Recommending Inferior Results: A General and Feature-Free Model for Spam Detection
CIKM '20: The 29th ACM International Conference on Information and Knowledge Management Virtual Event Ireland October, 2020(2020)
摘要
Spam activities on multifarious online platforms, such as the opinion spam and fake following relationships have been extensively studied for years. Existing works separately employ hand-crafted features --- mainly extracted from user behavior, text information, and relational network, to detect the specific spamming phenomenon on a certain kind of online platform. Although these attempts have made some headway, rapidly emerging spamming categories and frequently changing cheating strategies lead detection models to be subject to circumscribed usability and fragile effectiveness.
This paper is the first attempt to develop a general and feature-free fraud detection model, tackling the longstanding and thorny challenges in spam detection area. To achieve this, we first transform diverse relational networks that are contaminated by fraudsters into the unified matrix form. We then deal with the spam problem from a fresh perspective inspired by the pairwise learning thought in the area of recommender system. By comparing pairwise ranking relations of all the entities in the unified matrix, a new pairwise loss objective function is formulated to identify instances that occupy higher rankings as inferior (spamming) results. To further boost detection performance, we incorporate the pairwise ranking detection method and the widely used structure-based algorithm into an integrated framework. Experiments on real-word datasets of different Web applications show significant improvements of our proposed framework over competitive methods.
更多查看译文
关键词
spam detection, pairwise ranking, label propagation
AI 理解论文
溯源树
样例
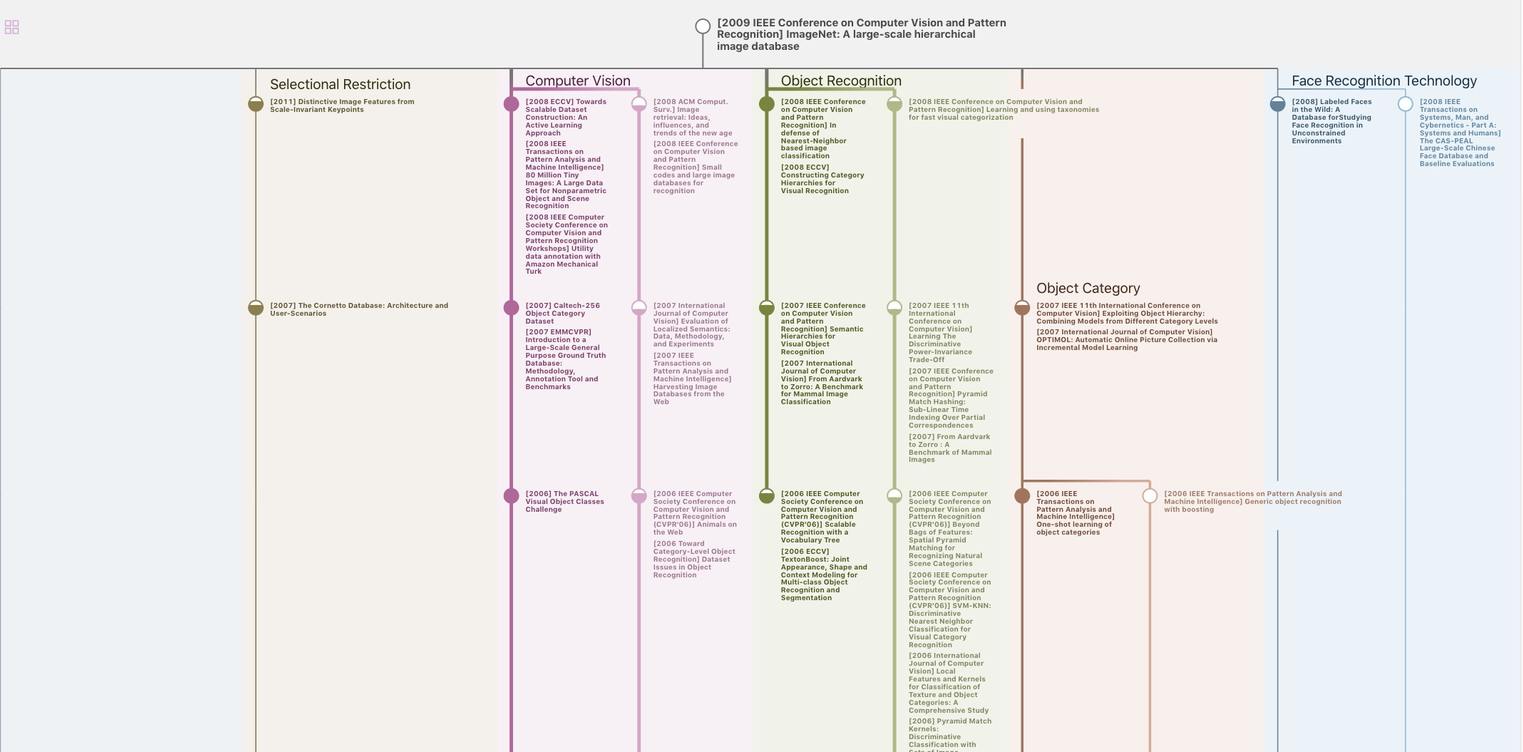
生成溯源树,研究论文发展脉络
Chat Paper
正在生成论文摘要