Machine learning assisted design of γ′-strengthened Co-base superalloys with multi-performance optimization
NPJ COMPUTATIONAL MATERIALS(2020)
摘要
Designing a material with multiple desired properties is a great challenge, especially in a complex material system. Here, we propose a material design strategy to simultaneously optimize multiple targeted properties of multi-component Co-base superalloys via machine learning. The microstructural stability, γ′ solvus temperature, γ′ volume fraction, density, processing window, freezing range, and oxidation resistance were simultaneously optimized. A series of novel Co-base superalloys were successfully selected and experimentally synthesized from >210,000 candidates. The best performer, Co-36Ni-12Al-2Ti-4Ta-1W-2Cr, possesses the highest γ′ solvus temperature of 1266.5 °C without the precipitation of any deleterious phases, a γ′ volume fraction of 74.5% after aging for 1000 h at 1000 °C, a density of 8.68 g cm −3 and good high-temperature oxidation resistance at 1000 °C due to the formation of a protective alumina layer. Our approach paves a new way to rapidly design multi-component materials with desired multi-performance functionality.
更多查看译文
关键词
Computational methods,Metals and alloys,Materials Science,general,Characterization and Evaluation of Materials,Mathematical and Computational Engineering,Theoretical,Mathematical and Computational Physics,Computational Intelligence,Mathematical Modeling and Industrial Mathematics
AI 理解论文
溯源树
样例
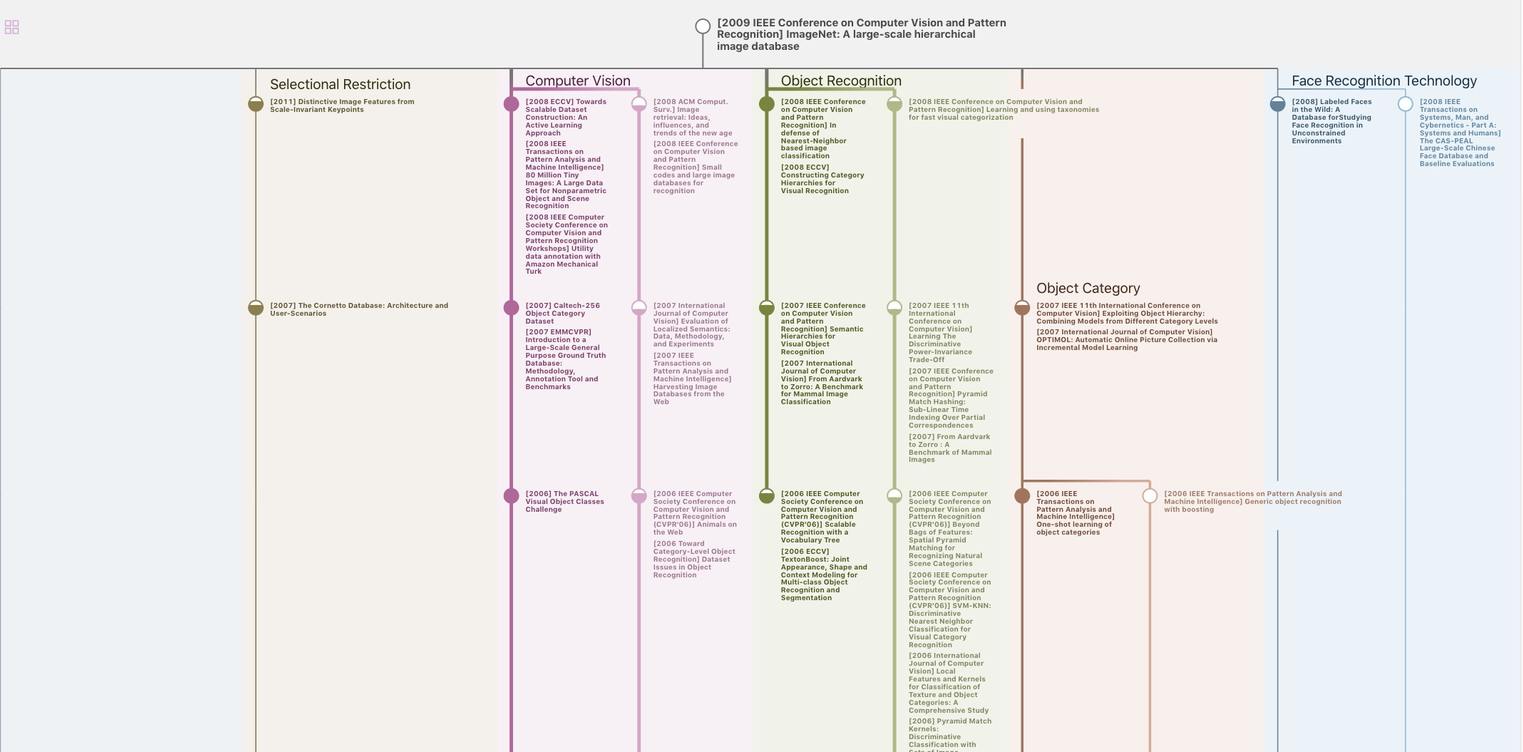
生成溯源树,研究论文发展脉络
Chat Paper
正在生成论文摘要