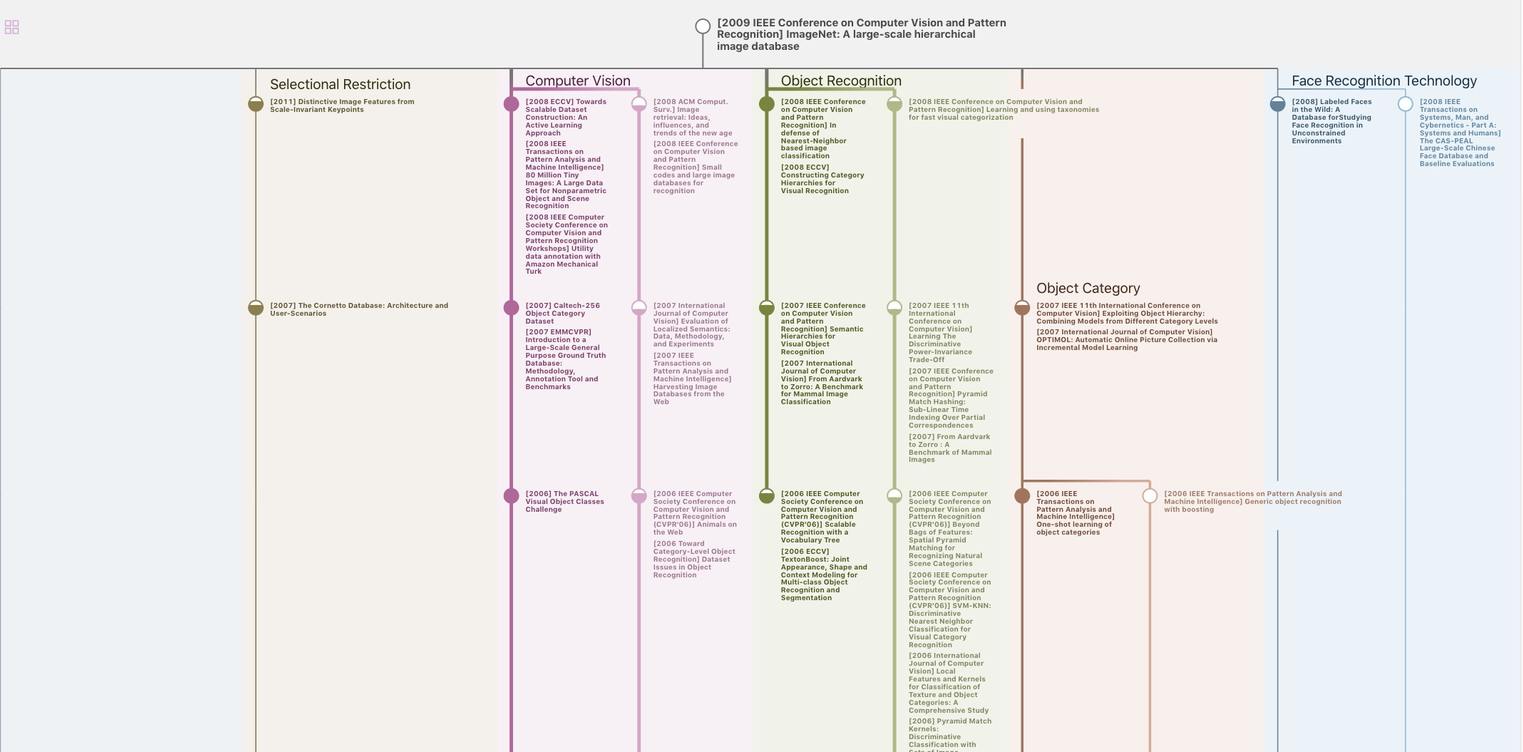
生成溯源树,研究论文发展脉络
Chat Paper
正在生成论文摘要