Regression via Kirszbraun Extension
arXiv preprint arXiv:1905.11930(2019)
摘要
We present a framework for performing regression between two Hilbert spaces. We accomplish this via Kirszbraun's extension theorem--apparently the first application of this technique to supervised learning--and analyze its statistical and computational aspects. We begin by formulating the correspondence problem in terms of quadratically constrained quadratic program (QCQP) regression. Then we describe a procedure for smoothing the training data, which amounts to regularizing hypothesis complexity via its Lipschitz constant. The Lipschitz constant is tuned via a Structural Risk Minimization (SRM) procedure, based on the covering-number risk bounds we derive. We apply our technique to learn a transformation between two robotic manipulators with different embodiments, and report promising results.
更多查看译文
AI 理解论文
溯源树
样例
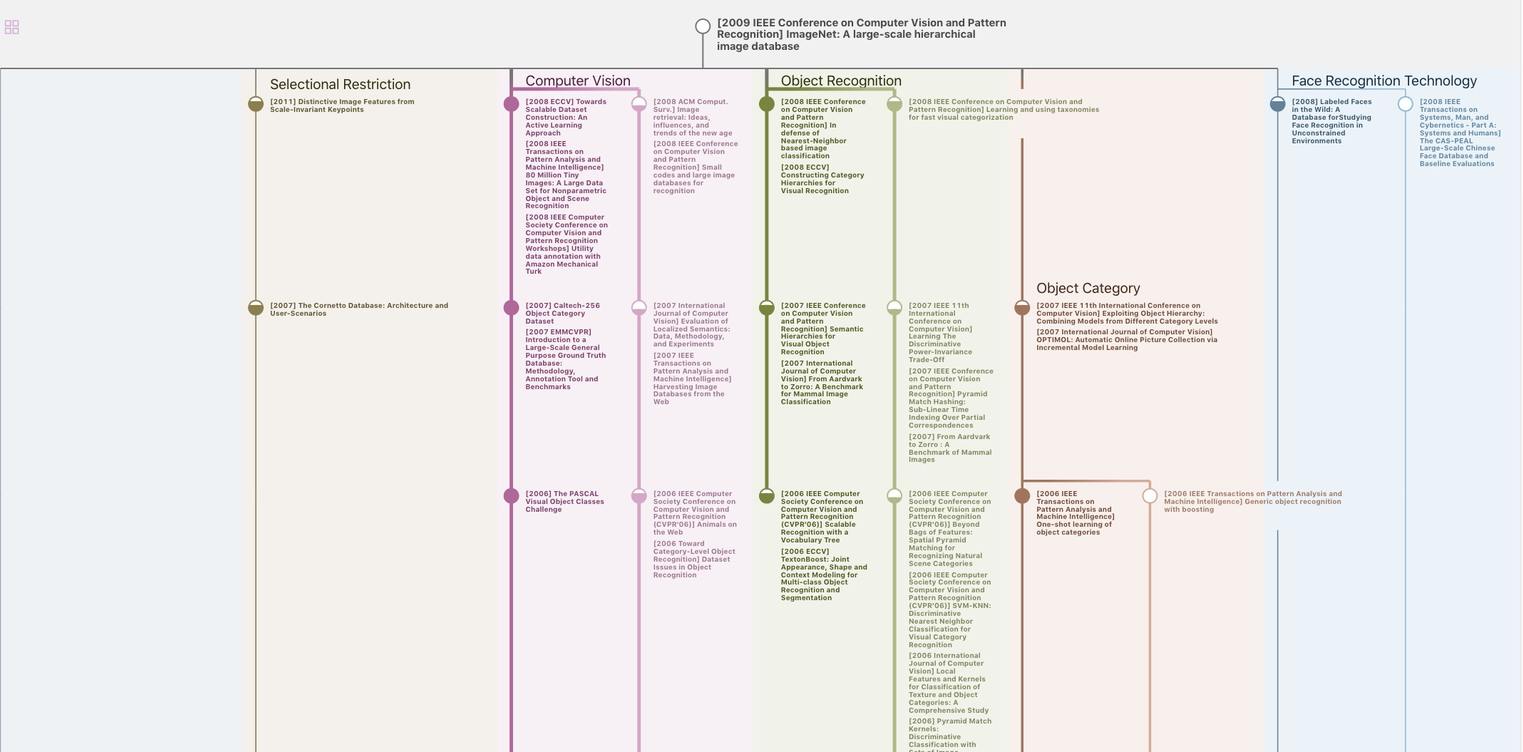
生成溯源树,研究论文发展脉络
Chat Paper
正在生成论文摘要