Differential diagnosis for esophageal protruded lesions using a deep convolution neural network in endoscopic images
Gastrointestinal Endoscopy(2021)
摘要
Background and Aims: Recent advances in deep convolutional neural networks (CNNs) have led to remarkable results in digestive endoscopy. In this study, we aimed to develop CNN-based models for the differential diagnosis of benign esophageal protruded lesions using endoscopic images acquired during real clinical settings.Methods: We retrospectively reviewed the images from 1217 patients who underwent white-light endoscopy (WLE) and EUS between January 2015 and April 2020. Three deep CNN models were developed to accomplish the following tasks: (1) identification of esophageal benign lesions from healthy controls using WLE images; (2) differentiation of 3 subtypes of esophageal protruded lesions (including esophageal leiomyoma [EL], esophageal cyst (EC], and esophageal papilloma [EP]) using WLE images; and (3) discrimination between EL and EC using EUS images. Six endoscopists blinded to the patients' clinical status were enrolled to interpret all images independently. Their diagnostic performances were evaluated and compared with the CNN models using the area under the receiver operating characteristic curve (AUC).Results: For task 1, the CNN model achieved an AUC of 0.751 (95% confidence interval [CI], 0.652-0.850) in identifying benign esophageal lesions. For task 2, the proposed model using WLE images for differentiation of esophageal protruded lesions achieved an AUC of 0.907 (95% CI, 0.835-0.979), 0.897 (95% CI, 0.8410.953), and 0.868 (95% CI, 0.769-0.968) for EP, EL, and EC, respectively. The CNN model achieved equivalent or higher identification accuracy for EL and EC compared with skilled endoscopists. In the task of discriminating EL from EC (task 3), the proposed CNN model had AUC values of 0.739 (EL, 95% CI, 0.600-0.878) and 0.724 (EC, 95% CI, 0.567-0.881), which outperformed seniors and novices. Attempts to combine the CNN and endoscopist predictions led to significantly improved diagnostic accuracy compared with endoscopists interpretations alone.Conclusions: Our team established CNN-based methodologies to recognize benign esophageal protruded lesions using routinely obtained WLE and EUS images. Preliminary results combining the results from the models and the endoscopists underscored the potential of ensemble models for improved differentiation of lesions in real endoscopic settings.
更多查看译文
关键词
AI,AUC,CI,CNN,EC,EL,EP,ROC,WLE
AI 理解论文
溯源树
样例
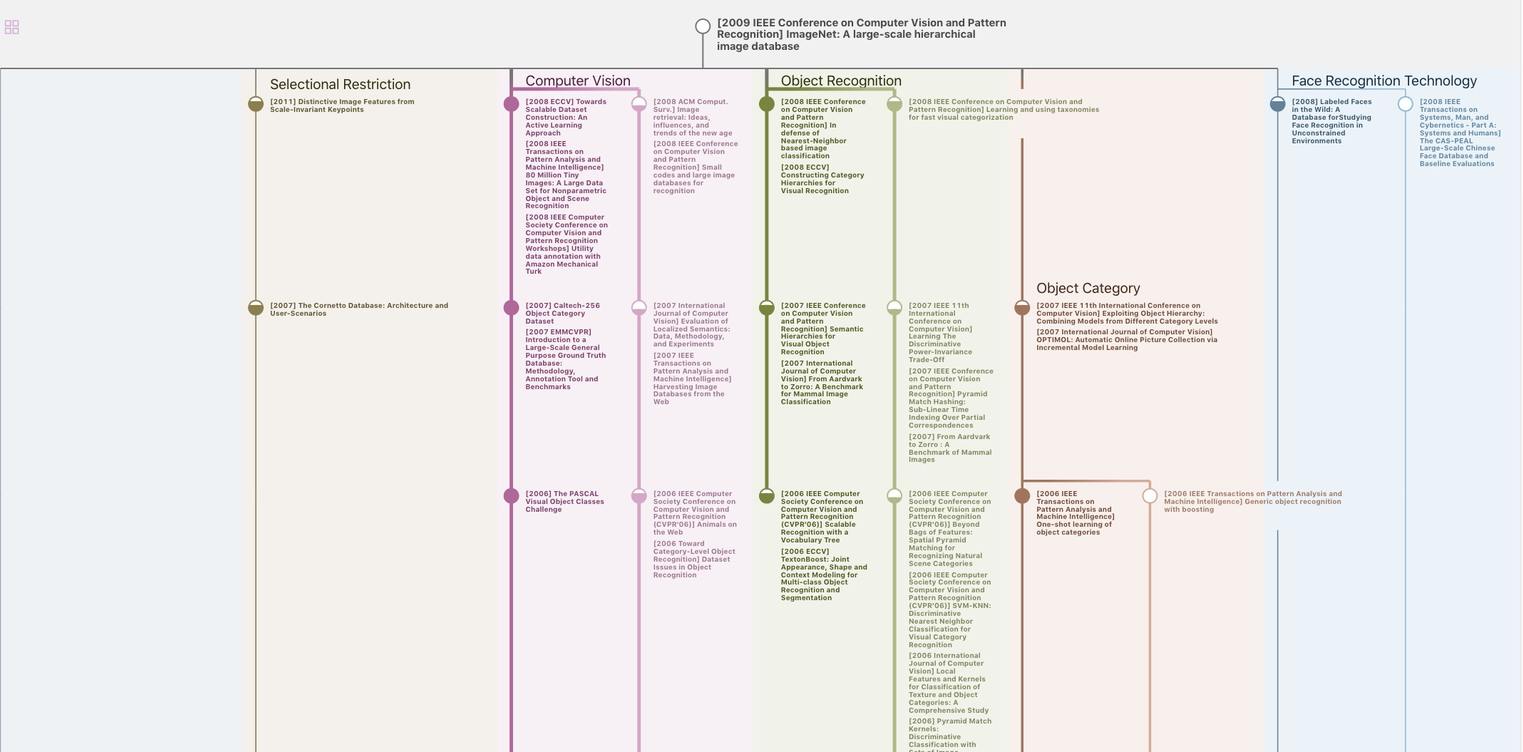
生成溯源树,研究论文发展脉络
Chat Paper
正在生成论文摘要