Probabilistic Inference with Generating Functions for Animal Populations
Artificial Intelligence and Conservation(2019)
摘要
Estimating the size and demographic parameters of animal populations is key to effective conservation. This is commonly done by using counts of animals made by human observers to estimate the parameters of a population model. When some variables from the population model are not directly observed–for example, the number of animals that are not detected by the observer, or the number of animals that leave a habitat patch between two consecutive surveys–an inference algorithm is required to reason about hidden events while fitting the model.Probabilistic inference is a challenging computational problem, and a great deal of AI research over the last 20 years has been devoted to developing efficient and general probabilistic inference algorithms. Despite great advances, models can still be “hard” for several reasons. One reason is model size and complexity, for example, as measured by the number of variables and the number, structure, and type of functional relationships among variables. There is a natural trend toward more complex models in ecology as we collect large and diverse datasets through efforts such as citizen science [Sullivan et al., 2009]. Developing efficient probabilistic inference algorithms to reason about complex ecological models from growing data resources is an important research direction.
更多查看译文
AI 理解论文
溯源树
样例
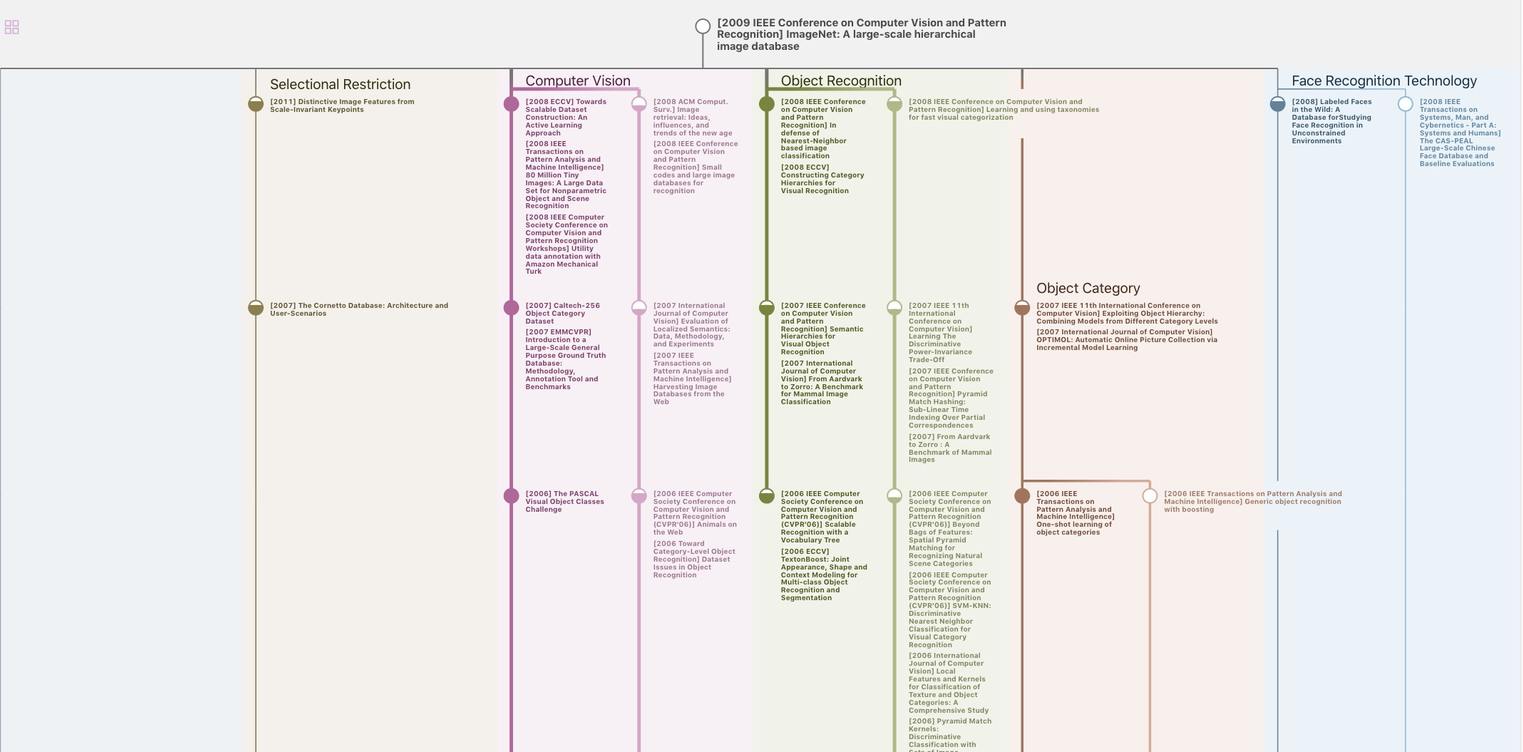
生成溯源树,研究论文发展脉络
Chat Paper
正在生成论文摘要