Learning from structured data: theory, algorithms, and applications
user-5f1692da4c775ed682f59262(2018)
摘要
With the unprecedented growth of massive data sets in modern data analytics, problems being investigated in machine learning and statistics often feature high-dimensional, sequential, and quantized data. For instance, in YouTube millions of new videos are uploaded every day (ie, sequential), each of which contains tremendous contents (ie, high-dimensional) while the user feedback is simply “thumbs up” or “thumbs down”(ie, quantized). These problem characteristics pose new challenges to computer scientists and statisticians, both in the statistical and computational aspects. On the statistical side, a long-term research line is to understand the fundamental limits imposed by the properties of the problems, and to determine the sample size under which accurate estimation of model parameters is possible. The question has been well-understood if there are sufficient samples or the sample size tends to infinity, which is known as asymptotic analysis. However, in the high-dimensional regime, the number of observations is typically of the same order of, or even smaller than the number of unknown parameters. Classical results immediately break down in this situation. As a matter of fact, it is not possible to identify the model without further information. On the computational side, parameter estimation usually boils down to solving an optimization problem, either convex or non-convex, and the regard (or objective) is to design efficient algorithms that achieve the optimal computational efficiency. While there have been numerous solvers developed in the last decades, they are typically not scalable to very large-scale data sets since the computational …
更多查看译文
AI 理解论文
溯源树
样例
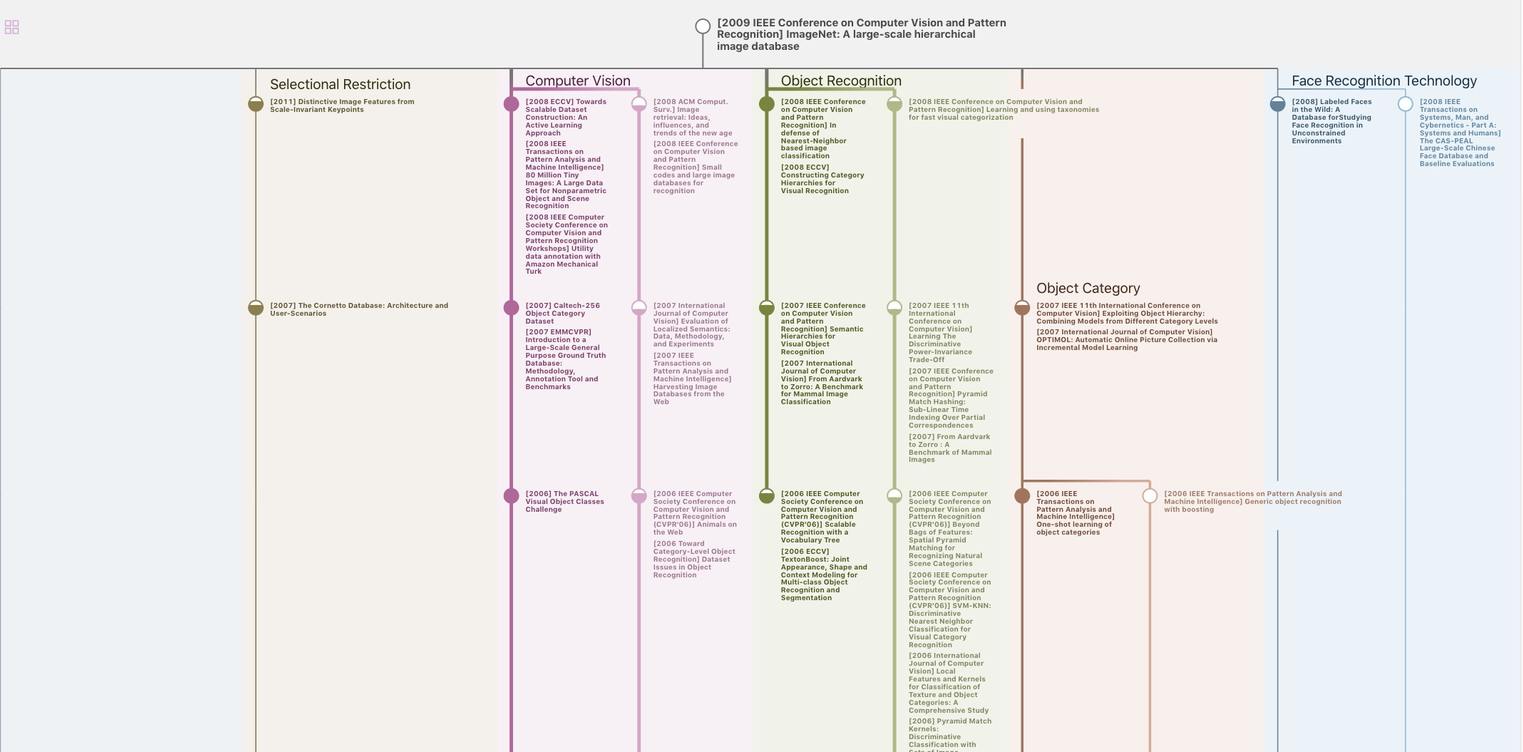
生成溯源树,研究论文发展脉络
Chat Paper
正在生成论文摘要