Individualized prediction of 2-year risk of relapse as indexed by psychiatric hospitalization following psychosis onset: Model development in two first episode samples.
Schizophrenia research(2020)
摘要
BACKGROUND:Although most patients with psychotic disorders experience relapse, it is not possible to predict whether or when an individual patient is going to relapse. We aimed to develop a multifactorial risk prediction algorithm for predicting risk of relapse in first episode psychosis (FEP).
METHODS:Data from two prospectively collected cohorts of FEP patients (N = 1803) were used to develop three multiple logistic prediction models to predict risk of relapse (defined as hospitalization) within the first 2 years of onset of psychosis. Model 1 (M1S1) used data obtained from clinical notes (Sample 1) while model 2 (M2S2) applied the same set of predictors using data obtained from research interviews (Sample 2). The final model (Sample 2: M3S2) used the same predictors plus additional detailed information on predictors. Model performance was evaluated employing measures of overall accuracy, calibration, discrimination and internal validation.
RESULTS:In both samples, the 2-year probability of psychiatric hospitalization was 37%. Of all the models, discrimination accuracy was lowest when limited information (such as socio-demographic and clinical parameters) was included in the prediction model. Model M3S2 using additional information (descriptors of pattern of cannabis, nicotine, alcohol and other illicit drug use) obtained from research interview had the best discrimination accuracy (Harrell's C index 0.749).
CONCLUSIONS:The measures that contributed most to predicting hospitalization are readily accessible in routine clinical practice, suggesting that a risk prediction tool based on these models would be clinically practicable following validation in independent samples and permit a personalized approach to relapse prevention in psychosis.
更多查看译文
AI 理解论文
溯源树
样例
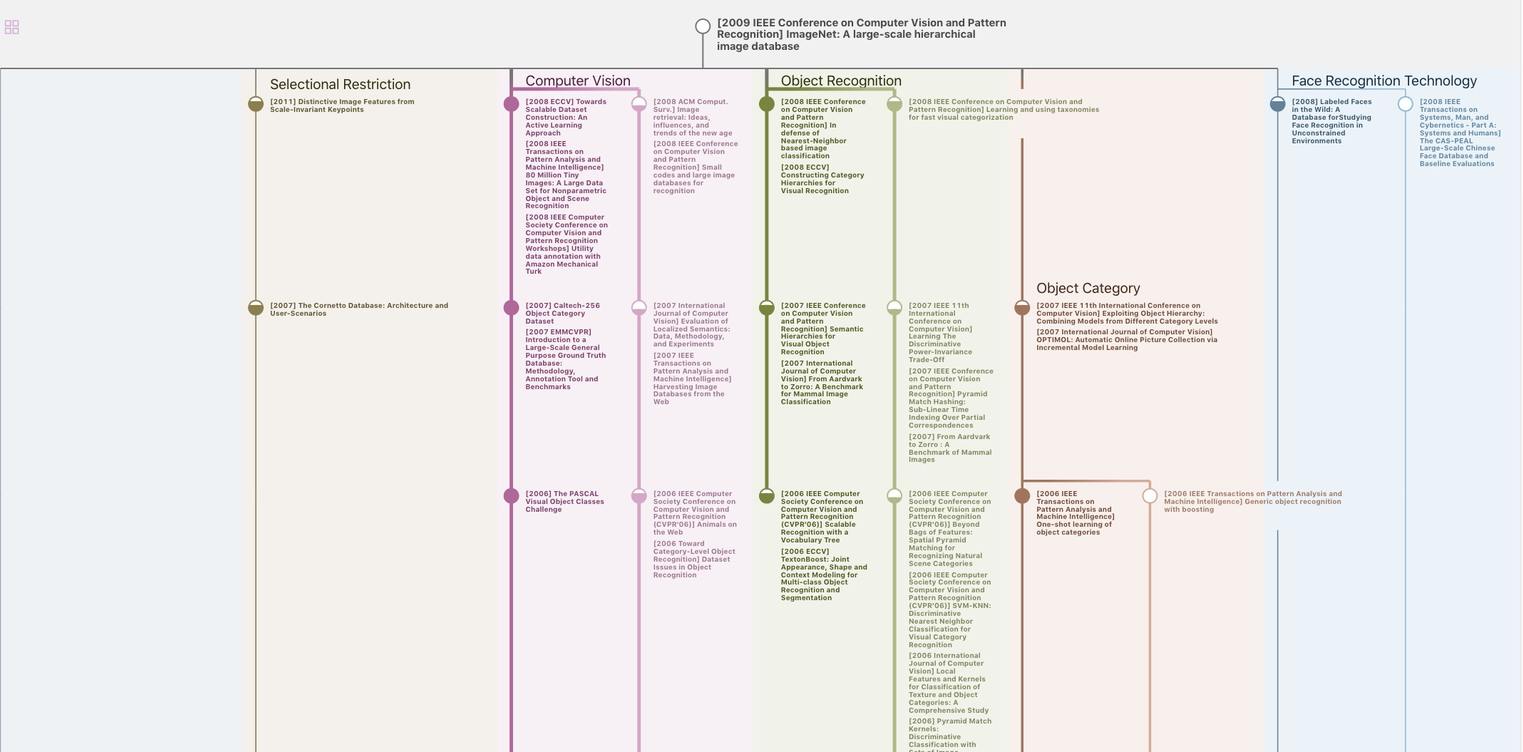
生成溯源树,研究论文发展脉络
Chat Paper
正在生成论文摘要