Regularly varying representation for sentence embedding
user-5f1696ff4c775ed682f5929f(2019)
摘要
The dominant approaches to sentence representation in natural language rely on learning embeddings on massive corpuses. The obtained embeddings have desirable properties such as compositionality and distance preservation (sentences with similar meanings have similar representations). In this paper, we develop a novel method for learning an embedding enjoying a dilation invariance property. We propose two algorithms: Orthrus, a classification algorithm, constrains the distribution of the embedded variable to be regularly varying, ie multivariate heavy-tail. and uses Extreme Value Theory (EVT) to tackle the classification task on two separate regions: the tail and the bulk. Hydra, a text generation algorithm for dataset augmentation, leverages the invariance property of the embedding learnt by Orthrus to generate coherent sentences with controllable attribute, eg positive or negative sentiment. Numerical experiments on synthetic and real text data demonstrate the relevance of the proposed framework.
更多查看译文
AI 理解论文
溯源树
样例
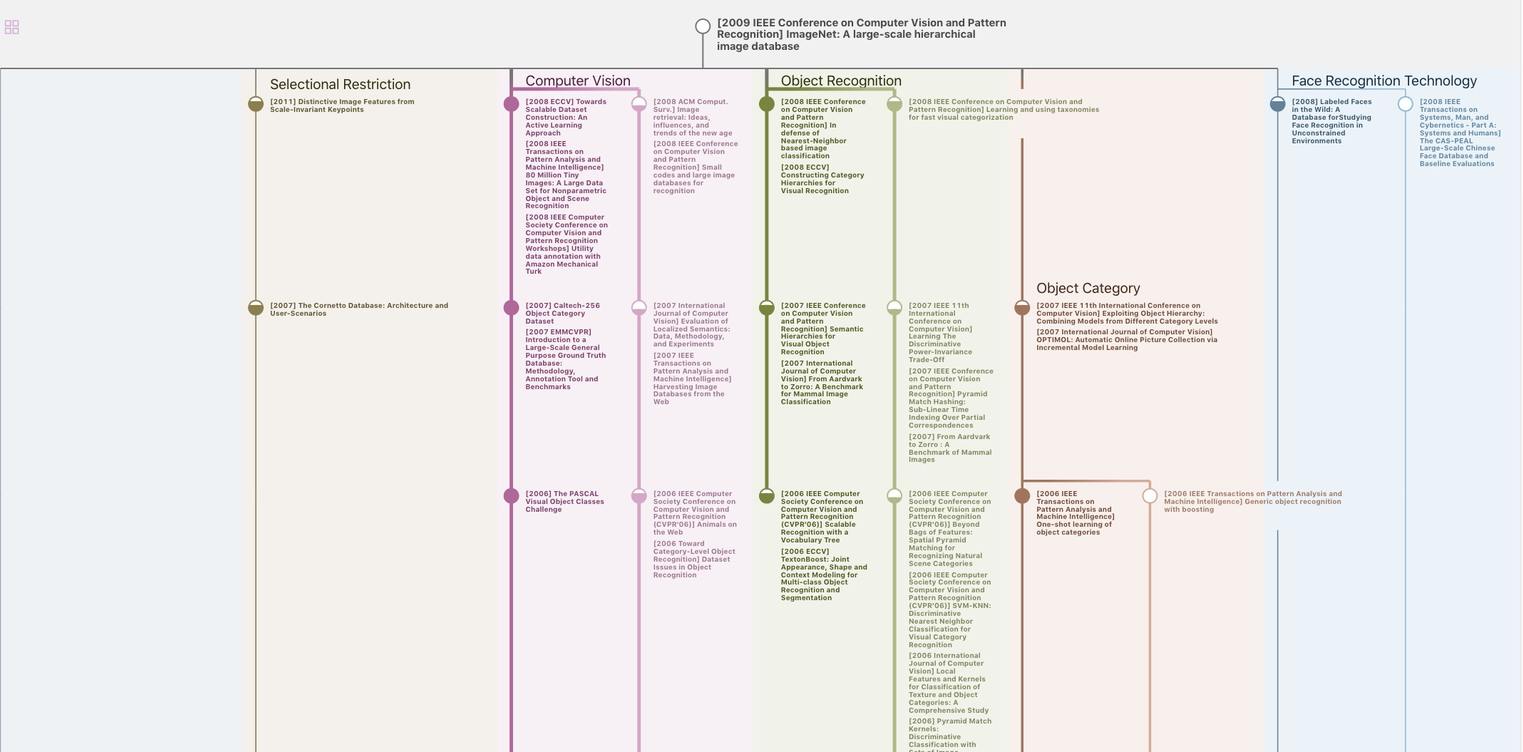
生成溯源树,研究论文发展脉络
Chat Paper
正在生成论文摘要