First-Person Video Domain Adaptation with Multi-Scene Cross-Site Datasets and Attention-Based Methods
IEEE TRANSACTIONS ON CIRCUITS AND SYSTEMS FOR VIDEO TECHNOLOGY(2023)
Chinese Acad Sci | Univ Sheffield
Abstract
Unsupervised Domain Adaptation (UDA) can transfer knowledge from labeled source data to unlabeled target data of the same categories. However, UDA for first-person video action recognition is an under-explored problem, with a lack of benchmark datasets and limited consideration of first-person video characteristics. Existing benchmark datasets provide videos with a single activity scene, e.g. kitchen, and similar global video statistics. However, multiple activity scenes and different global video statistics are still essential for developing robust UDA networks for real-world applications. To this end, we first introduce two first-person video domain adaptation datasets: ADL-7 and GTEA_KITCHEN-6. To the best of our knowledge, they are the first to provide multi-scene and cross-site settings for UDA problem on first-person video action recognition, promoting diversity. They provide five more domains based on the original three from existing datasets, enriching data for this area. They are also compatible with existing datasets, ensuring scalability. First-person videos have unique challenges, i.e. actions tend to occur in hand-object interaction areas. Therefore, networks paying more attention to such areas can benefit common feature learning in UDA. Attention mechanisms can endow networks with the ability to allocate resources adaptively for the important parts of the inputs and fade out the rest. Hence, we introduce channel-temporal attention modules to capture the channel-wise and temporal-wise relationships and model their inter-dependencies important to this characteristic. Moreover, we propose a Channel-Temporal Attention Network (CTAN) to integrate these modules into existing architectures. CTAN outperforms baselines on the new datasets and one existing dataset, EPIC-8.
MoreTranslated text
Key words
Action recognition,unsupervised domain adaptation,first-person vision,channel-temporal attention
求助PDF
上传PDF
View via Publisher
AI Read Science
AI Summary
AI Summary is the key point extracted automatically understanding the full text of the paper, including the background, methods, results, conclusions, icons and other key content, so that you can get the outline of the paper at a glance.
Example
Background
Key content
Introduction
Methods
Results
Related work
Fund
Key content
- Pretraining has recently greatly promoted the development of natural language processing (NLP)
- We show that M6 outperforms the baselines in multimodal downstream tasks, and the large M6 with 10 parameters can reach a better performance
- We propose a method called M6 that is able to process information of multiple modalities and perform both single-modal and cross-modal understanding and generation
- The model is scaled to large model with 10 billion parameters with sophisticated deployment, and the 10 -parameter M6-large is the largest pretrained model in Chinese
- Experimental results show that our proposed M6 outperforms the baseline in a number of downstream tasks concerning both single modality and multiple modalities We will continue the pretraining of extremely large models by increasing data to explore the limit of its performance
Upload PDF to Generate Summary
Must-Reading Tree
Example
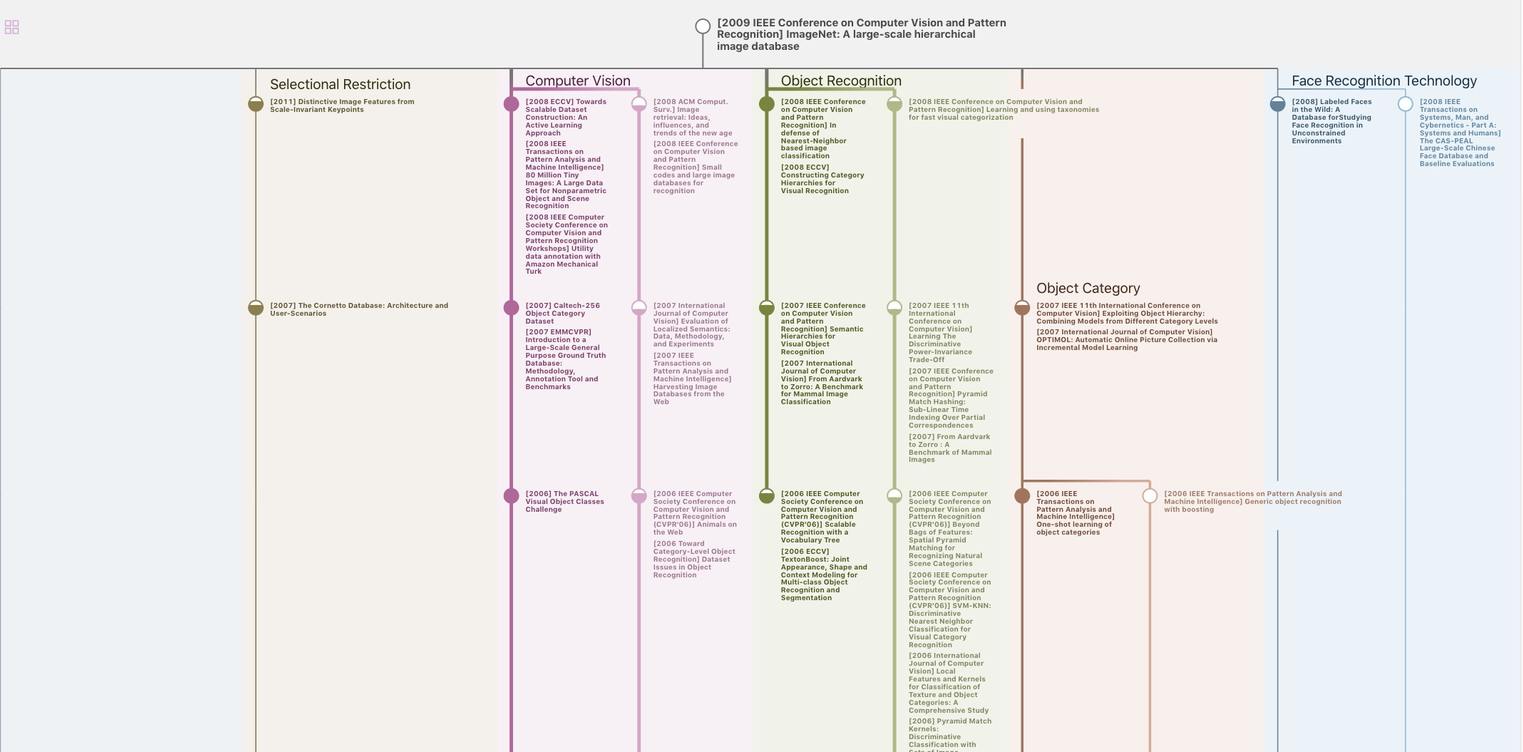
Generate MRT to find the research sequence of this paper
Related Papers
2020
被引用610 | 浏览
2017
被引用799 | 浏览
2018
被引用207 | 浏览
2018
被引用136 | 浏览
2023
被引用131 | 浏览
2021
被引用55 | 浏览
2021
被引用34 | 浏览
2022
被引用50 | 浏览
Data Disclaimer
The page data are from open Internet sources, cooperative publishers and automatic analysis results through AI technology. We do not make any commitments and guarantees for the validity, accuracy, correctness, reliability, completeness and timeliness of the page data. If you have any questions, please contact us by email: report@aminer.cn
Chat Paper
GPU is busy, summary generation fails
Rerequest