Probabilistic Programming for Deep Learning
user-5ebe3bbdd0b15254d6c50b2c(2020)
摘要
The nature of deep neural networks is compositional. Users can connect layers in creative ways, without having to worry about how to perform testing (forward propagation) or inference (gradientbased optimization, with back propagation and automatic differentiation).In this chapter, we design compositional representations for probabilistic programming. Probabilistic programming lets users specify generative probabilistic models as programs and then “compile” those models down into inference procedures. Probabilistic models are also compositional in nature, and much work has enabled rich probabilistic programs via compositions of random variables (Goodman et al., 2012; Ghahramani, 2015; Lake et al., 2016).
更多查看译文
AI 理解论文
溯源树
样例
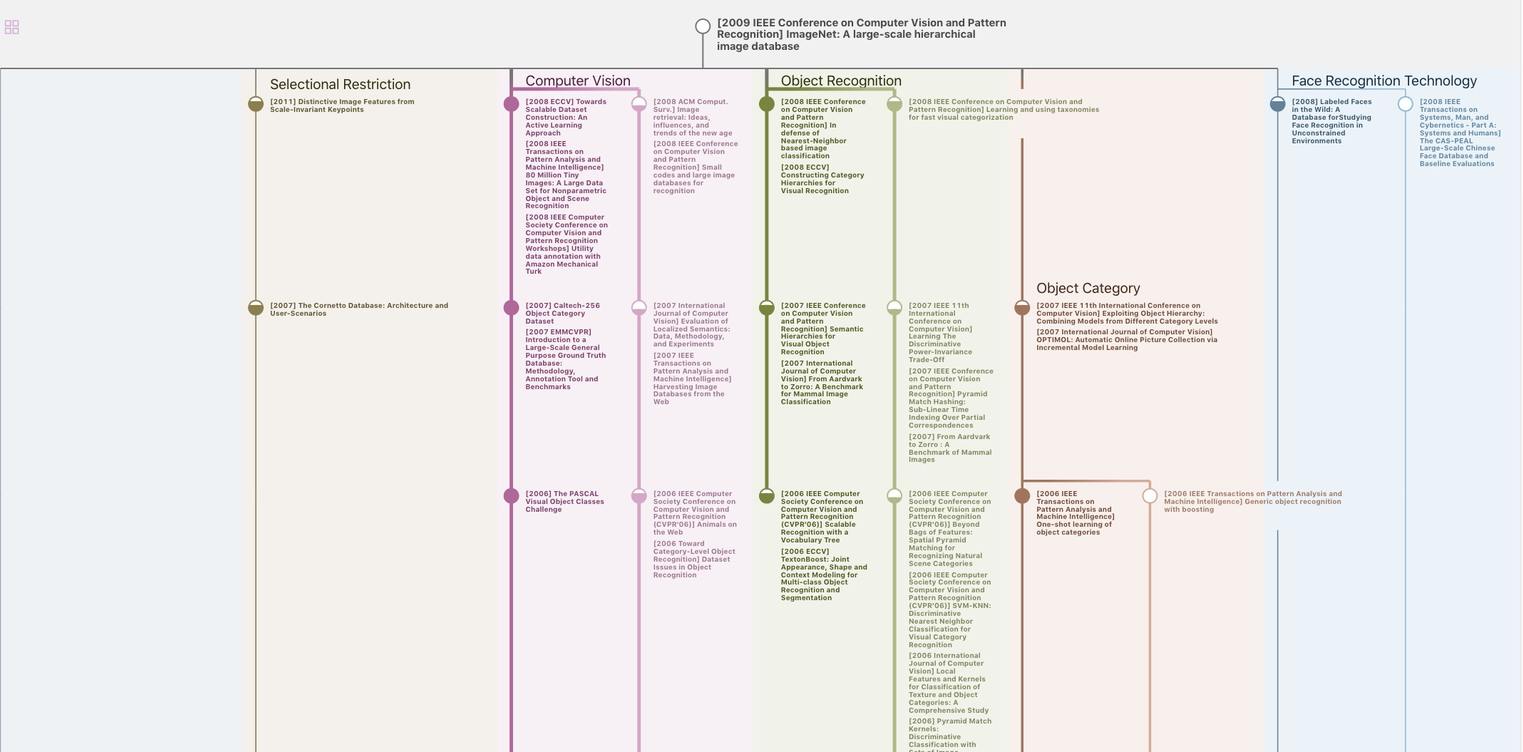
生成溯源树,研究论文发展脉络
Chat Paper
正在生成论文摘要