MICK: A Meta-Learning Framework for Few-shot Relation Classification with Small Training Data
CIKM '20: The 29th ACM International Conference on Information and Knowledge Management Virtual Event Ireland October, 2020(2020)
摘要
Few-shot relation classification seeks to classify incoming query instances after meeting only few support instances. This ability is gained by training with large amount of in-domain annotated data. In this paper, we tackle an even harder problem by further limiting the amount of data available at training time. We propose a few-shot learning framework for relation classification, which is particularly powerful when the training data is very small. In this framework, models not only strive to classify query instances, but also seek underlying knowledge about the support instances to obtain better instance representations. The framework also includes a method for aggregating cross-domain knowledge into models by open-source task enrichment. Additionally, we construct a brand new dataset: the TinyRel-CM dataset, a few-shot relation classification dataset in health domain with purposely small training data and challenging relation classes. Experimental results demonstrate that our framework brings performance gains for most underlying classification models, outperforms the state-of-the-art results given small training data, and achieves competitive results with sufficiently large training data.
更多查看译文
关键词
few-shot learning, meta-learning, small training data, relation classification
AI 理解论文
溯源树
样例
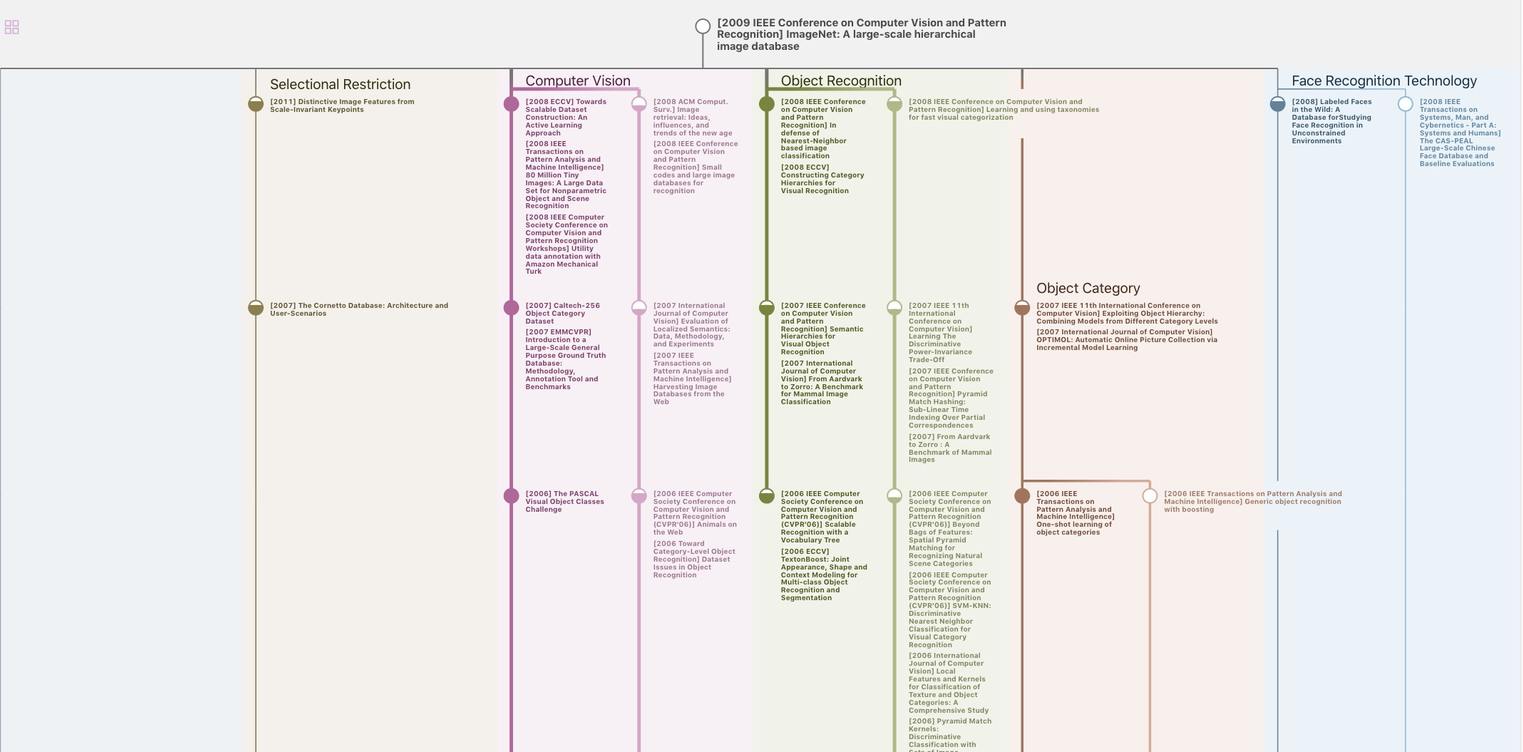
生成溯源树,研究论文发展脉络
Chat Paper
正在生成论文摘要