Enhanced Story Representation by ConceptNet for Predicting Story Endings
CIKM '20: The 29th ACM International Conference on Information and Knowledge Management Virtual Event Ireland October, 2020(2020)
摘要
Predicting endings for narrative stories is a grand challenge for machine commonsense reasoning. The task requires ac- curate representation of the story semantics and structured logic knowledge. Pre-trained language models, such as BERT, made progress recently in this task by exploiting spurious statistical patterns in the test dataset, instead of 'understanding' the stories per se. In this paper, we propose to improve the representation of stories by first simplifying the sentences to some key concepts and second modeling the latent relation- ship between the key ideas within the story. Such enhanced sentence representation, when used with pre-trained language models, makes substantial gains in prediction accuracy on the popular Story Cloze Test without utilizing the biased validation data.
更多查看译文
关键词
commonsense reasoning, story comprehension, commonsense knowledge
AI 理解论文
溯源树
样例
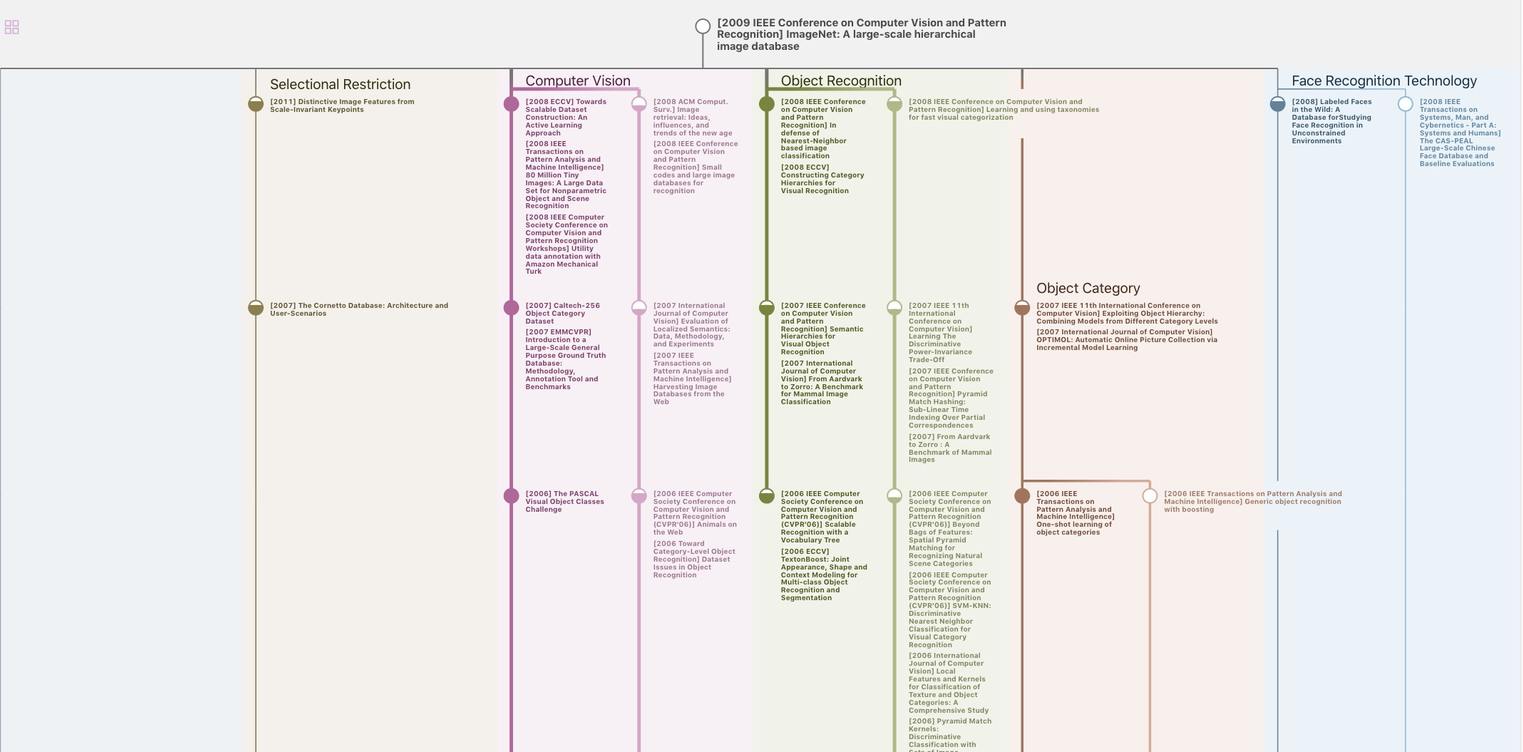
生成溯源树,研究论文发展脉络
Chat Paper
正在生成论文摘要