Ultra-low power on-chip learning of speech commands with phase-change memories
arxiv(2020)
摘要
Embedding artificial intelligence at the edge (edge-AI) is an elegant solution to tackle the power and latency issues in the rapidly expanding Internet of Things. As edge devices typically spend most of their time in sleep mode and only wake-up infrequently to collect and process sensor data, non-volatile in-memory computing (NVIMC) is a promising approach to design the next generation of edge-AI devices. Recently, we proposed an NVIMC-based neuromorphic accelerator using the phase change memories (PCMs), which we call as Raven. In this work, we demonstrate the ultra-low-power on-chip training and inference of speech commands using Raven. We showed that Raven can be trained on-chip with power consumption as low as 30~uW, which is suitable for edge applications. Furthermore, we showed that at iso-accuracies, Raven needs 70.36x and 269.23x less number of computations to be performed than a deep neural network (DNN) during inference and training, respectively. Owing to such low power and computational requirements, Raven provides a promising pathway towards ultra-low-power training and inference at the edge.
更多查看译文
关键词
speech commands,ultra-low,on-chip,phase-change
AI 理解论文
溯源树
样例
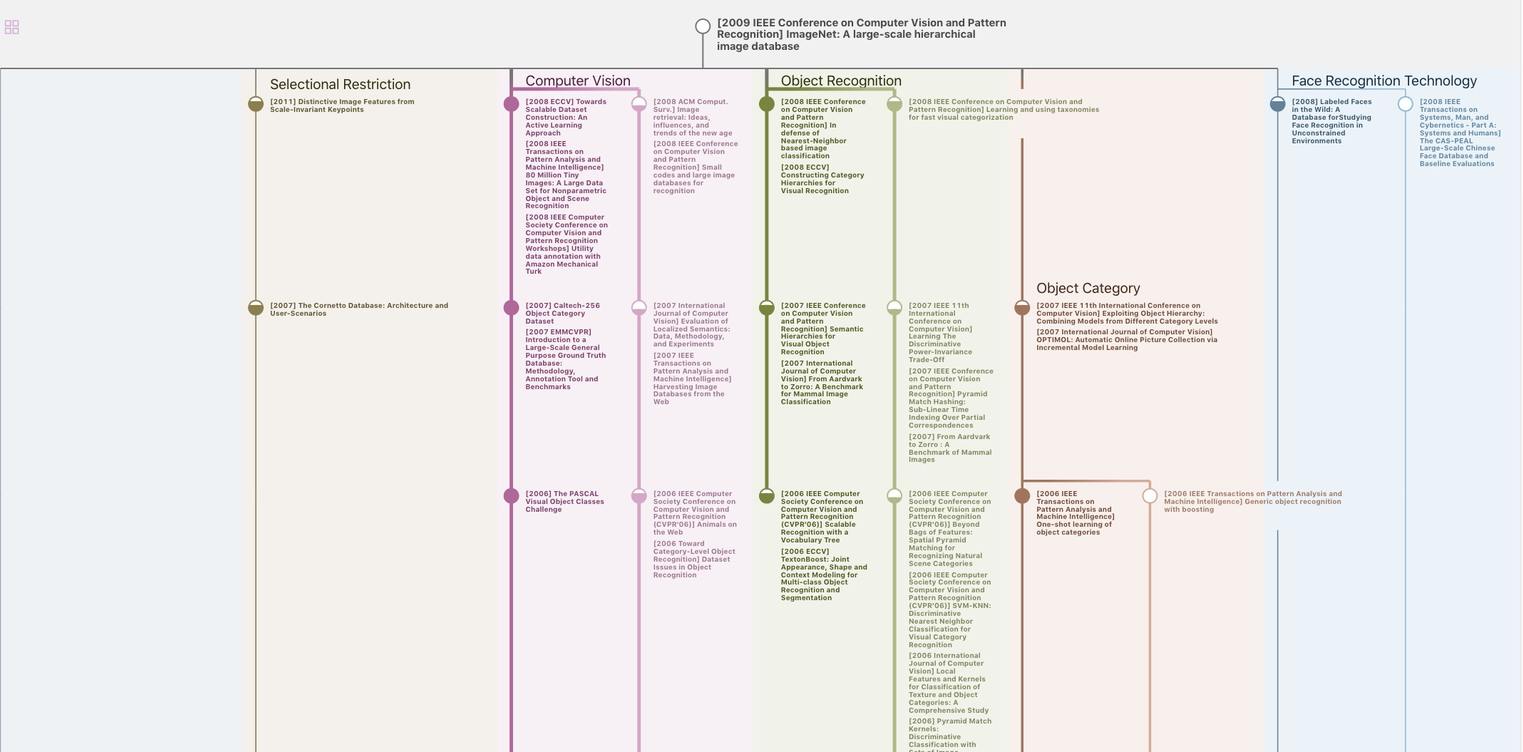
生成溯源树,研究论文发展脉络
Chat Paper
正在生成论文摘要