Imbalanced Time Series Classification for Flight Data Analyzing with Nonlinear Granger Causality Learning
CIKM '20: The 29th ACM International Conference on Information and Knowledge Management Virtual Event Ireland October, 2020(2020)
摘要
Identifying the faulty class of multivariate time series is crucial for today's flight data analysis. However, most of the existing time series classification methods suffer from imbalanced data and lack of model interpretability, especially on flight data of which faulty events are usually uncommon with a limited amount of data. Here, we present a neural network classification model for imbalanced multivariate time series by leveraging the information learned from normal class, which can also learn the nonlinear Granger causality for each class, so that we can pinpoint how time series classes differ from each other. Experiments on simulated data and real flight data shows that this model can achieve high accuracy of identifying anomalous flights.
更多查看译文
关键词
full flight data, time series classification, interpretability
AI 理解论文
溯源树
样例
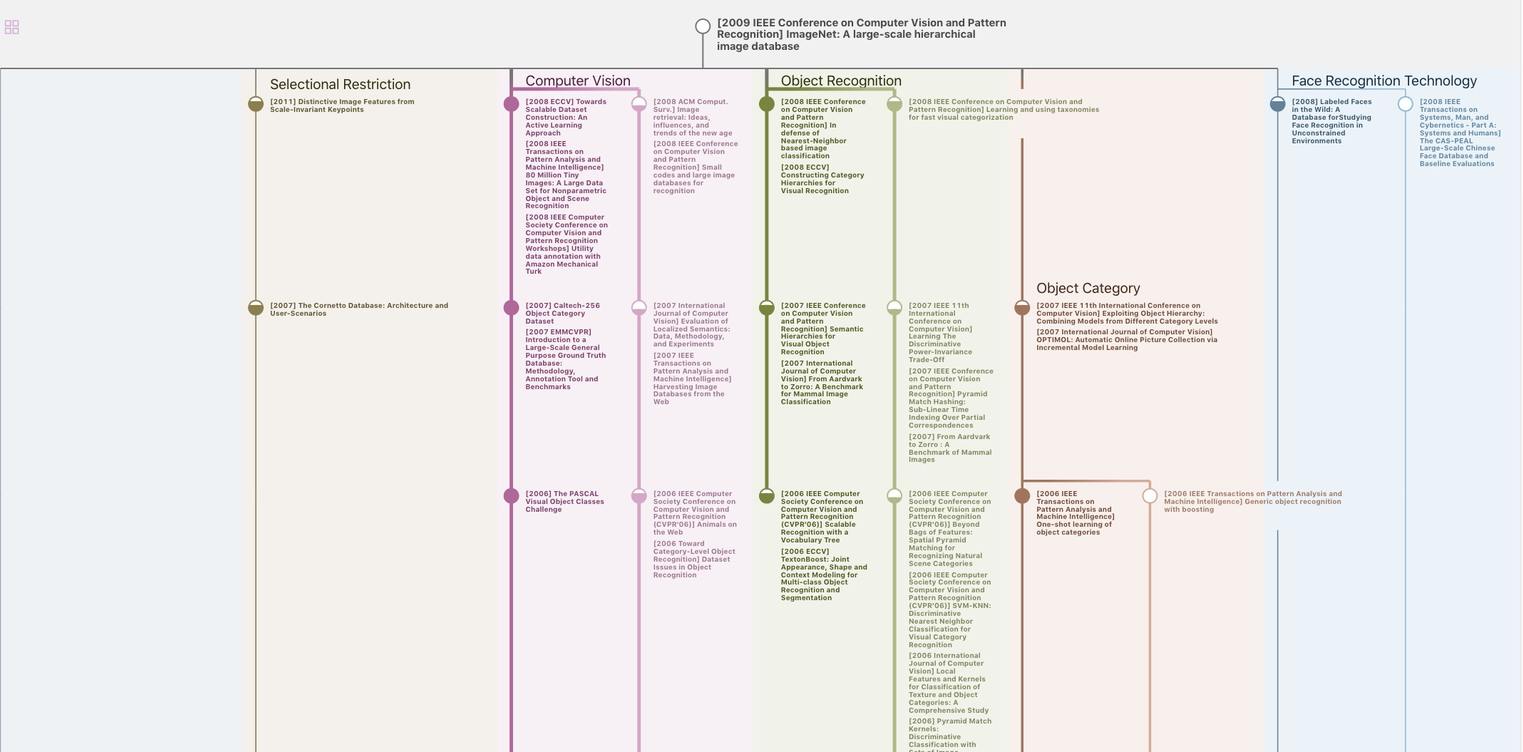
生成溯源树,研究论文发展脉络
Chat Paper
正在生成论文摘要