ONLINE TIME-VARYING TOPOLOGY IDENTIFICATION VIA PREDICTION-CORRECTION ALGORITHMS
2021 IEEE INTERNATIONAL CONFERENCE ON ACOUSTICS, SPEECH AND SIGNAL PROCESSING (ICASSP 2021)(2021)
摘要
Signal processing and machine learning algorithms for data supported over graphs, require the knowledge of the graph topology. Unless this information is given by the physics of the problem (e.g., water supply networks, power grids), the topology has to be learned from data. Topology identification is a challenging task, as the problem is often ill-posed, and becomes even harder when the graph structure is time-varying. In this paper, we address the problem of dynamic topology identification by building on recent results from time-varying optimization, devising a general-purpose online algorithm operating in non-stationary environments. Because of its iteration-constrained nature, the proposed approach exhibits an intrinsic temporal-regularization of the graph topology without explicitly enforcing it. As a case-study, we specialize our method to the Gaussian graphical model (GGM) problem and corroborate its performance.
更多查看译文
关键词
dynamic topology identification, online algorithm, graphical models, graph learning, time-varying optimization
AI 理解论文
溯源树
样例
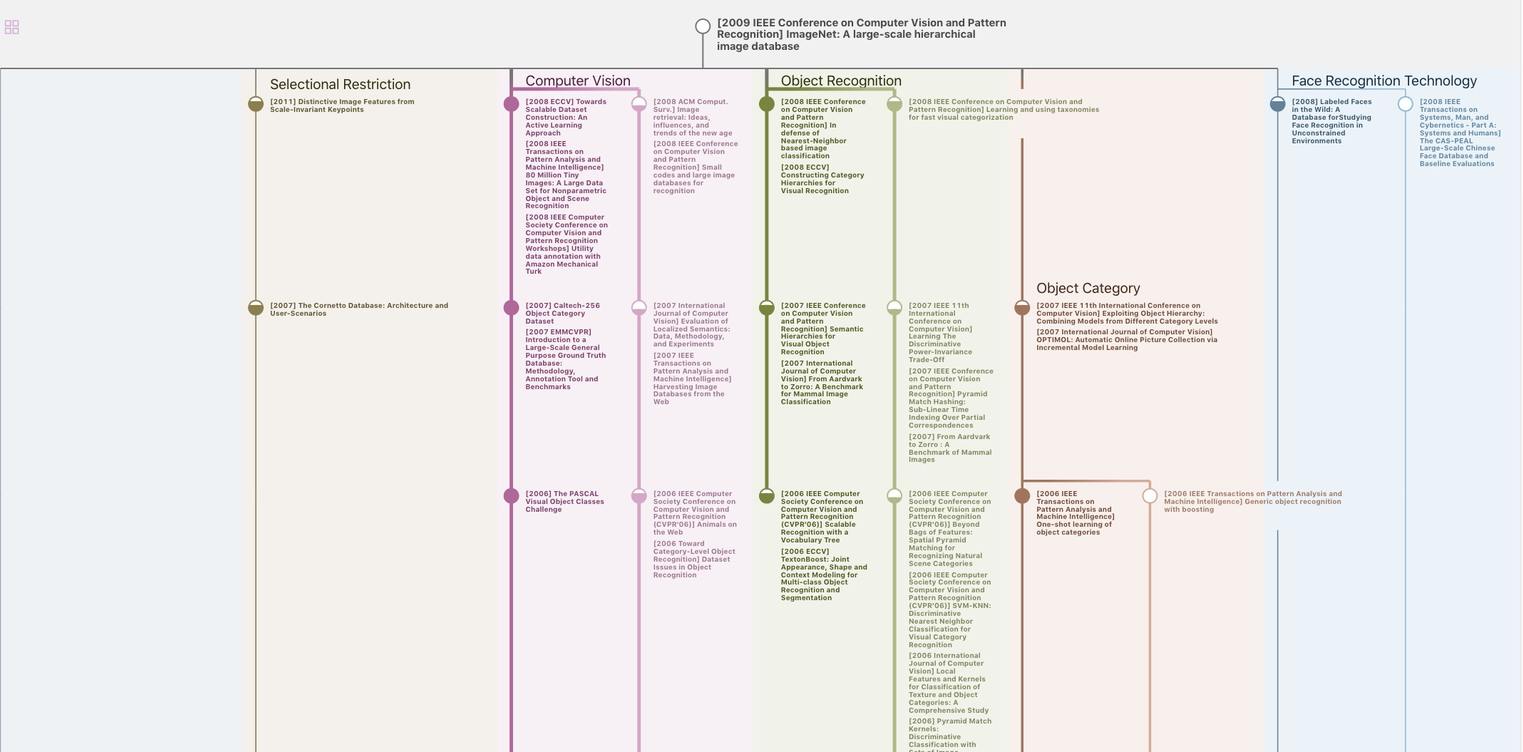
生成溯源树,研究论文发展脉络
Chat Paper
正在生成论文摘要