Offline Evaluation by Maximum Similarity to an Ideal Ranking
CIKM '20: The 29th ACM International Conference on Information and Knowledge Management Virtual Event Ireland October, 2020(2020)
摘要
NDCG and similar measures remain standard for the offline evaluation of search, recommendation, question answering and similar systems. These measures require definitions for two or more relevance levels, which human assessors then apply to judge individual documents. Due to this dependence on a definition of relevance, it can be difficult to extend these measures to account for factors beyond relevance. Rather than propose extensions to these measures, we instead propose a radical simplification to replace them. For each query, we define a set of ideal rankings and compute the maximum rank similarity between members of this set and an actual ranking generated by a system. This maximum similarity to an ideal ranking becomes our effectiveness measure, replacing NDCG and similar measures. We propose rank biased overlap (RBO) to compute this rank similarity, since it was specifically created to address the requirements of rank similarity between search results. As examples, we explore ideal rankings that account for document length, diversity, and correctness.
更多查看译文
AI 理解论文
溯源树
样例
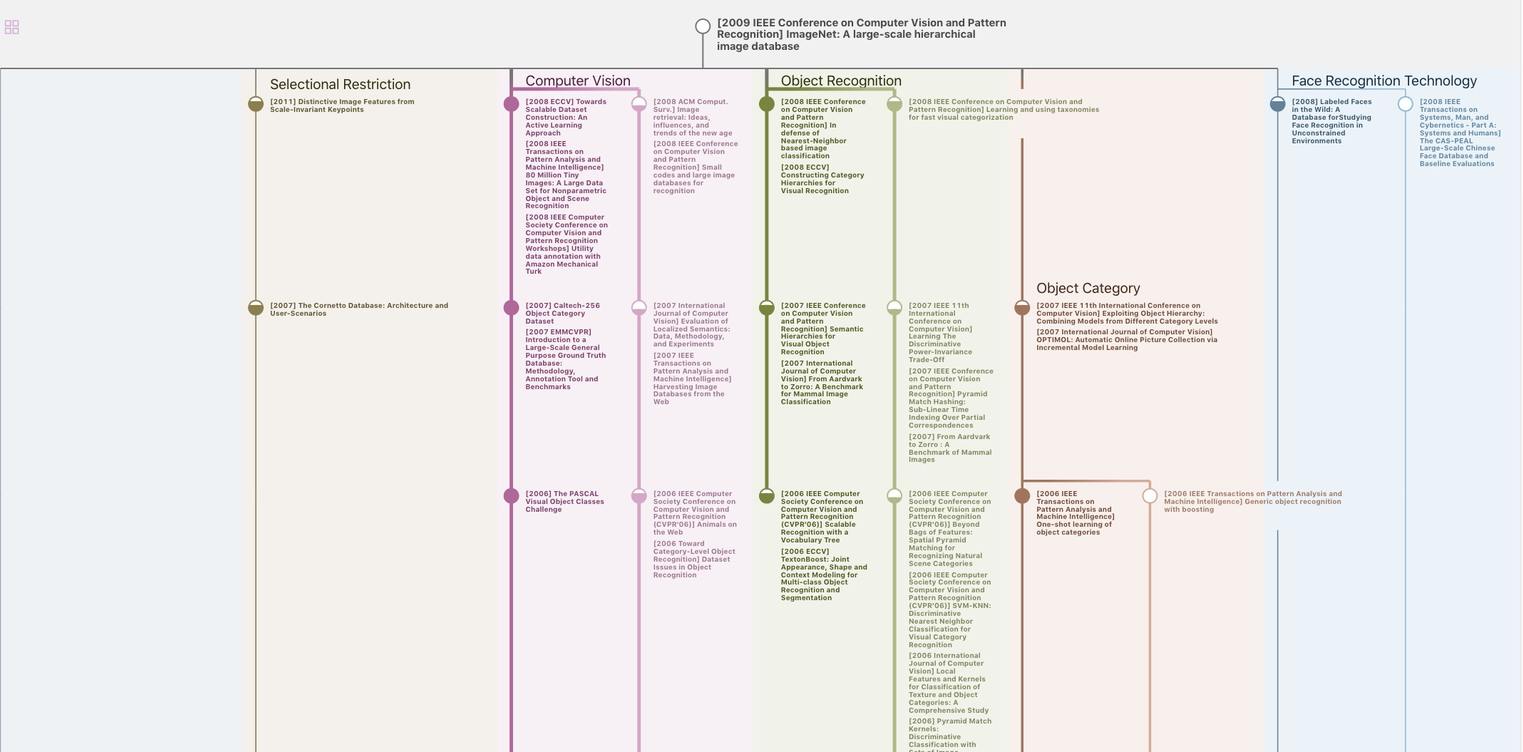
生成溯源树,研究论文发展脉络
Chat Paper
正在生成论文摘要