Towards Causal Understanding of Fake News Dissemination
arxiv(2020)
摘要
Recent years have witnessed remarkable progress made towards the computational detection of fake news. To mitigate its negative impact, however, we argue that a critical element is to understand why people spread fake news. Central to the question of "why" is the need to study the fake news sharing behavior. Deeply related to user characteristics and online activities, fake news sharing behavior is important to uncover the causal relationships between user attributes and the probability of this user to spread fake news. One obstacle in learning such user behavior is that most data is subject to selection bias, rendering partially observed fake news dissemination among users. To discover causal user attributes, we confront another obstacle of finding the confounders in fake news dissemination. Drawing on theories in causal inference, in this work, we first propose a principled approach to unbiased modelings of fake news dissemination under selection bias. We then consider the learned fake news sharing behavior as the measured confounder and further identify the user attributes that potentially cause users to spread fake news. We theoretically and empirically characterize the effectiveness of the proposed approach and find that it could be useful in protecting society from the perils of fake news.
更多查看译文
关键词
Fake news, User behavior, Causal inference, Social media
AI 理解论文
溯源树
样例
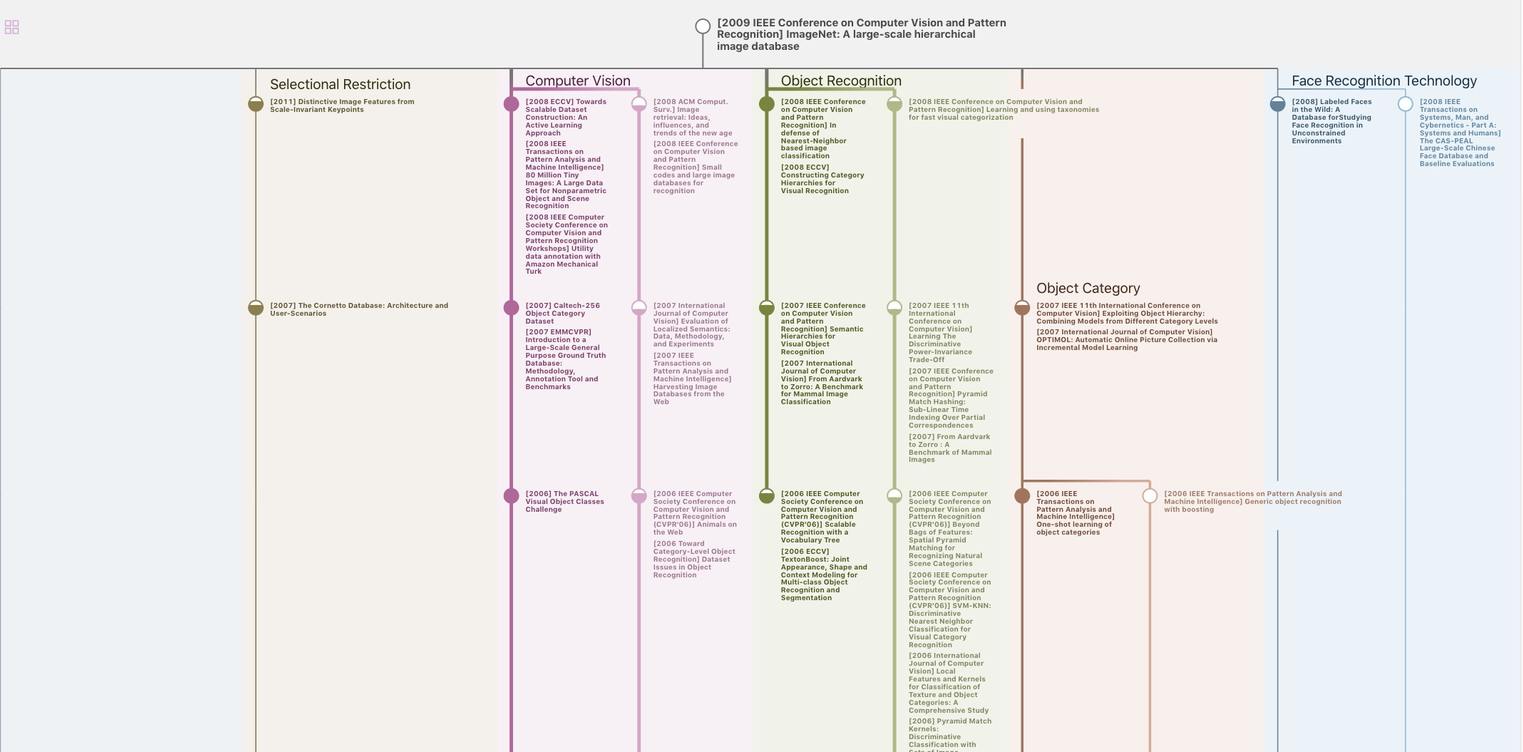
生成溯源树,研究论文发展脉络
Chat Paper
正在生成论文摘要