Diversifying Search Results using Self-Attention Network
CIKM '20: The 29th ACM International Conference on Information and Knowledge Management Virtual Event Ireland October, 2020(2020)
摘要
Search results returned by search engines need to be diversified in order to satisfy different information needs of different users. Several supervised learning models have been proposed for diversifying search results in recent years. Most of the existing supervised methods greedily compare each candidate document with the selected document sequence and select the next local optimal document. However, the information utility of each candidate document is not independent with each other, and research has shown that the selection of a candidate document will affect the utilities of other candidate documents. As a result, the local optimal document rankings will not lead to the global optimal rankings. In this paper, we propose a new supervised diversification framework to address this issue. Based on a self-attention encoder-decoder structure, the model can take the whole candidate document sequence as input, and simultaneously leverage both the novelty and the subtopic coverage of the candidate documents. We call this framework Diversity Encoder with Self-Attention (DESA). Comparing with existing supervised methods, this framework can model the interactions between all candidate documents and return their diversification scores based on the whole candidate document sequence. Experimental results show that our proposed framework outperforms existing methods. These results confirm the effectiveness of modeling all the candidate documents for the overall novelty and subtopic coverage globally, instead of comparing every single candidate document with the selected sequence document selection.
更多查看译文
关键词
Search Result Diversification, Self Attention
AI 理解论文
溯源树
样例
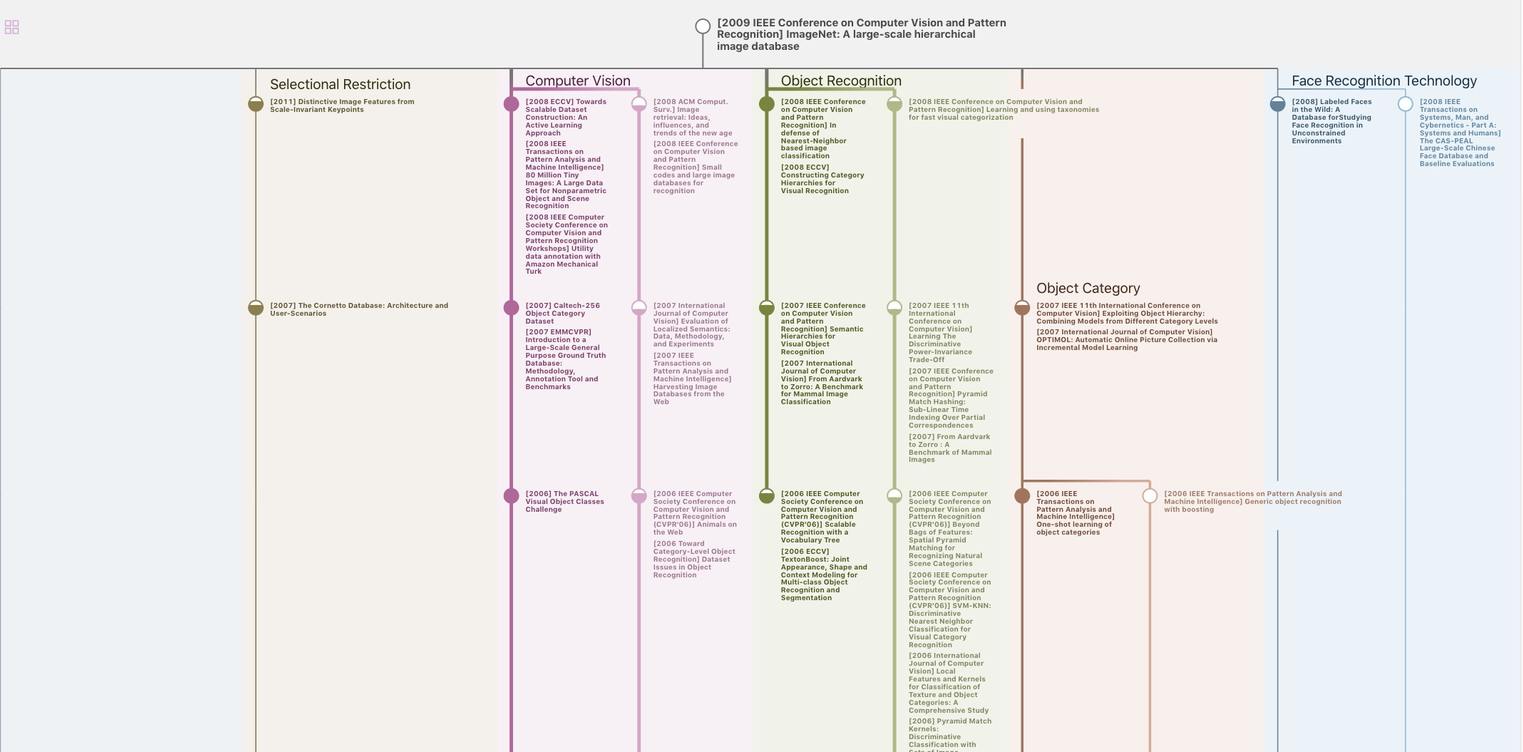
生成溯源树,研究论文发展脉络
Chat Paper
正在生成论文摘要