Comparison of Interactive Knowledge Base Spelling Correction Models for Low-Resource Languages
arxiv(2020)
摘要
Spelling normalization for low resource languages is a challenging task because the patterns are hard to predict and large corpora are usually required to collect enough examples. This work shows a comparison of a neural model and character language models with varying amounts on target language data. Our usage scenario is interactive correction with nearly zero amounts of training examples, improving models as more data is collected, for example within a chat app. Such models are designed to be incrementally improved as feedback is given from users. In this work, we design a knowledge-base and prediction model embedded system for spelling correction in low-resource languages. Experimental results on multiple languages show that the model could become effective with a small amount of data. We perform experiments on both natural and synthetic data, as well as on data from two endangered languages (Ainu and Griko). Last, we built a prototype system that was used for a small case study on Hinglish, which further demonstrated the suitability of our approach in real world scenarios.
更多查看译文
关键词
interactive,low-resource
AI 理解论文
溯源树
样例
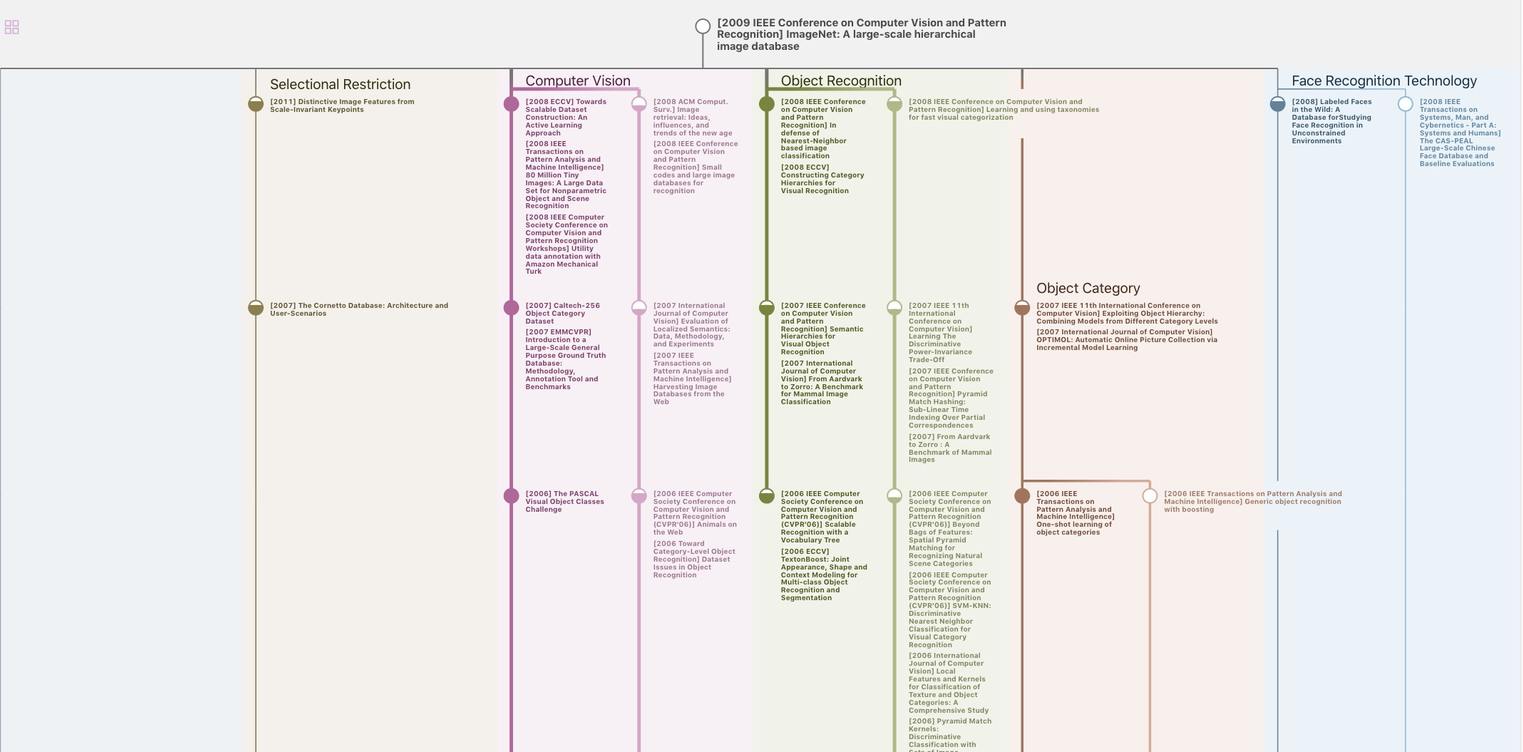
生成溯源树,研究论文发展脉络
Chat Paper
正在生成论文摘要