A Unified Adversarial Learning Framework for Semi supervised Multi target Domain Adaptation
user-5d54d8d2530c705f51c2f7fc(2020)
摘要
Machine learning algorithms have been criticized as difficult to apply to new tasks or datasets without sufficient annotations. Domain adaptation is expected to tackle this problem by establishing knowledge transfer from a labeled source domain to an unlabeled or sparsely labeled target domain. Most existing domain adaptation models focus on the single-source-single-target scenario. However, the pairwise domain adaptation approaches may lead to suboptimal performance when there are multiple target domains available, because the information from other related target domains is not being utilized. In this work, we propose a unified semi-supervised multi-target domain adaptation framework to implement knowledge transfer among multiple domains (a single source domain and multiple target domains). Specifically, we aim to learn an embedded space and minimize the marginal probability distribution differences among all domains in the space. Meanwhile, we introduce Prototypical Networks to perform classification, and extend it to semi-supervised settings. On this basis, we further align the conditional probability distributions among the domains by generating pseudo-labels for the unlabeled target data and training the model with bootstrapping method. Extensive sentiment analysis experiments show that our approach significantly outperforms several state-of-the-art methods.
更多查看译文
关键词
Domain adaptation, Adversarial learning, Semi-supervised, Prototypical networks, Self-training, Sentiment analysis
AI 理解论文
溯源树
样例
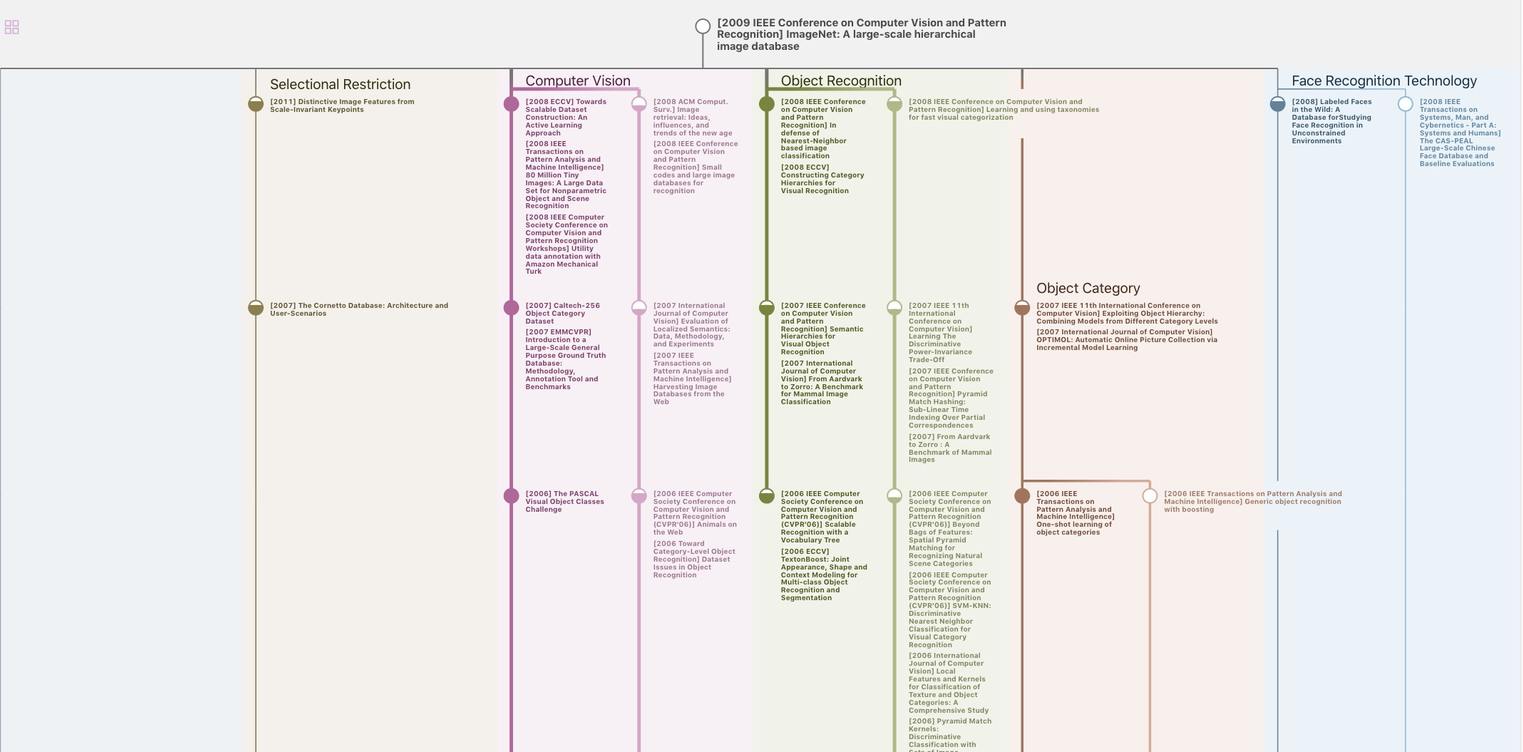
生成溯源树,研究论文发展脉络
Chat Paper
正在生成论文摘要