Incorporating User Feedback into Sequence to Sequence Model Training
CIKM '20: The 29th ACM International Conference on Information and Knowledge Management Virtual Event Ireland October, 2020(2020)
摘要
As the largest professional network, LinkedIn hosts millions of user profiles and job postings. Users effectively find what they need by entering search queries. However, finding what they are looking for can be a challenge, especially if they are unfamiliar with specific keywords from their industry. Query Suggestion is a popular feature where a search engine can suggest alternate, related queries. At LinkedIn, we have productionized a deep learning Seq2Seq model to transform an input query into several alternatives. This model is trained by examining search history directly typed by users. Once online, we can determine whether or not users clicked on suggested queries. This new feedback data indicates which suggestions caught the user's attention. In this work, we propose training a model with both the search history and user feedback datasets. We examine several ways to incorporate feedback without any architectural change, including adding a novel pairwise ranking loss term during training. The proposed new training technique produces the best combined score out of several alternatives in offline metrics. Deployed in the LinkedIn search engine, it significantly outperforms the control model with respect to key business metrics.
更多查看译文
关键词
Ranking models, Deep Neural Networks, Sequence to sequence, Query Suggestion, Machine Translation
AI 理解论文
溯源树
样例
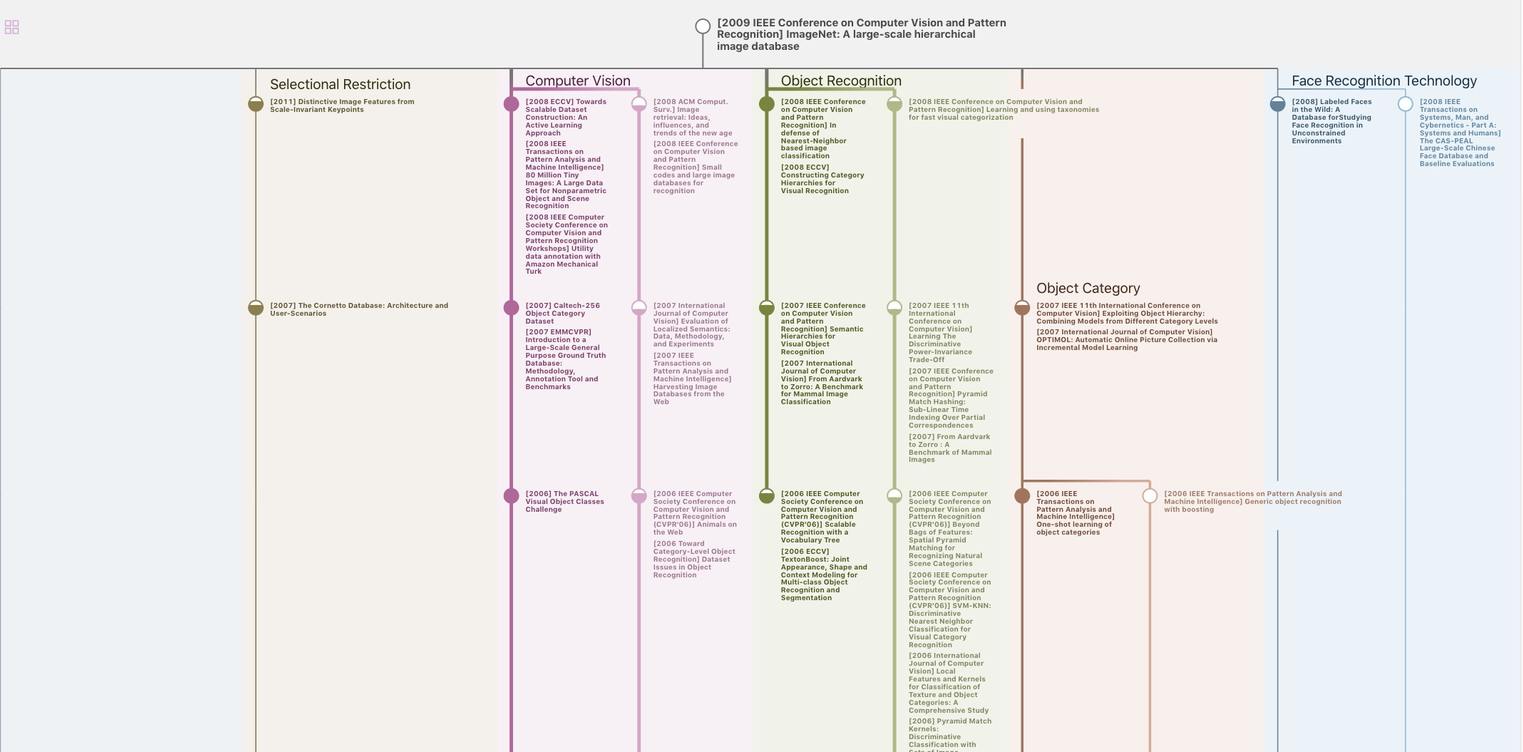
生成溯源树,研究论文发展脉络
Chat Paper
正在生成论文摘要