Flexible Approximate Inference via Stratified Normalizing Flows
UAI(2020)
摘要
A major obstacle to forming posterior distributions in machine learning is the difficulty of evaluating partition functions. Monte-Carlo approaches are unbiased, but can suffer from high variance. Variational methods are biased, but tend to have lower variance. We develop an approximate inference procedure that allows explicit control of the bias/variance tradeoff, interpolating between the sampling and the variational regime. We use a normalizing flow to map the integrand onto a uniform distribution. We then randomly sample regions from a partition of this uniform distribution and fit simpler, local variational approximations in the image of these regions through the flow. When a partition with only one region is used, we recover standard variational inference, and in the limit of an infinitely fine partition we recover Monte-Carlo sampling. We show experiments validating the effectiveness of our approach.
更多查看译文
AI 理解论文
溯源树
样例
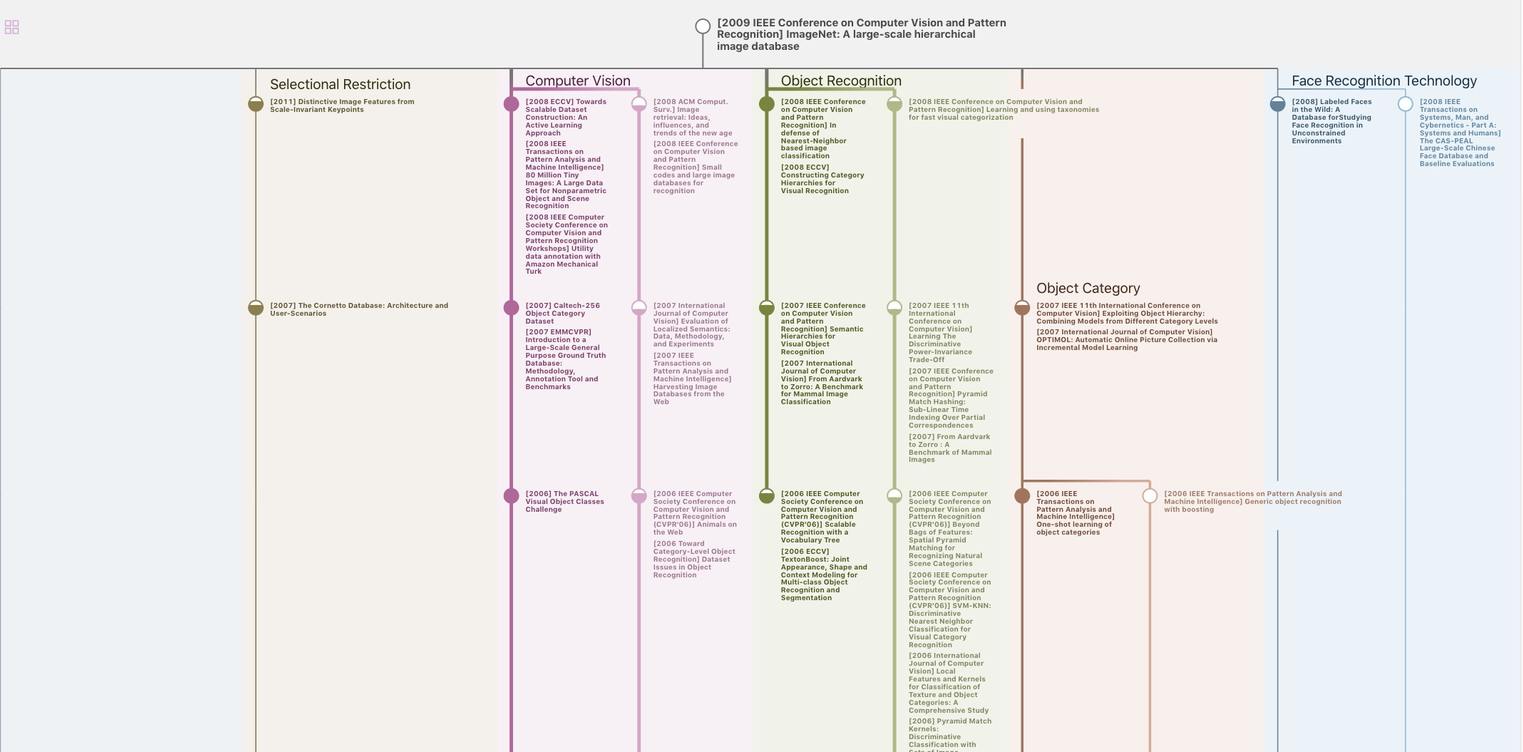
生成溯源树,研究论文发展脉络
Chat Paper
正在生成论文摘要