BERTnesia: Investigating the capture and forgetting of knowledge in BERT
arxiv(2021)
摘要
Probing complex language models has recently revealed several insights into linguistic and semantic patterns found in the learned representations. In this paper, we probe BERT specifically to understand and measure the relational knowledge it captures. We utilize knowledge base completion tasks to probe every layer of pre-trained as well as fine-tuned BERT (ranking, question answering, NER). Our findings show that knowledge is not just contained in BERT's final layers. Intermediate layers contribute a significant amount (17-60%) to the total knowledge found. Probing intermediate layers also reveals how different types of knowledge emerge at varying rates. When BERT is fine-tuned, relational knowledge is forgotten but the extent of forgetting is impacted by the fine-tuning objective but not the size of the dataset. We found that ranking models forget the least and retain more knowledge in their final layer. We release our code on github to repeat the experiments.
更多查看译文
关键词
bertnesia,knowledge,capture
AI 理解论文
溯源树
样例
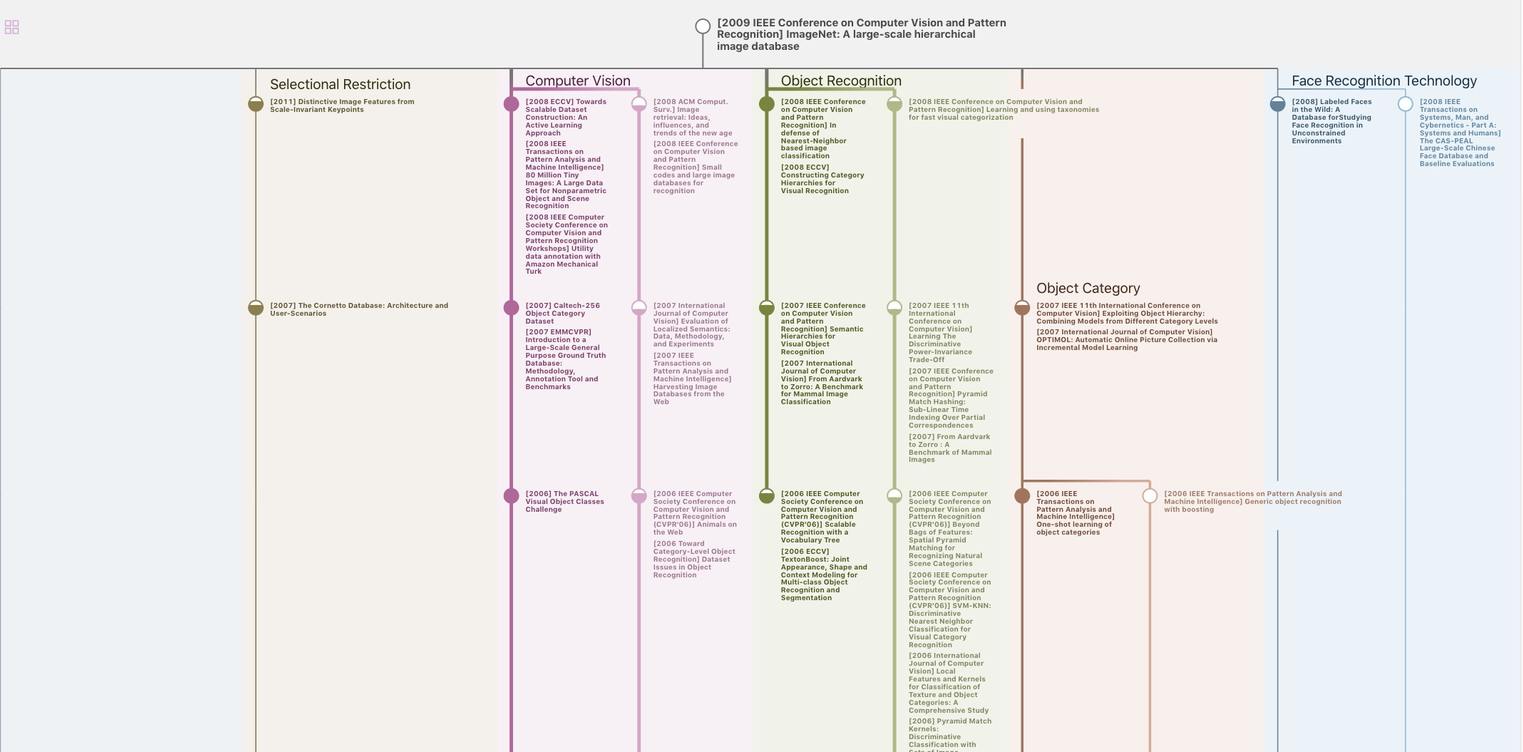
生成溯源树,研究论文发展脉络
Chat Paper
正在生成论文摘要