Twisted variational sequential monte carlo
Third workshop on Bayesian Deep Learning (NeurIPS)(2018)
摘要
Latent variable models have achieved wide-ranging success in generative modeling of images, speech, and video. Recent work has developed specialized objectives for fitting sequential latent variable models [1, 2, 3], but these methods are based on filtering sequential Monte Carlo (SMC), which can prevent them from successfully modeling data with long-term dependencies. In this work, we introduce twisted variational sequential Monte Carlo (TVSMC), a family of variational objectives that use learned tilting functions to approximate smoothing SMC without direct access to the smoothing distributions. We demonstrate that models trained with TVSMC can outperform models trained with VSMC, and theoretically, the TVSMC bound can become tight.
更多查看译文
AI 理解论文
溯源树
样例
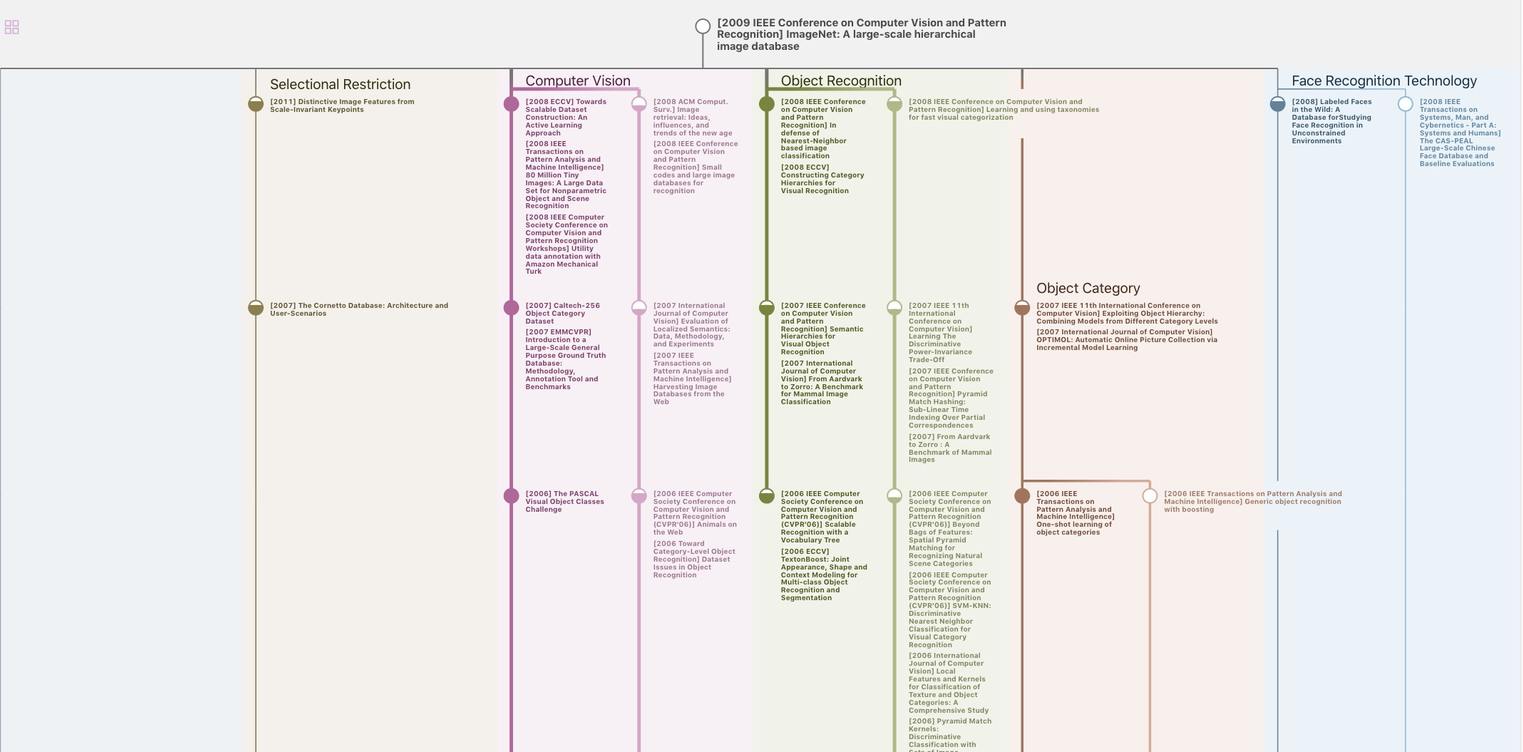
生成溯源树,研究论文发展脉络
Chat Paper
正在生成论文摘要