Deep Multi-Interest Network for Click-through Rate Prediction
CIKM '20: The 29th ACM International Conference on Information and Knowledge Management Virtual Event Ireland October, 2020(2020)
摘要
Click-through rate prediction plays an important role in many fields, such as recommender and advertising systems. It is one of the crucial parts to improve user experience and increase industry revenue. Recently, several deep learning-based models are successfully applied to this area. Some existing studies further model user representation based on user historical behavior sequence, in order to capture dynamic and evolving interests. We observe that users usually have multiple interests at a time and the latent dominant interest is expressed by the behavior. The switch of latent dominant interest results in the behavior changes. Thus, modeling and tracking latent multiple interests would be beneficial. In this paper, we propose a novel method named as Deep Multi-Interest Network (DMIN) which models user's latent multiple interests for click-through rate prediction task. Specifically, we design a Behavior Refiner Layer using multi-head self-attention to capture better user historical item representations. Then the Multi-Interest Extractor Layer is applied to extract multiple user interests. We evaluate our method on three real-world datasets. Experimental results show that the proposed DMIN outperforms various state-of-the-art baselines in terms of click-through rate prediction task.
更多查看译文
关键词
Recommender System, Click-through Rate prediction, Multi-interest
AI 理解论文
溯源树
样例
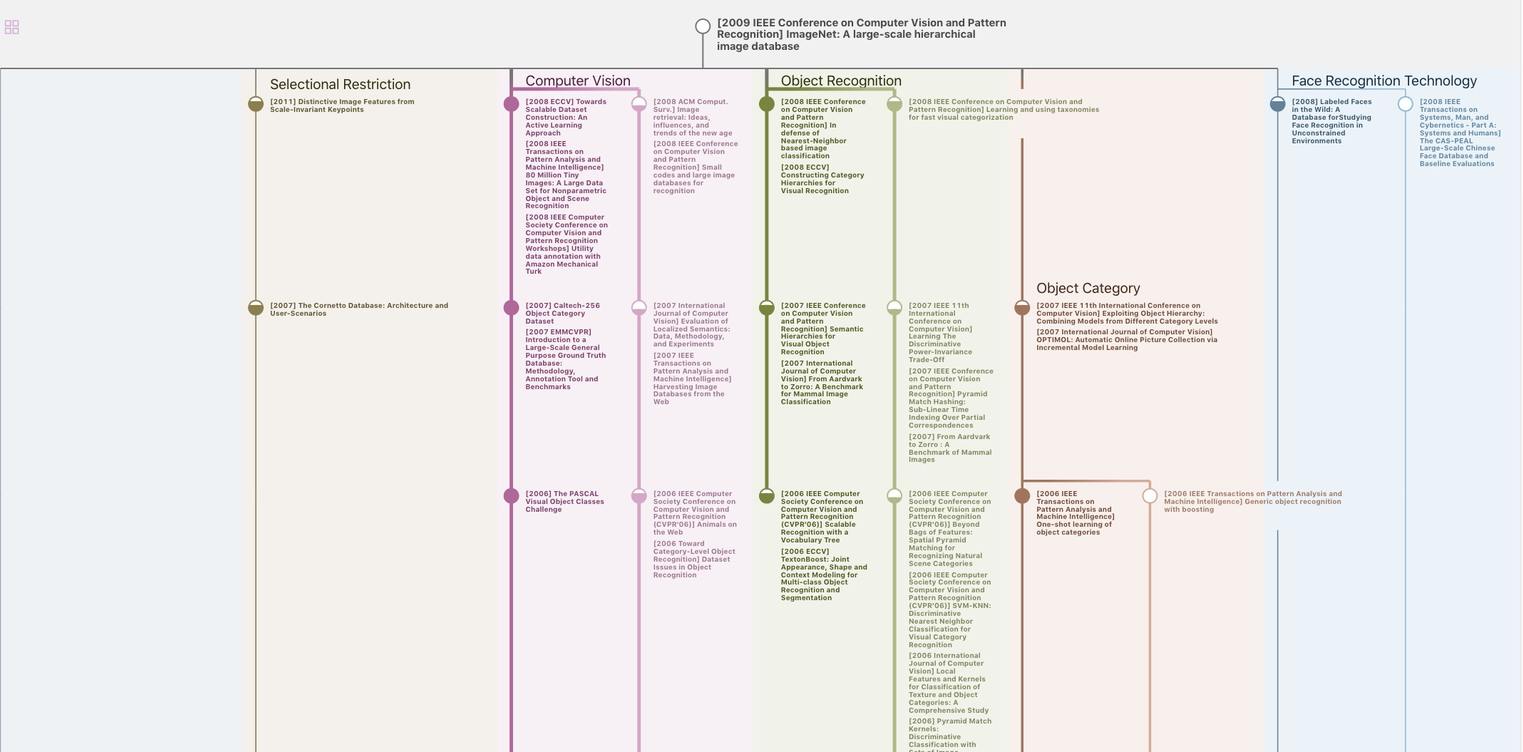
生成溯源树,研究论文发展脉络
Chat Paper
正在生成论文摘要