Characterizing and Taming Model Instability Across Edge Devices.
Conference on Machine Learning and Systems (MLSys)(2021)
摘要
The same machine learning model running on different edge devices may produce highly-divergent outputs on a nearly-identical input. Possible reasons for the divergence include differences in the device sensors, the device's signal processing hardware and software, and its operating system and processors. This paper presents the first methodical characterization of the variations in model prediction across real-world mobile devices. We demonstrate that accuracy is not a useful metric to characterize prediction divergence, and introduce a new metric, instability, which captures this variation. We characterize different sources for instability, and show that differences in compression formats and image signal processing account for significant instability in object classification models. Notably, in our experiments, 14-17% of images produced divergent classifications across one or more phone models. We evaluate three different techniques for reducing instability. In particular, we adapt prior work on making models robust to noise in order to fine-tune models to be robust to variations across edge devices. We demonstrate our fine-tuning techniques reduce instability by 75%.
更多查看译文
关键词
instability,edge,model
AI 理解论文
溯源树
样例
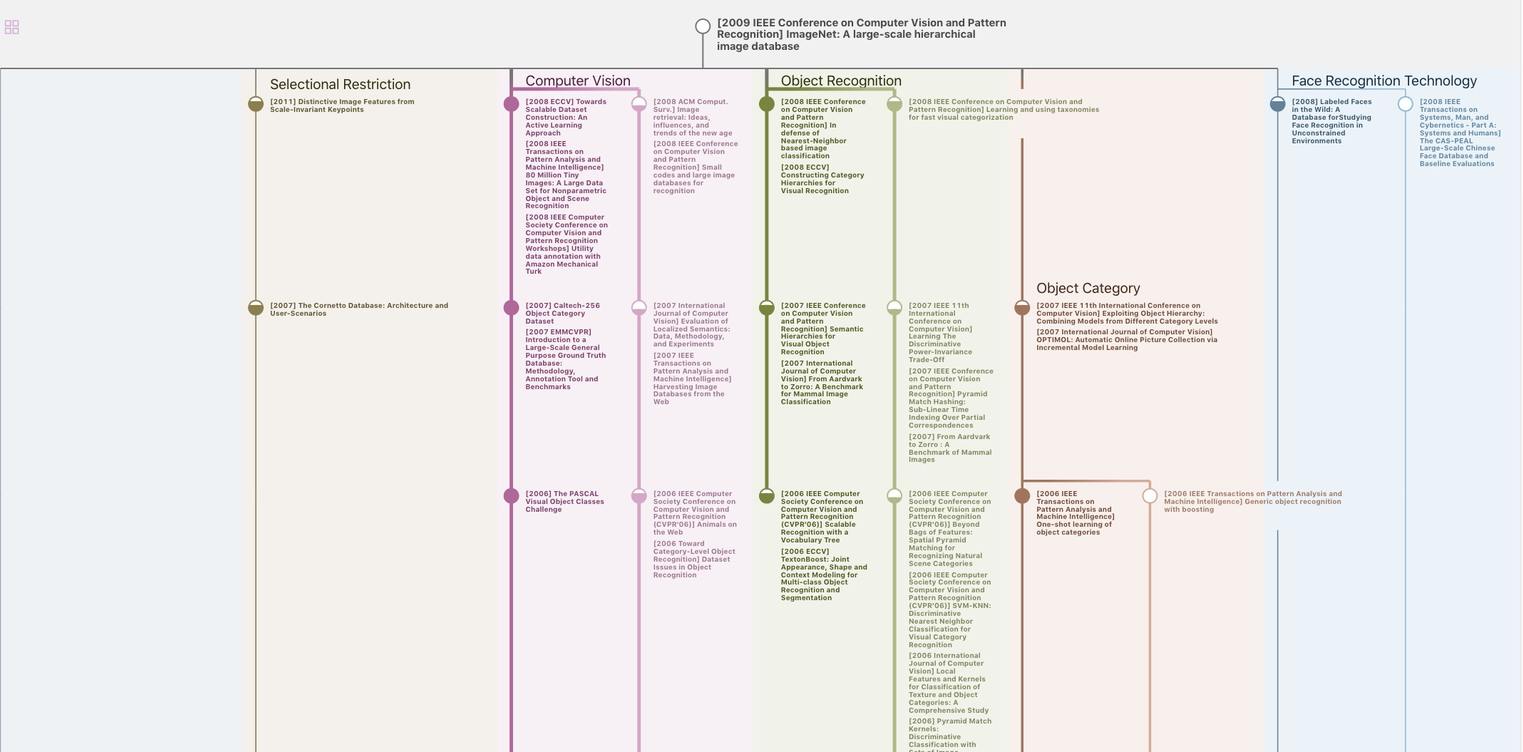
生成溯源树,研究论文发展脉络
Chat Paper
正在生成论文摘要