Detecting and Responding to Information Overload With an Adaptive User Interface
HUMAN FACTORS(2022)
摘要
Objective The objective of this study was to develop and evaluate an adaptive user interface that could detect states of operator information overload and calibrate the amount of information on the screen. Background Machine learning can detect changes in operating context and trigger adaptive user interfaces (AUIs) to accommodate those changes. Operator attentional state represents a promising aspect of operating context for triggering AUIs. Behavioral rather than physiological indices can be used to infer operator attentional state. Method In Experiment 1, a network analysis task sought to induce states of information overload relative to a baseline. Streams of interaction data were taken from these two states and used to train machine learning classifiers. We implemented these classifiers in Experiment 2 to drive an AUI that automatically calibrated the amount of information displayed to operators. Results Experiment 1 successfully induced information overload in participants, resulting in lower accuracy, slower completion time, and higher workload. A series of machine learning classifiers detected states of information overload significantly above chance level. Experiment 2 identified four clusters of users who responded significantly differently to the AUIs. The AUIs benefited performance, completion time, and workload in three clusters. Conclusion Behavioral indices can successfully detect states of information overload and be used to effectively drive an AUI for some user groups. The success of AUIs may be contingent on characteristics of the user group. Application This research applies to domains seeking real-time assessments of user attentional or psychological state.
更多查看译文
关键词
attentional processes, adaptive automation, information overload, passive data monitoring, machine learning
AI 理解论文
溯源树
样例
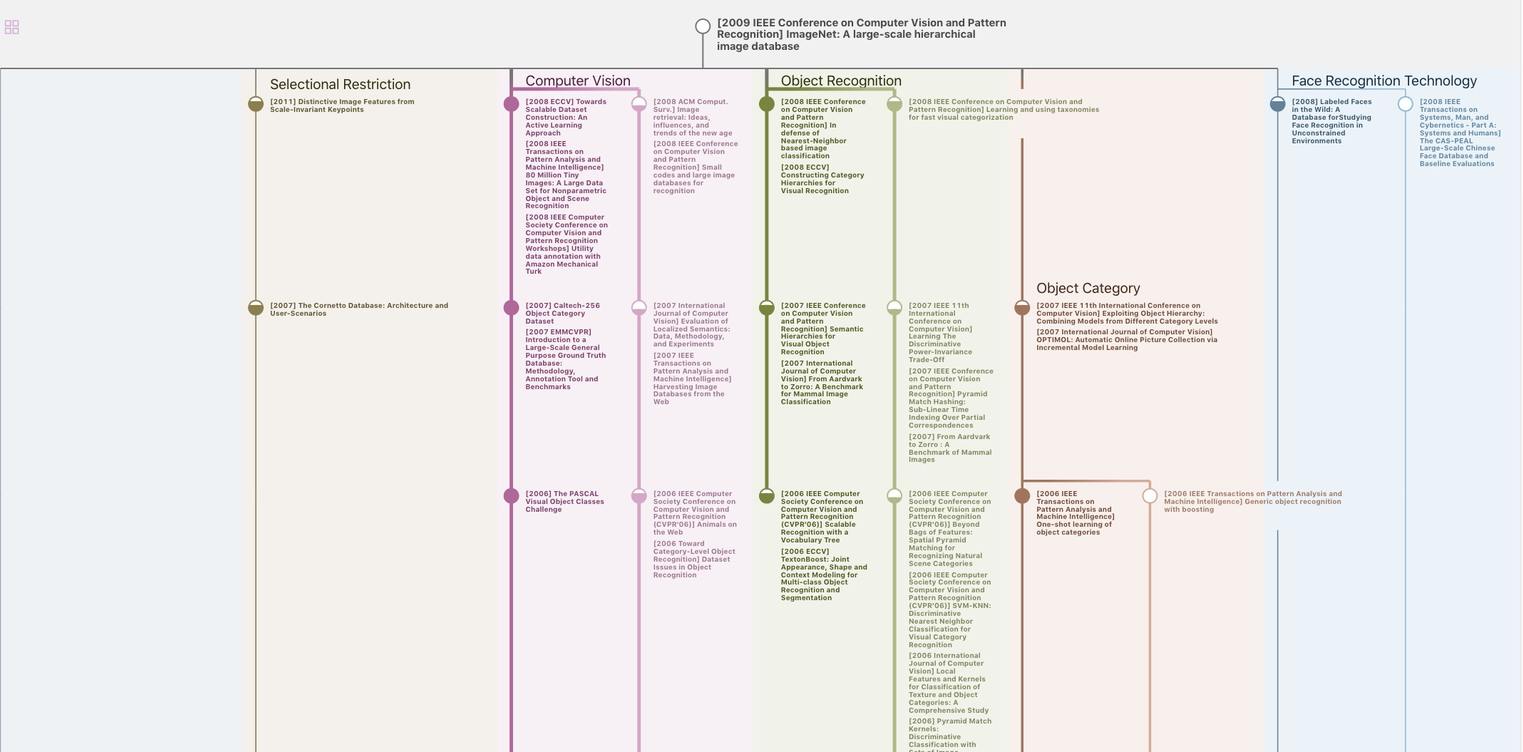
生成溯源树,研究论文发展脉络
Chat Paper
正在生成论文摘要