Cluster-formula-embedded machine learning for design of multicomponent beta-Ti alloys with low Young's modulus
NPJ COMPUTATIONAL MATERIALS(2020)
摘要
The present work formulated a materials design approach, a cluster-formula-embedded machine learning (ML) model, to search for body-centered-cubic (BCC) beta-Ti alloys with low Young's modulus (E) in the Ti-Mo-Nb-Zr-Sn-Ta system. The characteristic parameters, including the Mo equivalence and the cluster-formula approach, are implemented into the ML to ensure the accuracy of prediction, in which the former parameter represents the BCC-beta structural stability, and the latter reflects the interactions among elements expressed with a composition formula. Both auxiliary gradient-boosting regression tree and genetic algorithm methods were adopted to deal with the optimization problem in the ML model. This cluster-formula-embedded ML can not only predict alloy property in the forward design, but also design and optimize alloy compositions with desired properties in multicomponent systems efficiently and accurately. By setting different objective functions, several new beta-Ti alloys with either the lowestE(E = 48 GPa) or a specificE(E = 55 and 60 GPa) were predicted by ML and then validated by a series of experiments, including the microstructural characterization and mechanical measurements. It could be found that the experimentally obtainedEof predicted alloys by ML could reach the desired objectiveE, which indicates that the cluster-formula-embedded ML model can make the prediction and optimization of composition and property more accurate, effective, and controllable.
更多查看译文
关键词
Computational methods,Implants,Metals and alloys,Materials Science,general,Characterization and Evaluation of Materials,Mathematical and Computational Engineering,Theoretical,Mathematical and Computational Physics,Computational Intelligence,Mathematical Modeling and Industrial Mathematics
AI 理解论文
溯源树
样例
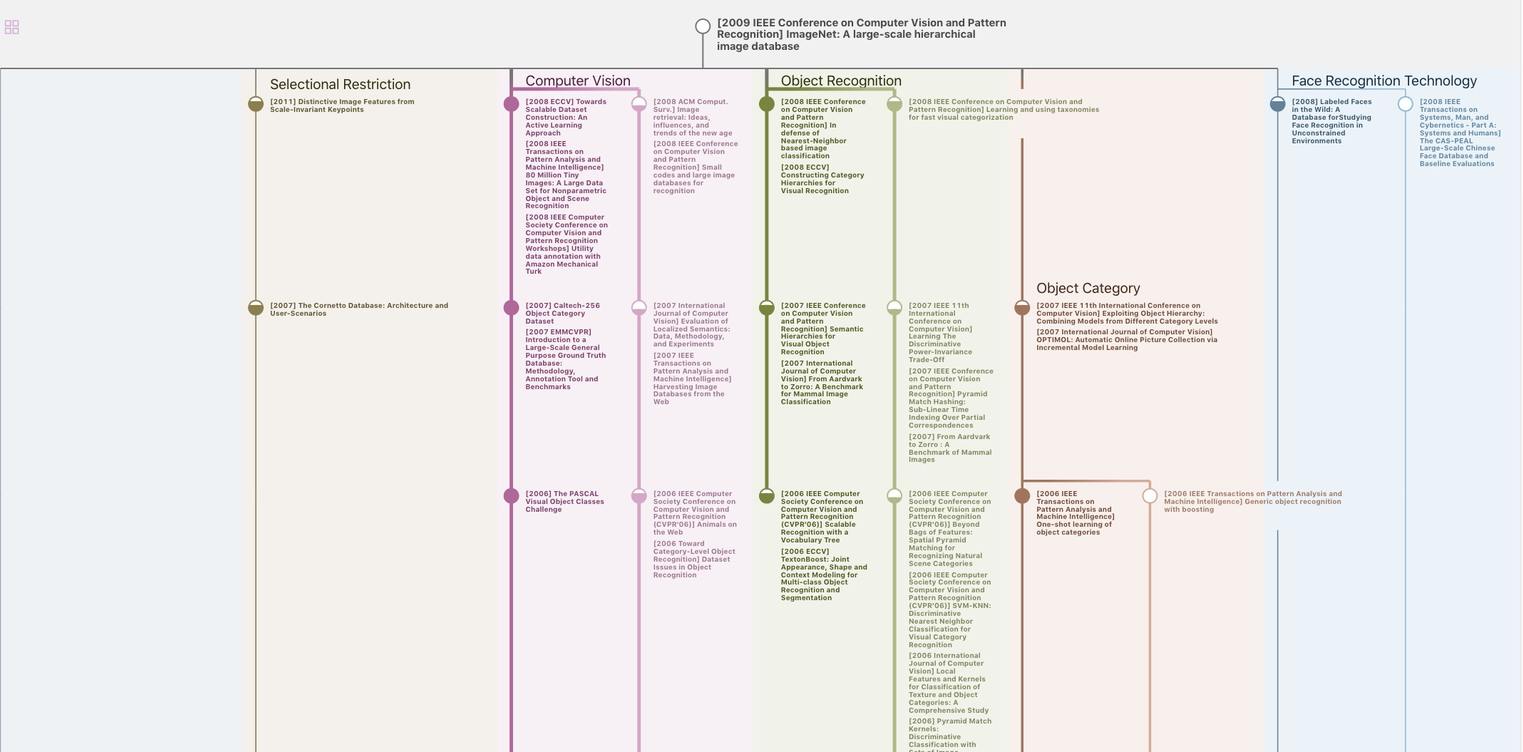
生成溯源树,研究论文发展脉络
Chat Paper
正在生成论文摘要