Tolerant Markov Boundary Discovery for Feature Selection
CIKM '20: The 29th ACM International Conference on Information and Knowledge Management Virtual Event Ireland October, 2020(2020)
摘要
Due to the interpretability and robustness, Markov boundary (MB) has received much attention and been widely applied to causal feature selection. However, enormous empirical studies show that, existing algorithms achieve outstanding performance only on the standard Bayesian network data. While on the real-world data, they could not identify some of the relevant features since the large conditioning set and the ignored multivariate dependence lead to performance degradation. In this paper, we propose a tolerant MB discovery algorithm (TLMB), which maps the feature space and target space to a reproducing kernel Hilbert space through the conditional covariance operator, to measure the causal information carried by a feature. Specifically, TLMB uses a score function to filter the redundant features first and then minimize the trace of the conditional covariance operator, where both of the score function and the optimization problem work in the reproducing kernel Hilbert space so that TLMB can select features with not only pairwise dependence but also multivariate dependence. Moreover, as a MB-based method, TLMB can automatically determine the number of selected features due to the property of MB.
更多查看译文
关键词
Markov boundary, feature selection, kernel method
AI 理解论文
溯源树
样例
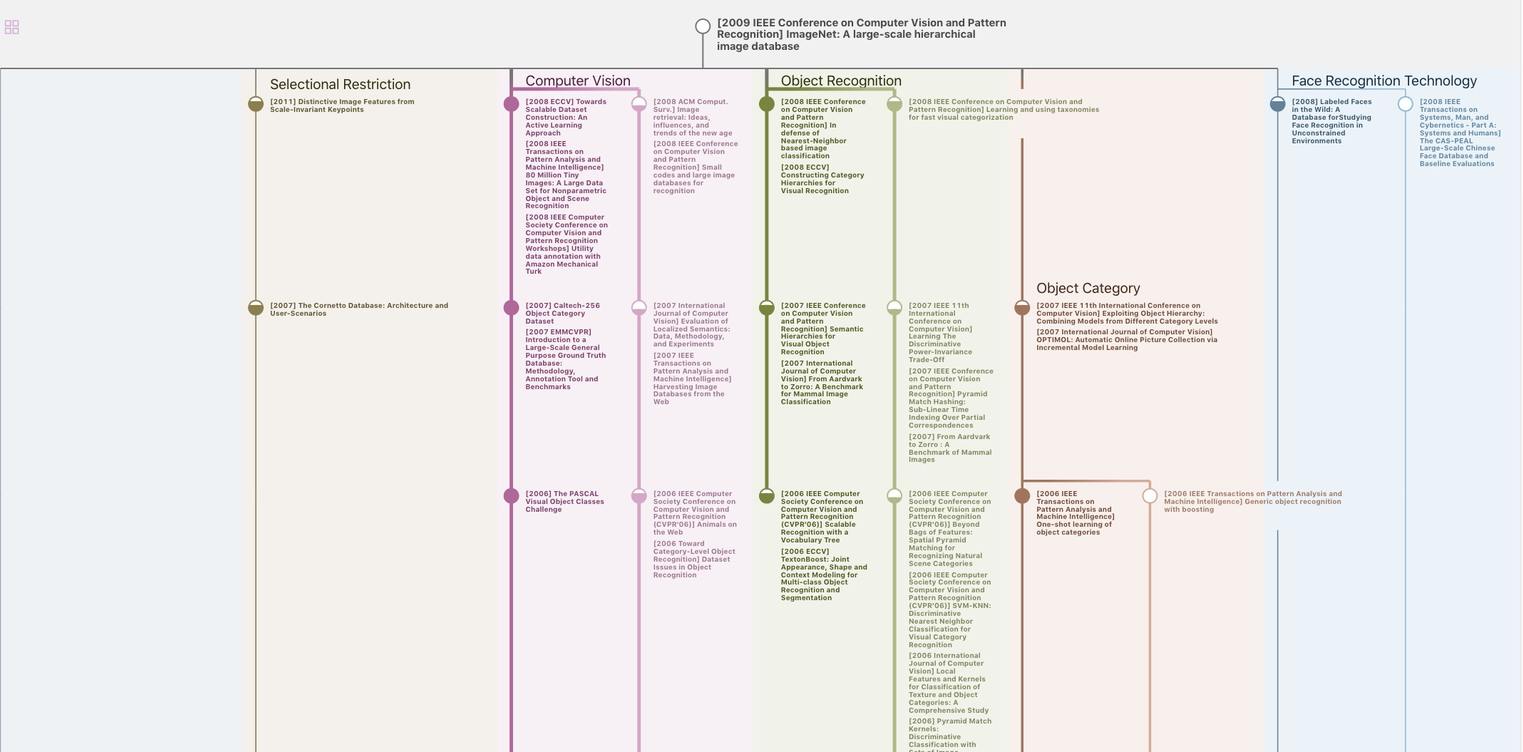
生成溯源树,研究论文发展脉络
Chat Paper
正在生成论文摘要