Simple and Effective: Spatial Rescaling for Person Reidentification
IEEE Transactions on Neural Networks and Learning Systems(2022)
摘要
Global average pooling (GAP) allows convolutional neural networks (CNNs) to localize discriminative information for recognition using only image-level labels. While GAP helps CNNs to attend to the most discriminative features of an object, e.g., head of a bird or one man’s bag, it may suffer if that information is missing due to camera viewpoint changes and intraclass variations in some tasks. To circumvent this issue, we propose one new module to help CNNs to see more, namely, Spatial Rescaling (SpaRs) layer. It introduces spatial relations among the feature map activations back to the model, guiding the model to focus on a broad area in the feature map. With simple implementation, it can be inserted into CNNs of various architectures directly. SpaRs layer consistently improves the performance over the reidentification (re-ID) models. Besides, the new module based on different normalization methods also demonstrates the superiority of fine-grained and general image classification benchmarks. The visualization method shows the changes in activated regions when equipped with the SpaRs layer for better understanding. Our code is publicly available at
https://github.com/HRanWang/Spatial-Re-Scaling
.
更多查看译文
关键词
Consistent improvement,global average pooling (GAP),person reidentification (re-ID),spatial relation,spatial rescaling (SpaRs)
AI 理解论文
溯源树
样例
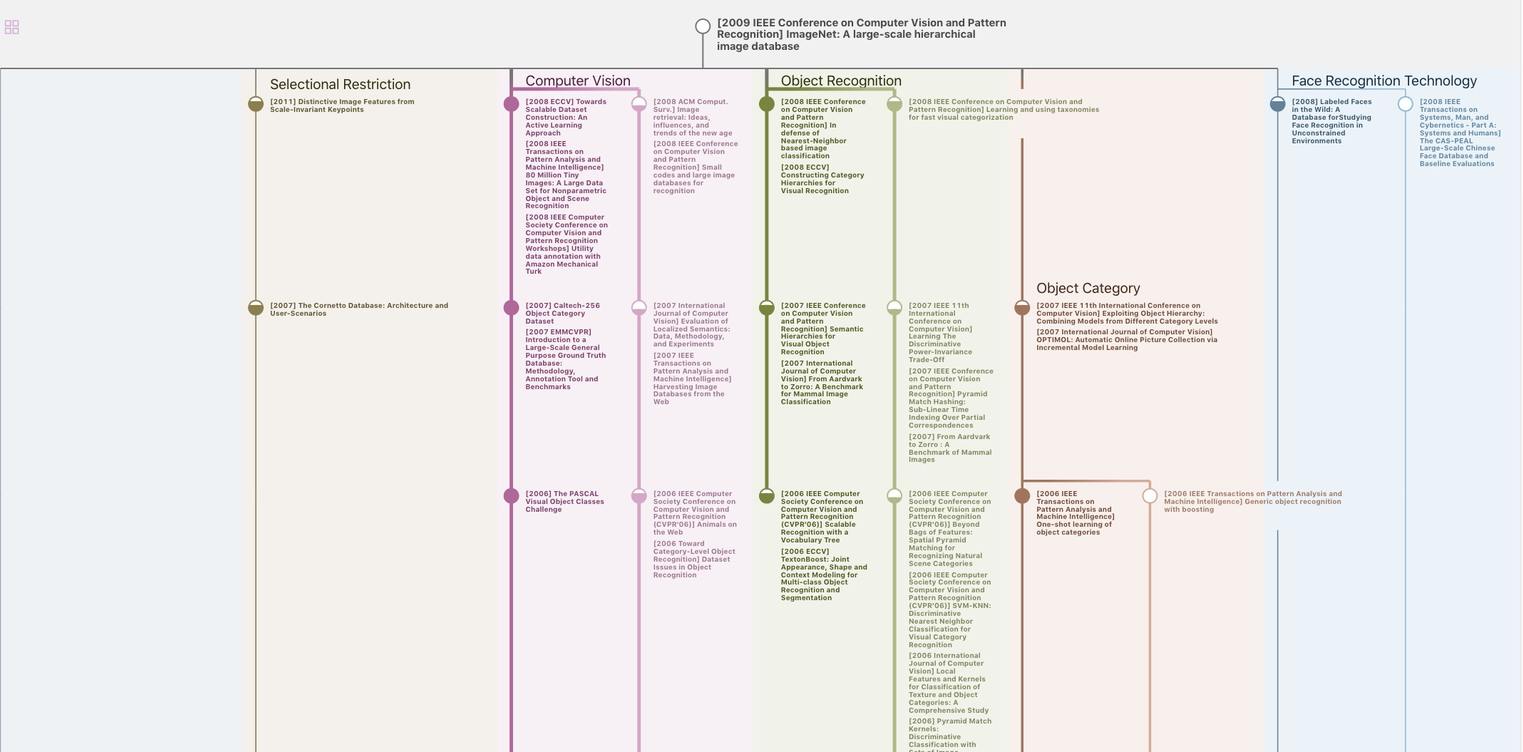
生成溯源树,研究论文发展脉络
Chat Paper
正在生成论文摘要