Predicting miRNA-disease associations using a hybrid feature representation in the heterogeneous network
BMC MEDICAL GENOMICS(2020)
摘要
Background Studies have found that miRNAs play an important role in many biological activities involved in human diseases. Revealing the associations between miRNA and disease by biological experiments is time-consuming and expensive. The computational approaches provide a new alternative. However, because of the limited knowledge of the associations between miRNAs and diseases, it is difficult to support the prediction model effectively. Methods In this work, we propose a model to predict miRNA-disease associations, MDAPCOM, in which protein information associated with miRNAs and diseases is introduced to build a global miRNA-protein-disease network. Subsequently, diffusion features and HeteSim features, extracted from the global network, are combined to train the prediction model by eXtreme Gradient Boosting (XGBoost). Results The MDAPCOM model achieves AUC of 0.991 based on 10-fold cross-validation, which is significantly better than that of other two state-of-the-art methods RWRMDA and PRINCE. Furthermore, the model performs well on three unbalanced data sets. Conclusions The results suggest that the information behind proteins associated with miRNAs and diseases is crucial to the prediction of the associations between miRNAs and diseases, and the hybrid feature representation in the heterogeneous network is very effective for improving predictive performance.
更多查看译文
关键词
miRNA-disease association,HeteSim measure,Diffusion feature,eXtreme gradient boosting
AI 理解论文
溯源树
样例
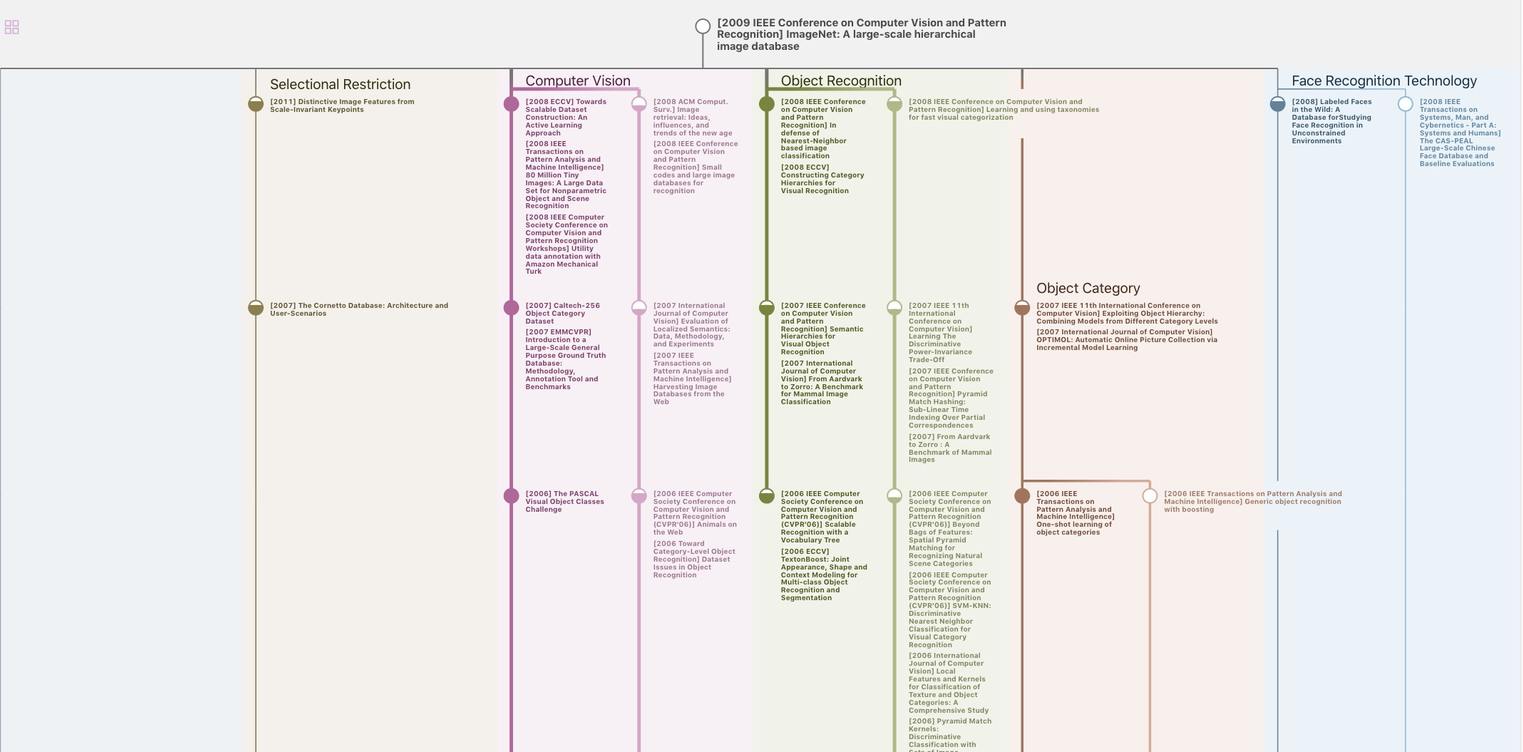
生成溯源树,研究论文发展脉络
Chat Paper
正在生成论文摘要