Safety Considerations in Deep Control Policies with Safety Barrier Certificates Under Uncertainty
IROS(2020)
摘要
Recent advances in Deep Machine Learning have shown promise in solving complex perception and control loops via methods such as reinforcement and imitation learning. However, guaranteeing safety for such learned deep policies has been a challenge due to issues such as partial observability and difficulties in characterizing the behavior of the neural networks. While a lot of emphasis in safe learning has been placed during training, it is non-trivial to guarantee safety at deployment or test time. This paper extends how under mild assumptions, Safety Barrier Certificates can be used to guarantee safety with deep control policies despite uncertainty arising due to perception and other latent variables. Specifically for scenarios where the dynamics are smooth and uncertainty has a finite support, the proposed framework wraps around an existing deep control policy and generates safe actions by dynamically …
更多查看译文
关键词
Safety considerations,deep control policies,Safety Barrier Certificates,Deep Machine Learning,complex perception,control loops,imitation learning,guaranteeing safety,learned deep policies,partial observability,safe learning,existing deep control policy,safe actions,control barrier functions,control actions,original actions,safety constraint
AI 理解论文
溯源树
样例
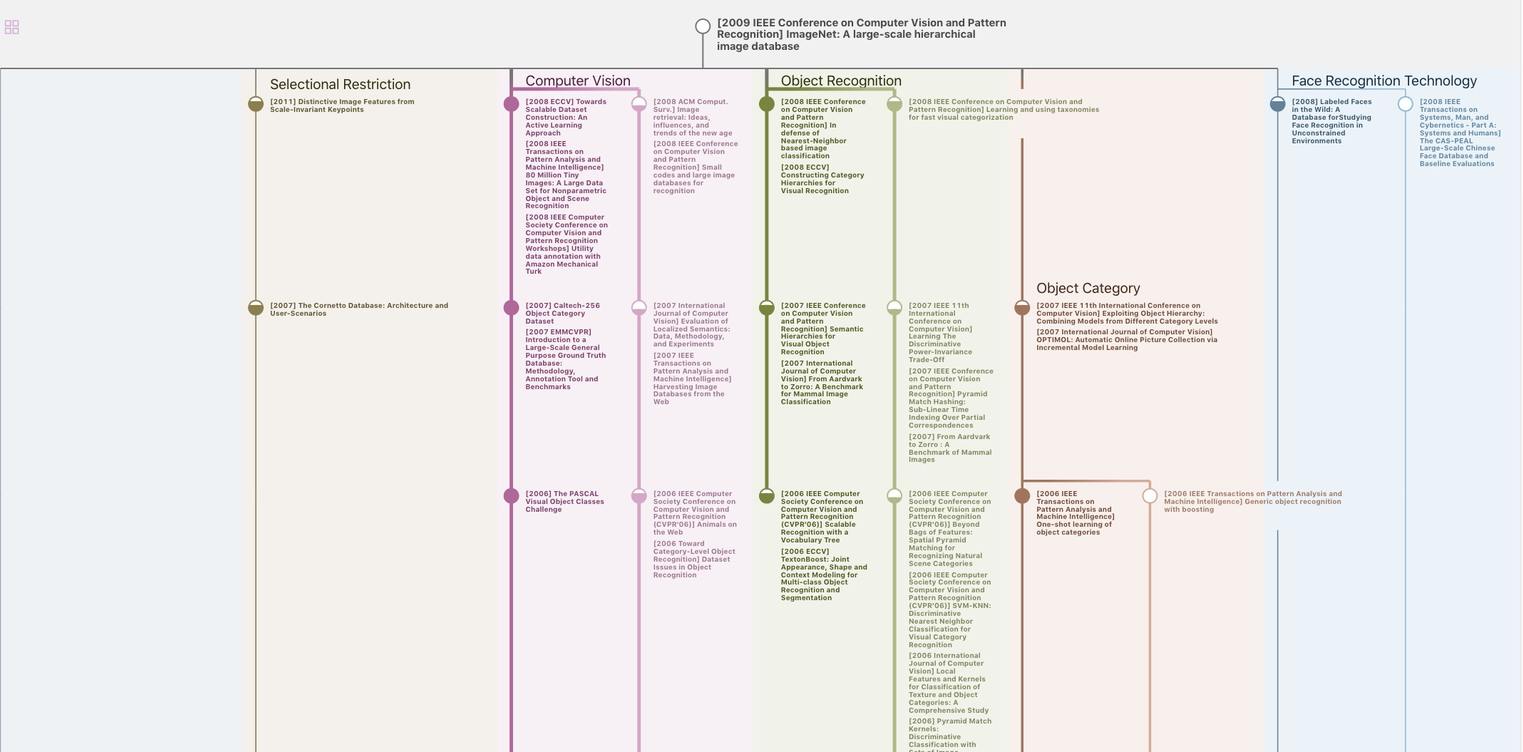
生成溯源树,研究论文发展脉络
Chat Paper
正在生成论文摘要