Probabilistic Dynamic Non-negative Group Factor Model for Multi-source Text Mining
CIKM '20: The 29th ACM International Conference on Information and Knowledge Management Virtual Event Ireland October, 2020(2020)
摘要
Nonnegative matrix factorization (NMF) is a popular approach to model data, however, most models are unable to flexibly take into account multiple matrices across sources and time or apply only to integer-valued data. We introduce a probabilistic, Gaussian Process-based, more inclusive NMF-based model which jointly analyzes nonnegative data such as text data word content from multiple sources in a temporal dynamic manner. The model collectively models observed matrix data, source-wise latent variables, and their dependencies and temporal evolution with a full-fledged hierarchical approach including flexible nonparametric temporal dynamics. Experiments on simulated data and real data show the model out-performs, comparable models. A case study on social media and news demonstrates the model discovers semantically meaningful topical factors and their evolution
更多查看译文
关键词
Nonnegative Matrix Factorization, Gaussian Process, Multiple Sources
AI 理解论文
溯源树
样例
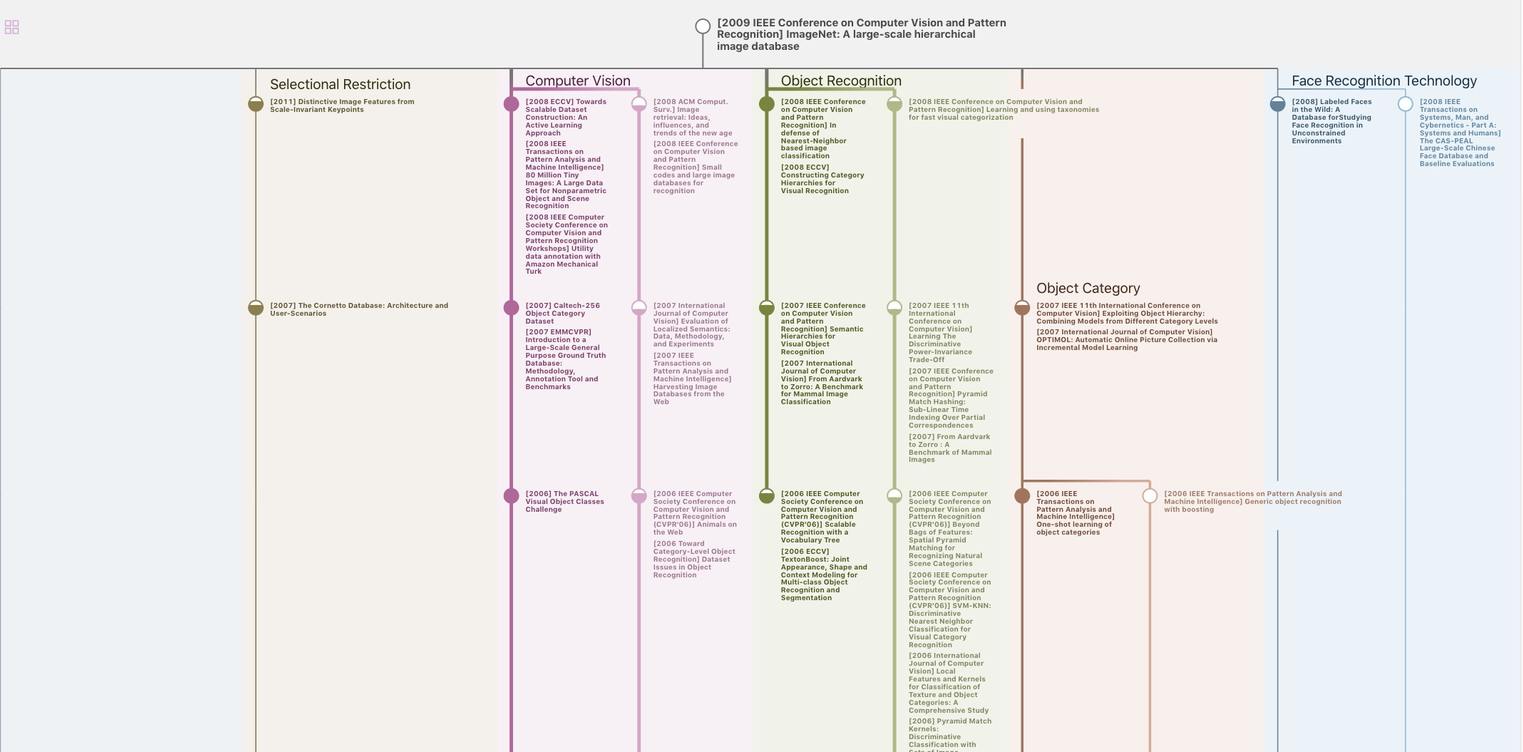
生成溯源树,研究论文发展脉络
Chat Paper
正在生成论文摘要