Generative Adversarial Attributed Network Anomaly Detection
CIKM '20: The 29th ACM International Conference on Information and Knowledge Management Virtual Event Ireland October, 2020(2020)
摘要
Anomaly detection is a useful technique in many applications such as network security and fraud detection. Due to the insufficiency of anomaly samples as training data, it is usually formulated as an unsupervised model learning problem. In recent years there is a surge of adopting graph data structure in numerous applications. Detecting anomaly in an attributed network is more challenging than the sample based task because of the sample information representations in the form of graph nodes and edges. In this paper, we propose a generative adversarial attributed network (GAAN) anomaly detection framework. The fake graph nodes are generated by a generator module with Gaussian noise as input. An encoder module is employed to map both real and fake graph nodes into a latent space. To encode the graph structure information into the node latent representation, we compute the sample covariance matrix for real nodes and fake nodes respectively. A discriminator is trained to recognize whether two connected nodes are from the real or fake graph. With the learned encoder module output, an anomaly evaluation measurement considering the sample reconstruction error and real-sample identification confidence is employed to make prediction. We conduct extensive experiments on benchmark datasets and compare with state-of-the-art attributed graph anomaly detection methods. The superior AUC score demonstrates the effectiveness of the proposed method.
更多查看译文
关键词
GAN, anomaly detection, attributed networks
AI 理解论文
溯源树
样例
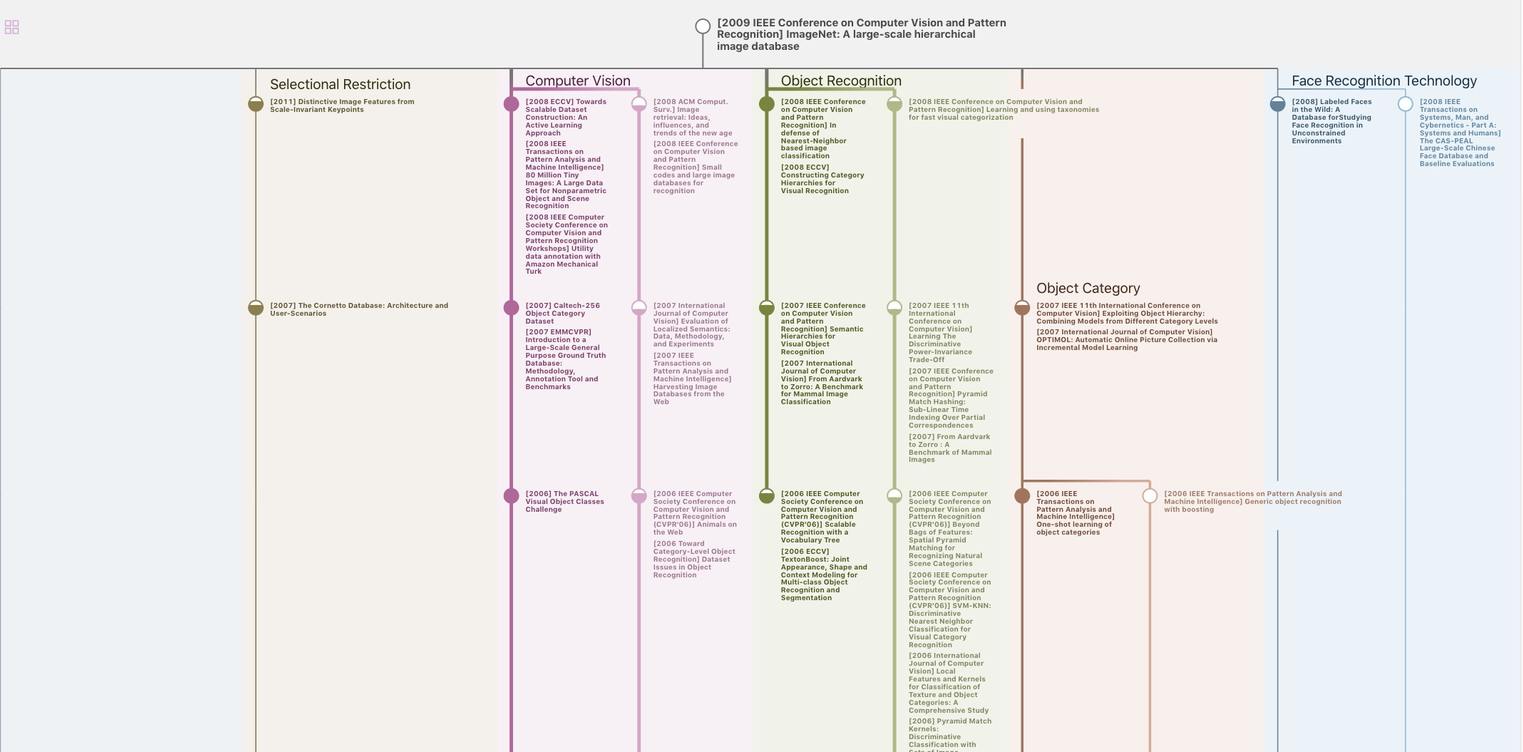
生成溯源树,研究论文发展脉络
Chat Paper
正在生成论文摘要